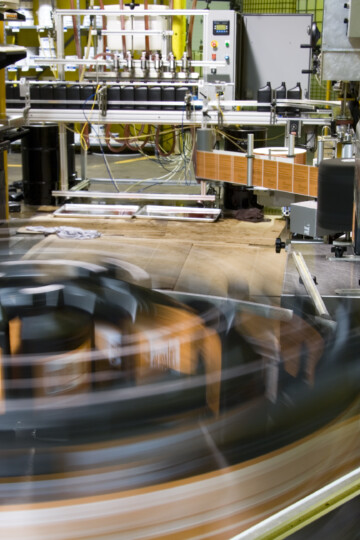
Production
Autonomous Systems on a Leash
A precisely defined operating environment is not only important for autonomous driving. The Operational Design Domain (ODD) also ensures safety for many other highly automated systems in rail transport, logistics and mobile robotics.