Read next
Safetronic 2023
On the Safety of A*
Assuming we knew how to properly assess the safety of an autonomous driving application, what would be the consequences? A preview of my presentation at the Safetronic conference (November 15th and 16th 2023).
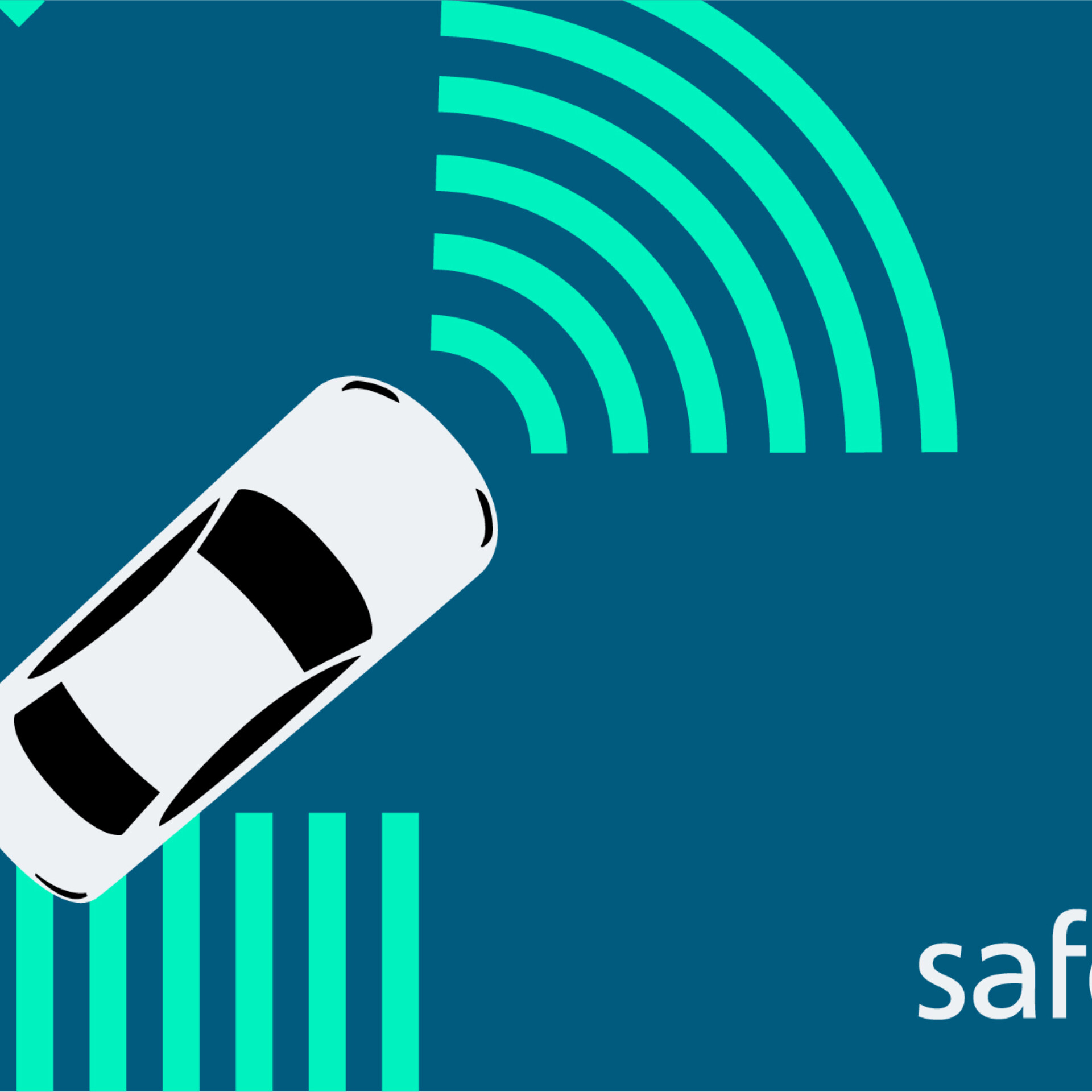
© FraunhoferIKS
A lot could be said and written about the safety of A*, but before it would make good sense to be more specific: the wildcard string A* stands for AD (Automated Driving) and AI (Artificial Intelligence), but we do not intend to cover AI in general. The recently quite popular generative AI applications for example are exempted because these are not relevant for AD. Instead, we will only consider AI applications required to implement AD safely, i.e., Supervised Learning using Artificial Neural Networks like Deep or Convolutional Neural Networks.
With this, we can now start to ask the right questions to develop a concept for analyzing the level of safety of AD implementations, such as:
- Why and for what do we need AI for AD?
- Why is it so difficult to prove the safety of AD or any behavioral property of AI?
- Are these similar difficulties just coincidence or are they related, or do they have a common cause?
All these questions will be addressed, with varying intensity. And slightly different answers than generally will be proposed. Take the capability to make ethical decisions for example. It is not relevant to justify the deployment of AI in AD applications because, against contrary belief, not even humans can make such decisions in concrete situations, and even more so, if they are directly affected – ethical decisions in real traffic situations are just a romantic illusion. More importantly, a sketch of a framework concept providing satisfying answers to these, and other questions, will be proposed. This framework concept builds on a strict separation of the design tasks (analysis and synthesis, to be more specific) and a new system classification based on system complexity and regularity (the presence of an inner structure like equivalence classes that would simplify the analysis).
From level 4 (SAE J3016) on it gets interesting …
With this background, we will be able to show –, not just argue or assume, like previously – that any implementation of a high-level automated driving system (AD L4+) requires at least – but probably also at most – a component implemented with artificial intelligence. We will further show, why this is the case and what the most important and inevitable drawback of this fact is. It will thus become obvious, why the safety ofAD is determined by (and not only somehow linked to) that of AI.
Safetronic 2023
You can meet Dr. Andreas Amoroso, Continental, and discuss his approach with him. Visit Safetronic, the International Conference on Holistic Safety for Road Vehicles. It will take place on November 15 and 16 2023 in Leinfelden-Echterdingen near Stuttgart.
Learn more about the program and the planned presentations.
It pays to be quick! Register by October 10 and benefit from our Early Bird offer!
We will then examine the safety of AI more closely and show, that because of the intrinsic complexity and irregularity of the task it is supposed to solve, the safety of corresponding implementations cannot be guaranteed anymore. Like, in principle, it used to be the case previously, when a “safety proof” could be provided. Instead, the safety of such implementations must be assessed statistically or probabilistically. Any such assessment must be an estimation of one or more parameters of a probability distribution supposed or assumed to correctly model the relevant aspects of the behavior on the system level, e.g. the probability of getting involved in an accident, the so-called “safety mileage” or more precisely, the mean distance between accidents, that obviously corresponds to the mean time between failures (MTBF) known from reliability engineering.
What does that mean for the “rest” of the
product life cycle?
This has consequences across the complete product lifecycle of any AD application. We will conclude by deriving and examining the most important ones for the underlying business cases, which have not been explicitly considered – though vaguely anticipated – previously. First, the obligatory validation of products before the final release, which used to be a snapshot validation of a prototypical incarnation of the product (type approval) at a single point in time, will have to be turned into a continuous validation or a supervised deployment, as it has been proposed for AI applications already covering all deployment phases of every concrete product until decommissioning. The intensity e.g., the amount of field operation data to be analyzed will be unprecedented. In other words, contemporary inspection and service obligations will be exceeded by far.
Secondly and consequently, the differentiation between producer and operator vanishes, and thus the operator’s role and responsibility will eventually not be associated with consumers anymore. Interestingly, this development is unconsciously anticipated whenever the recently popular SMSes (Safety Management Systems) are discussed. Lastly, a few further implications will be mentioned but not discussed in detail, e.g., the necessity to provide a regulatory framework suitable for automated driving (the German StVO might serve as an example). Likewise, an approach for insurance models well-suited for the task will be discussed which surprisingly will not have to differ much from current approaches.
Only based on these considerations, an appropriate assessment of the attractivity of available business case variants is possible. And for a sustained business with AD applications, it is also more than overdue. But answering the interesting question, of to what extent these conclusions may be generalized to AI applications outside the automotive industry, which are intensely discussed currently, is left as an exercise to the reader.