Read next
Railway transportation
Thank you for traveling with AI
Using artificial intelligence (AI) in the rail industry could potentially increase both efficiency and quality. At a workshop hosted by Fraunhofer IKS, experts from Deutsche Bahn, Siemens Mobility and the institute itself gathered to discuss the possible uses and challenges associated with AI in rail applications, as well as strategies for ensuring system safety.

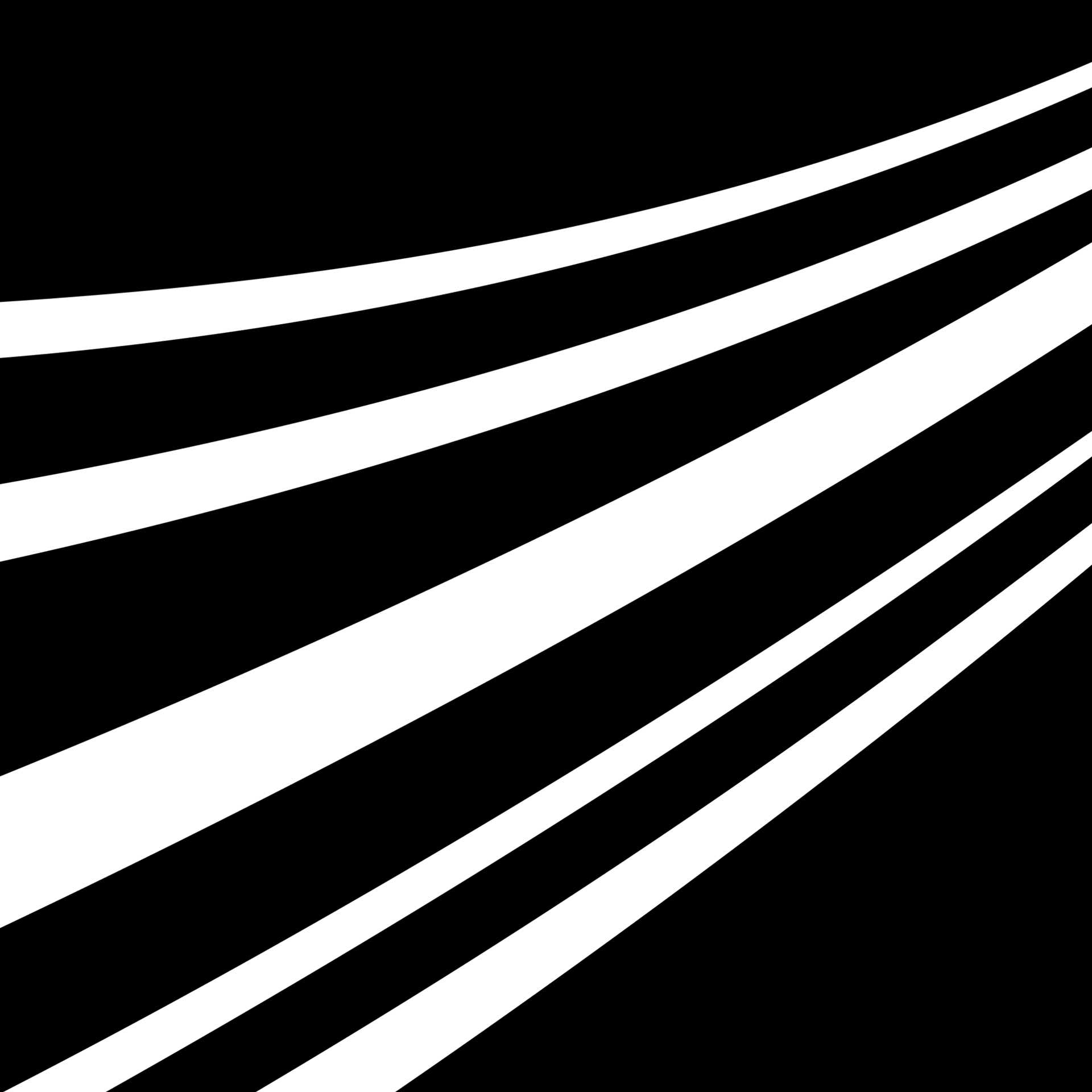
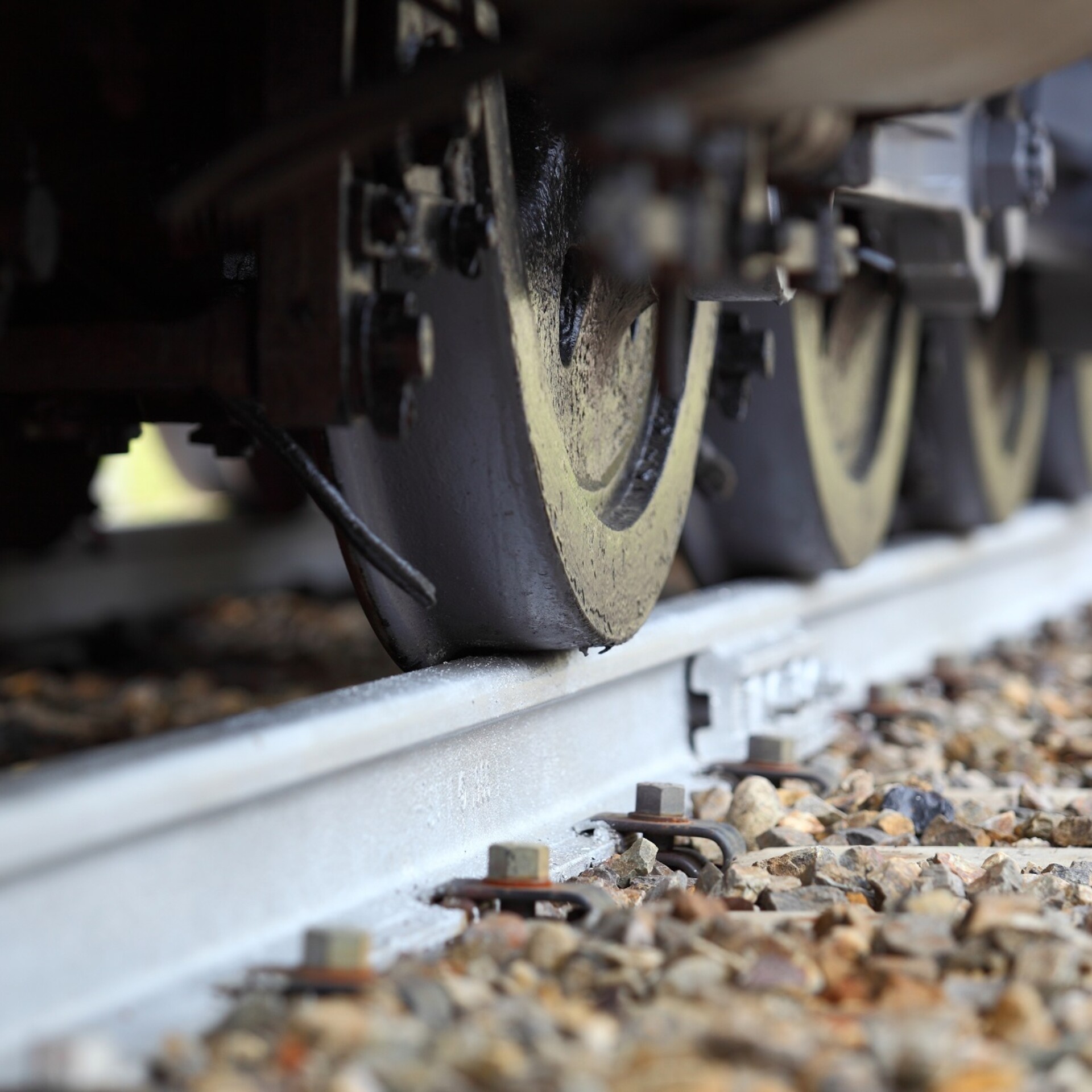
© iStock/75 tiks
Rail maintenance, network disruption, breakdowns, canceled trains — every rail passenger has seen messages like these on the platform information board. But what ultimately causes these delays? Could they have been avoided? Could the trains not run more regularly? These are the demands facing the complex public transportation systems that have to facilitate a smooth flow of traffic both within and between cities. Using artificial intelligence (AI) in these systems offers enormous potential for optimization across many fields of application, including driverless trains and predictive maintenance.
Artificial intelligence for railways — all aboard!
But why do we need AI in our railways? Dr. Claus Bahlmann, head of the R&D Department Artificial Intelligence at Siemens Mobility, offered some answers to this question at the workshop. Researchers have identified use cases for AI along the entire value chain, he explained; these have the potential to reduce the time and money spent on development and increase the quality of the service. Such use cases include project bidding processes supported by NLP (natural language processing), digital twins of infrastructures based on a mobile mapping system, predictive maintenance and driverless trains. “Some of these solutions are already in use today,” Dr. Bahlmann said, “but others require more development before they can be marketed as a product.”
There is, for example, a demonstrator of a GoA3/4 (i.e. driverless) driving system that includes automated environment perception technology, the ability to understand the surrounding environment, action planning and train control — it’s an entirely automated system with no human intervention whatsoever. But when the streetcar demonstrator appeared at Innotrans 2018, it was still equipped with a safety driver, who could intervene if the AI made an error in judgment. Using this example, Dr. Bahlmann pointed out the biggest challenge facing autonomous driving in rail transportation, namely the use of AI in safety-critical applications. “Say the AI makes the wrong call: if there isn’t a person that can intervene in the decision-making processes in the automated system, then the risk of damage is high,” warned Dr. Bahlmann. “At Siemens Mobility, we recognize the need to develop basic strategies for mitigating this risk so that we can fully realize the high potential of artificial intelligence in safety-critical applications.”
Outside of safety-critical applications, however, it’s an entirely different story: Artificial intelligence is now a commonplace feature in the production industry, where the safety standards associated with human participation are not as stringent. Examples include AI-driven internal processes, such as automated identification and ordering of spare parts, or digital assistance systems.
Intelligent maintenance of trains — how it works in practice
By way of illustration, Gregor Schmid, head of the E-Check project, and Patrick Goldschmidt, a specialist in AI, used a concrete example of a project at the company they both work for, Deutsche Bahn — the largest rail transport and infrastructure company in Central Europe. Their E-Check project is aimed at enabling automated maintenance of long-distance trains in the future.
As part of the project, an intercity express (ICE) train is driven slowly into the E-Check facility between service periods. There, a gate equipped with cameras captures 360-degree images of the train, which are then evaluated by an AI system. If the AI spots any abnormalities, on-site technicians will be sent all the relevant information via their mobile devices. They can use this information to either validate the fault and rectify it or log an inspection order in the central software system. There are also plans in place to use robots for automated water filling and removal processes.
Mr. Goldschmidt explained that the team opted for edge computing in order to meet the system’s quality requirements. When an ICE arrives, the system starts by identifying its product series, so as to ensure that it uses the correct machine learning model and metadata from the central software system. In addition, the models and data are constantly monitored by the central system in order to counteract model drift and concept drift.
Next, the E-check is conducted directly on site — this is done without connecting to the cloud, due to the large amounts of data involved. For Mr. Goldschmidt, the features that ensure the system’s quality pose a major challenge: There are no suitable standards to refer to as yet and no comparable systems aimed at implementing deep learning in train maintenance procedures. This means that researchers must rely on recent research findings and standards that are still at the drafting stage instead. The Fraunhofer Institute for Cognitive Systems IKS has a part to play here too, as it has been tasked with formulating the requirements for the AI system in order to ensure that the E-check is at least as safe as inspections conducted by humans.
From demonstrators to products — research challenges for ensuring AI safety
Dr. Chih-Hong Cheng, head of the Safety Assurance for AI department at Fraunhofer IKS, gave a first-hand account of his experience in applied research and his work in standardization committees. In his joint role as leader of a sub-project for validating AI systems within the safe.trAIn pilot project by the German Federal Ministry for Economic Affairs and Energy (BMWi) and deputy lead for the development of the ISO standard on safety and artificial intelligence (ISO PAS 8800), he was able to directly address the challenges mentioned by the previous speakers.
In particular, he emphasized the essential difference between working demonstrators and safe, marketable products. The safety requirements for the latter are far more extensive, as they must be able to respond to a variety of scenarios, some of which are unknown, in an uncontrollable environment. Other industries have already had experiences with this, which led Dr. Cheng to conclude: “We need to make note of the successes and failures of artificial intelligence in other domains and transfer this know-how to the railroad industry. That’s the only way to avoid wasting our efforts on reinventing the wheel.”
He divided the research required to develop AI systems for safety-critical applications into two overarching categories: “methodology” and “algorithms and tools.” In terms of methodology, Dr. Cheng sees the need to “marry” conventional security technology with data-driven system development, and then expand upon the resulting framework. This must be supported by new algorithms and tools. Key functionalities include enabling continuous development and safety improvements, as well as tool integrations to enable MLOps (machine learning operations). However, there is also a need to develop more advanced functionalities for supporting systematic testing, robust training and the capacity to work with specialized safety metrics.
Dr. Cheng then cited some real-world examples to demonstrate that the causes of problems in neural networks can be found across the entire ML development process. His goals are to curb these causes by preventing as many errors as possible from occurring and to formulate a safety case including usable metrics. He hopes to achieve this by systematically analyzing the function that is in development and dividing it up into problem classes with subgoals.
Dr. Cheng concluded his talk by providing some insight into the challenges of his current research, which is focused on finding the right approach for simulated data. This type of data is used to generate scenarios, but since it is not quite “real,” the possibilities for using it in safety-critical systems are limited. He went on to add that to ensure operational capability, we first require a systematic method. This might consist of comparing or combining real and synthetic data in artificial intelligence applications, for example.
But when will AI see extensive use in railway systems? This was the subject of a subsequent discussion between the speakers, which included representatives from rail vehicle manufacturers, suppliers, infrastructure operators and transportation companies. However, they were unable to reach a consensus, with estimates ranging from a few years to more than a decade. Despite this, there was one point they could all agree on: The train has already left the station. And the entire industry is already on board.