Read next
Future Mobility
What happens when AI gets confused
A sticker on a give way sign. Branches hanging in front of a stop sign. Graffiti on a speed limit sign. These are all completely normal sights on our roads, aren’t they? But things that wouldn’t generally be a problem for humans can really make life difficult for artificial intelligence.

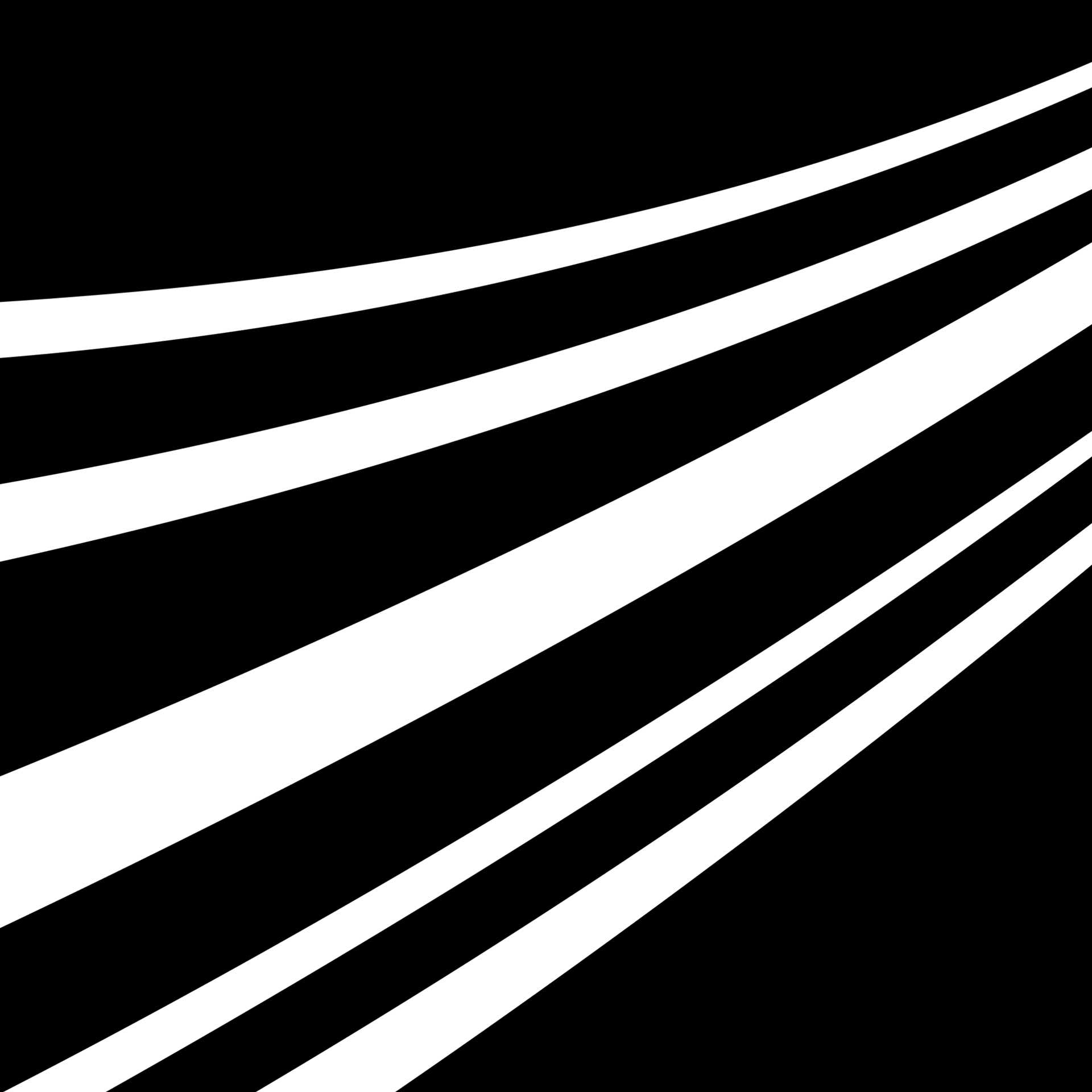
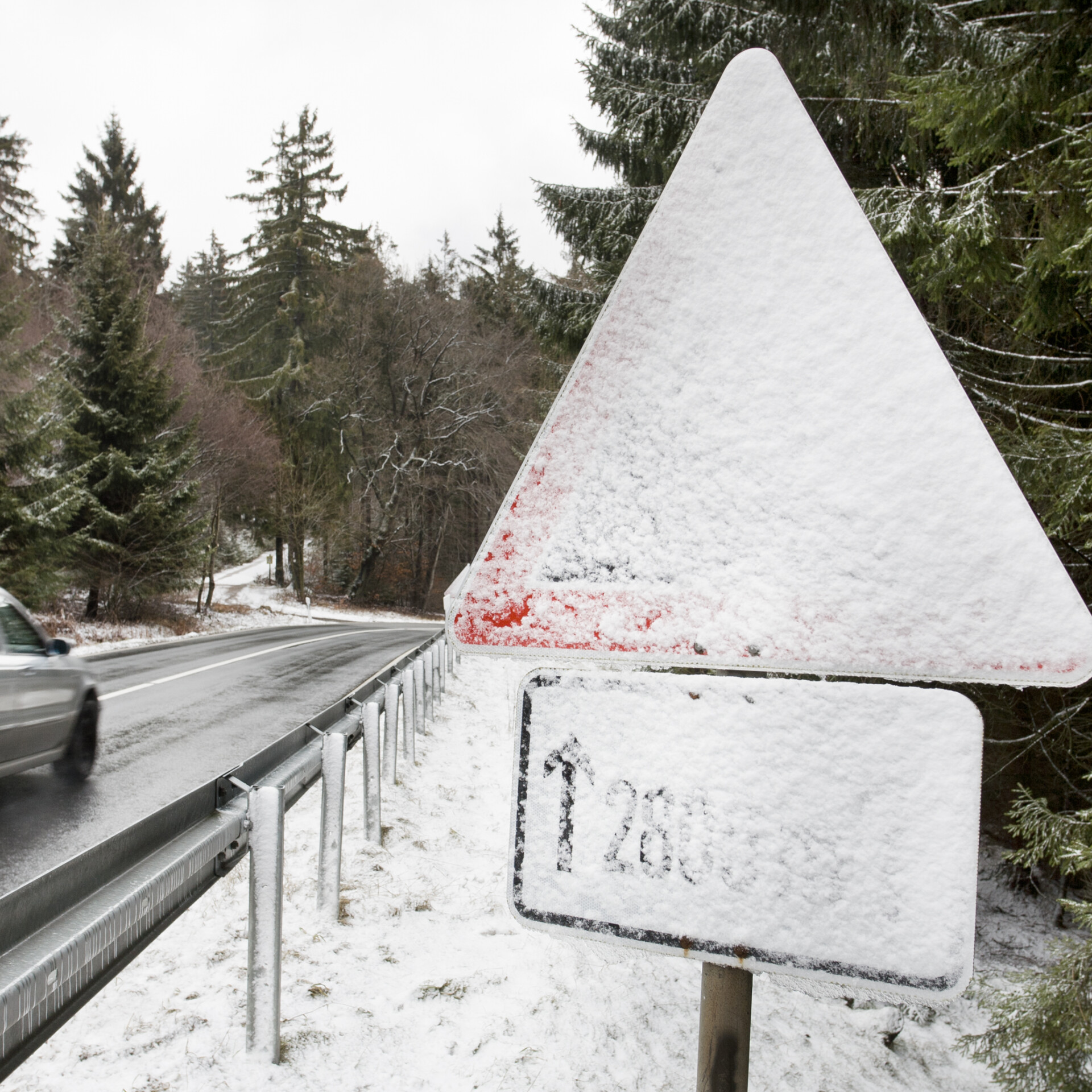
© iStock.com/ollo
For driver assistance systems and autonomous driving in particular, it is absolutely vital that artificial intelligence (AI) delivers reliable information from what it perceives in its surroundings. It needs to identify road signs and respond accordingly so that it can take the right course of action in any given traffic situation. But if the visual appearance of these signs is altered by environmental conditions, dirt or even vandalism, the result can be problematic — and dangerous — misinterpretations by the system. If it incorrectly interprets an obscured or dirty stop sign, for instance, an autonomous vehicle might speed up instead of braking.
How do these misinterpretations happen?
The three most important technical requirements for autonomous driving are machine perception, situational comprehension and lane maneuvering. Cameras and sensors scan the environment in real time, while (D)GPS location systems provide additional information gleaned from the surroundings. All of this data is constantly merged and analyzed, resulting in the machine-based perception of the vehicle’s environment. Based on this perception, artificial intelligence then generates a model of the environment and uses it to record the situation at hand and make forecasts. These forecasts are used to decide which steps should be taken next: for example, when the vehicle should brake, speed up or change lane.
However, if the cameras record manipulated input data, such as modified road signs or traffic lights that are difficult to see, AI makes false assumptions about the situation at hand and ultimately makes the wrong decisions. In the case of image recognition systems based on neural networks, only a very small number of input pixels need to change in order for the system to make a wrong decision — this could be a few leaves, a reflection of sunlight or an advertising sticker. Even bad weather or the failure of individual cameras and sensors could — at any time — cause AI to generate a flawed model of the driving situation and then act based on this.
Possible solutions based on research from Fraunhofer IKS
In light of this, it is vital that the system is able to monitor itself and evaluate its own state and level of dependability. Accordingly, AI needs to constantly question itself and its decisions. In its research, the Fraunhofer Institute for Cognitive Systems IKS adopts a holistic approach to AI assurance, as Adrian Schwaiger, Research Fellow at the institute, explains: »We are working on making artificial intelligence more robust and self-critical, yet are always thinking about how it is embedded in the software architecture as a whole. We analyze the entire system and examine the AI as well as any gaps it has from various perspectives.«
We analyze the entire system and examine the AI as well as any gaps it has from various perspectives.
Research Engineer at the Fraunhofer Institute for Cognitive Systems IKS
For example, scientists are conducting research into the intelligent cross validation of internal and external sensor data with different weak points. Redundancy is the key concept here: Various sensors and cameras deliver data that becomes plausible when cross-checked and combined with GPS map material, for instance. A stop sign on the highway even though the speed limit sign just read 120? That would be unlikely, so braking wouldn’t be advised!
Adaptive software architectures can also be hugely beneficial in this context. These systems independently adapt to changing conditions in the environment. For example, if a sensor fails, a camera is covered up or erroneous input data is provided by road signs, the software architectures have to suggest alternative, safe patterns of behavior for the autonomous vehicle. Researchers are working on resilient software architectures that are able to respond dependably, even when faced with unforeseen and unknown challenges.
Driver assistance systems such as lane assistance systems, parking aids and emergency braking systems are already doing a lot of the work for the driver. But artificial intelligence will need to be much more robust before we can sit back and read the newspaper while driving an autonomous vehicle!