Read next
Smart farming
Digital transformation takes the field
As farmers step up their use of information and communication technology, agriculture is not only becoming more efficient, but also more sustainable and resilient. A Fraunhofer IKS webinar explored some different solutions and strategies in the field of smart farming.
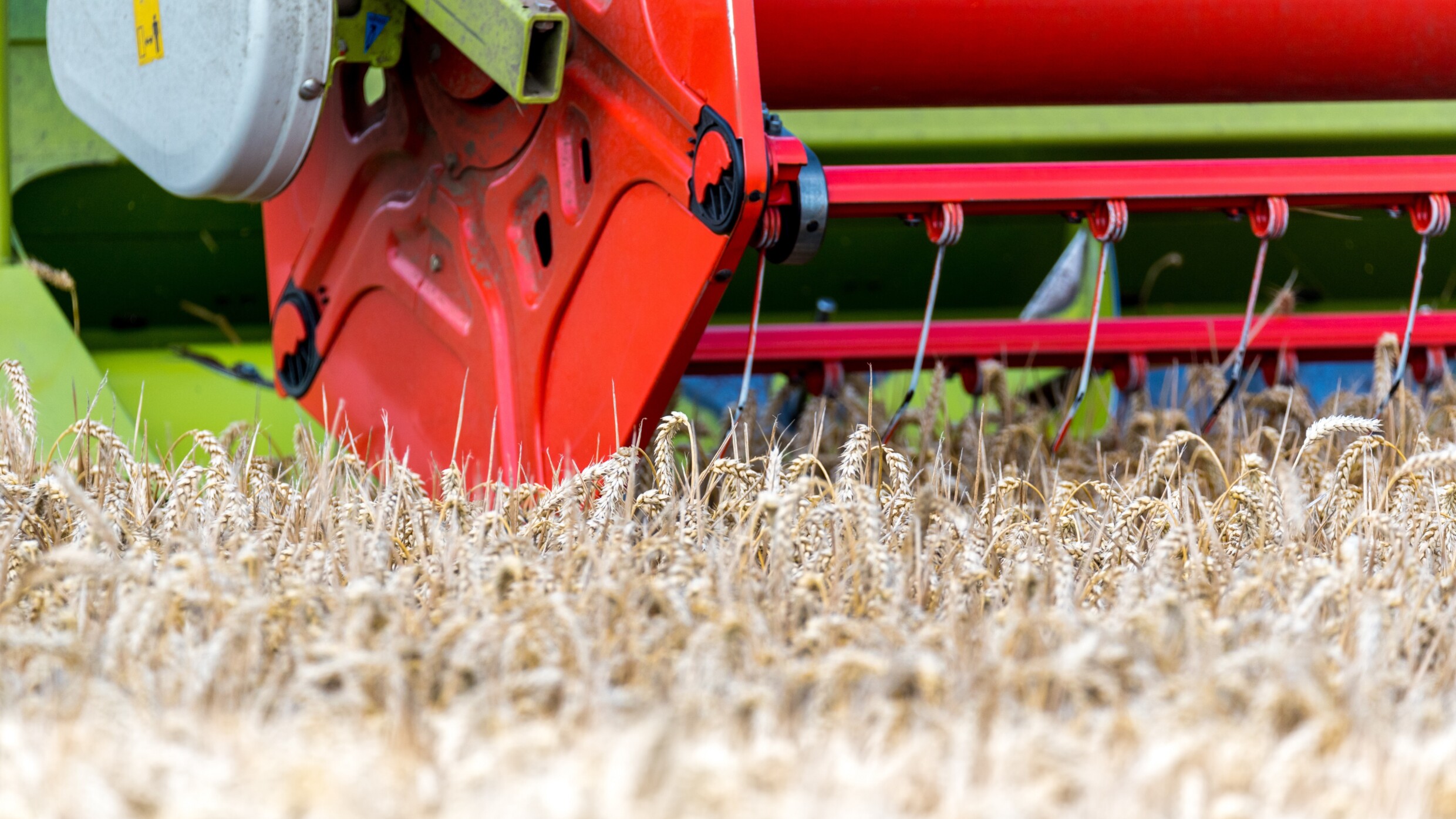
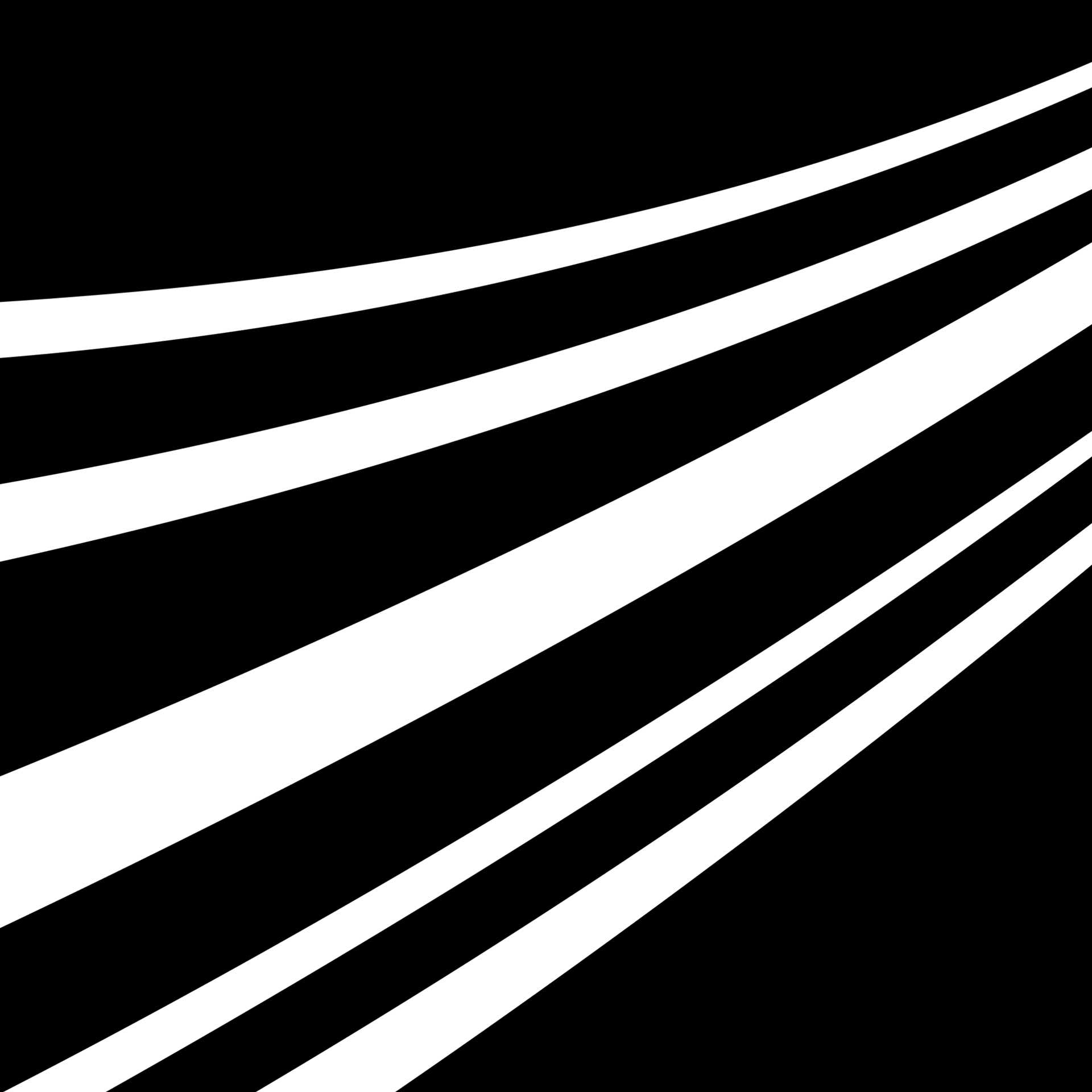
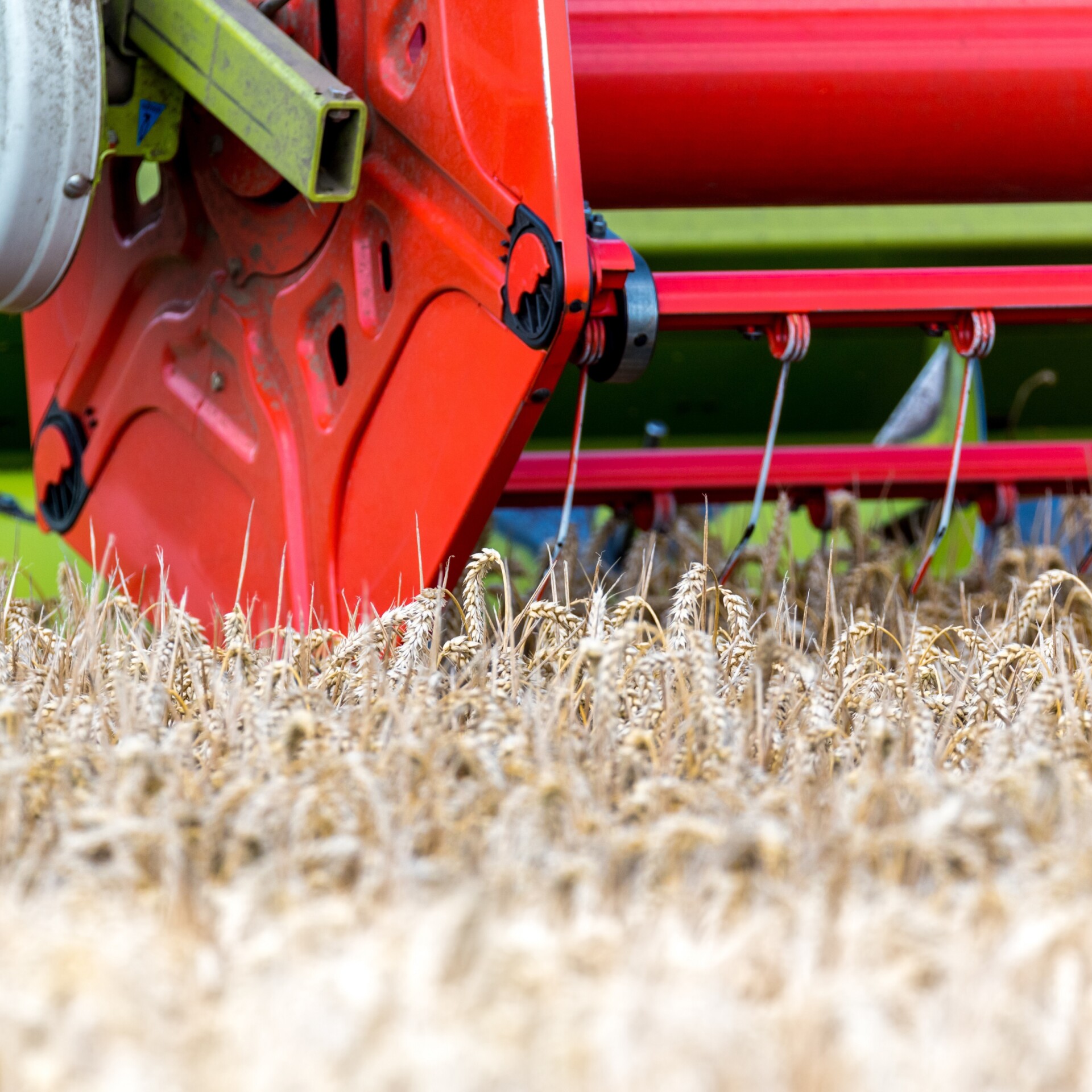
© iStock.com/i Stockr
When the subject of autonomous driving comes up, people tend to think of roads rather than farms. But digital transformation, the Internet of Things and artificial intelligence have long since made their way into the agricultural sector, without attracting much attention from the general public — and autonomous vehicles have come along for the ride. These days, evaluating sensor data to identify the environment, cooperative driving maneuvers with connected vehicles and even "platooning” are all part and parcel of modern agriculture.
Sustainability: The driving force of modern agriculture
“From the road to the field”, a webinar hosted by the Fraunhofer Institute for Cognitive Systems IKS, focused on the opportunities and challenges that this development presents. One of the speakers, Prof. Peter Pickel, deputy director of the JD European Technology Innovation Center at the agricultural machinery manufacturer John Deere, gave a presentation on a new technology that uses artificial intelligence and a whole series of cameras to detect weeds — a vivid demonstration of the kind of opportunities that digital transformation in agriculture can unlock. Far more precise than conventional methods, this technology allows a 50–90 percent reduction in the use of herbicidal sprays.
As such, it’s an excellent example of a real world application for two out of the four technologies highlighted by Prof. Pickel as key enablers — namely sustainable technologies and high-precision, autonomous machinery. His other top picks for drivers of digital transformation in agriculture were the switch to electric engines and the Internet of Things. For John Deere, these technologies are the key to overcoming the challenge of climate change while still ensuring reliable food production. That’s why the company has come up with two guiding principles for farmers: “Produce more with less” and “Independence” — meaning independence in the future use of resources in particular.
To give his audience a glimpse of the future, Prof. Pickel drew parallels between a possible farm management system and the systems used in industry. According to his projections, this farm management system would combine functions equivalent to ERP, MES and process control systems. In terms of real-world work processes, he predicted an increase in the use of monitoring devices and sensors, a trend that he called “vertical integration.” He contrasted this with “horizontal integration,” where these processes are transferred completely to the cloud, and encapsulated the two developments in another newly minted term: “Agriculture 4.0.”
Autonomous driving: Cooperative risk detection in action
In the next presentation, the approximately 60 participants journeyed from the fields to the roads with Josef Jiru, who is responsible for the automotive field within Business Development at Fraunhofer IKS. Jiru highlighted various use cases for autonomous driving, such as cooperative perception, for example, which effectively illustrates the opportunities and challenges that come with connected, autonomous driving.
This technology would allow vehicles to share their observations of their surroundings on the roads with each other, and so to avoid dangerous situations before they have a chance to occur. As an example, Jiru described a situation where a car is about to overtake a truck; if the truck shares the information from its own sensors with the car, the car will be able to perceive much more of its surroundings. So if the overtaking maneuver were unsafe, it could be prevented before it ever began. This would also benefit road users whose vehicles are not equipped with sensors, but do have a communication interface. However, data quality and data integrity are crucial here, with both requiring robust AI and a secure communications network. This is especially important when the data is used as a basis for decisions about safety.
Bringing cooperative vehicles to smart farming
As Jiru went on to explain, autonomous vehicles can also be used in cooperative driving maneuvers and “platooning,” where a group of vehicles drives very close together. These use cases come with their own special requirements, such as reliable data and, as a particular prerequisite, a system for process monitoring. However, some key questions for this technology have yet to be answered. For example, what happens when unexpected conditions occur, such as a deterioration in visibility? How do you ensure that the cooperative maneuver is safely aborted under these conditions?
Find out more!
If you want to know more about application fields for Fraunhofer IKS research into smart farming, please contact our Business Development team to arrange a one-to-one meeting.
Here, the automotive expert drew parallels to the field of smart farming, where swarm technology and, consequently, cooperative driving maneuvers are becoming ever more important. He also saw plenty of potential for cooperative perception in the smart farming domain. For example, a drone could search the field for wild animals, such as deer, before an approaching combine harvester puts them in danger.
Jiru finished his talk with a concise summary: “Despite their natural differences, the smart farming sector can really benefit from what the automotive sector has to offer, especially with regards to interoperability, standardized message formats, safety strategies and sensor technology.”
Tried and tested reliability
Finally, Dr. Chih-Hong Cheng, Head of the Safety Assurance for AI department at Fraunhofer IKS, gave some insight into current research and developments in the field of autonomous vehicle testing. Addressing the ISO/TR 4804 standard, he emphasized the absolute necessity of taking into account qualitative tests as well as quantitative, statistical tests, for example, by defining equivalence classes or reusing accident scenarios. This would prevent a situation where the only measure of reliability is the number of kilometers driven.
There are two dimensions to these kind of qualitative tests — breadth and depth. In this context, breadth means using a small number of example scenarios to test for all requirements, with a view to increasing efficiency. Meanwhile, depth refers to the active search for bugs and vulnerabilities in the system.
These new requirements for tests have given rise to a number of research questions that Fraunhofer IKS is currently working on. For example, it’s still not clear exactly how the previously mentioned bug hunting process will work at a technical level. Another question in need of clarification is how best to define the operational design domain, i.e. the area where an autonomous vehicle must function safely.
Given the high level of interest this event attracted, Fraunhofer IKS is taking the opportunity to continue this series of webinars over the coming year. You can find the topics and dates of these seminars here.