Read next
Searching for the unknown
Uncovering difficult situations for autonomous driving systems
Today, autonomous vehicles function reasonably well in test situations since the conditions are severely restricted and thus easy to manage. A key issue however is how to design autonomous vehicles so that they operate dependably even in complex and previously unknown situations. A solution from Fraunhofer IKS is helping to uncover and predict such difficult situations.
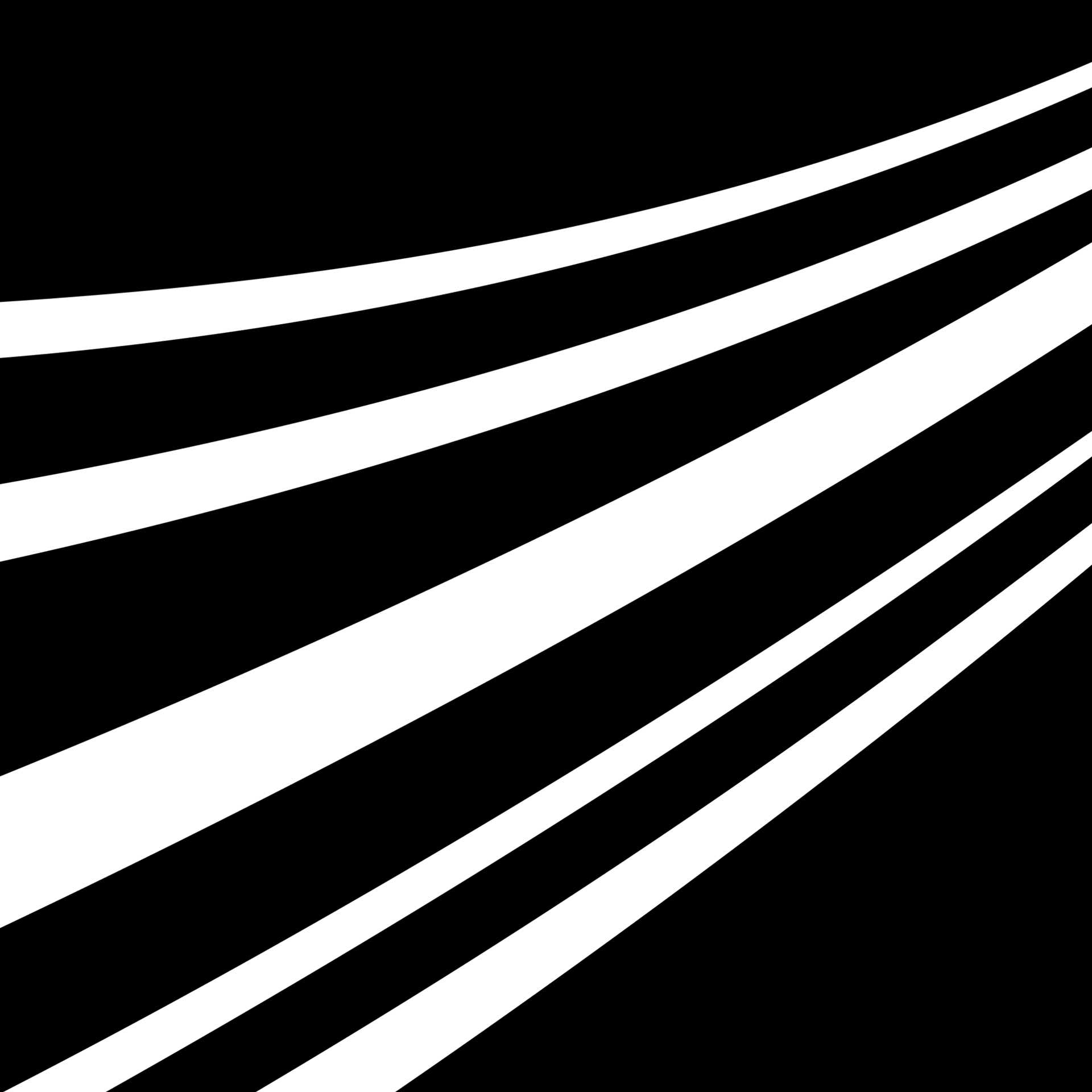
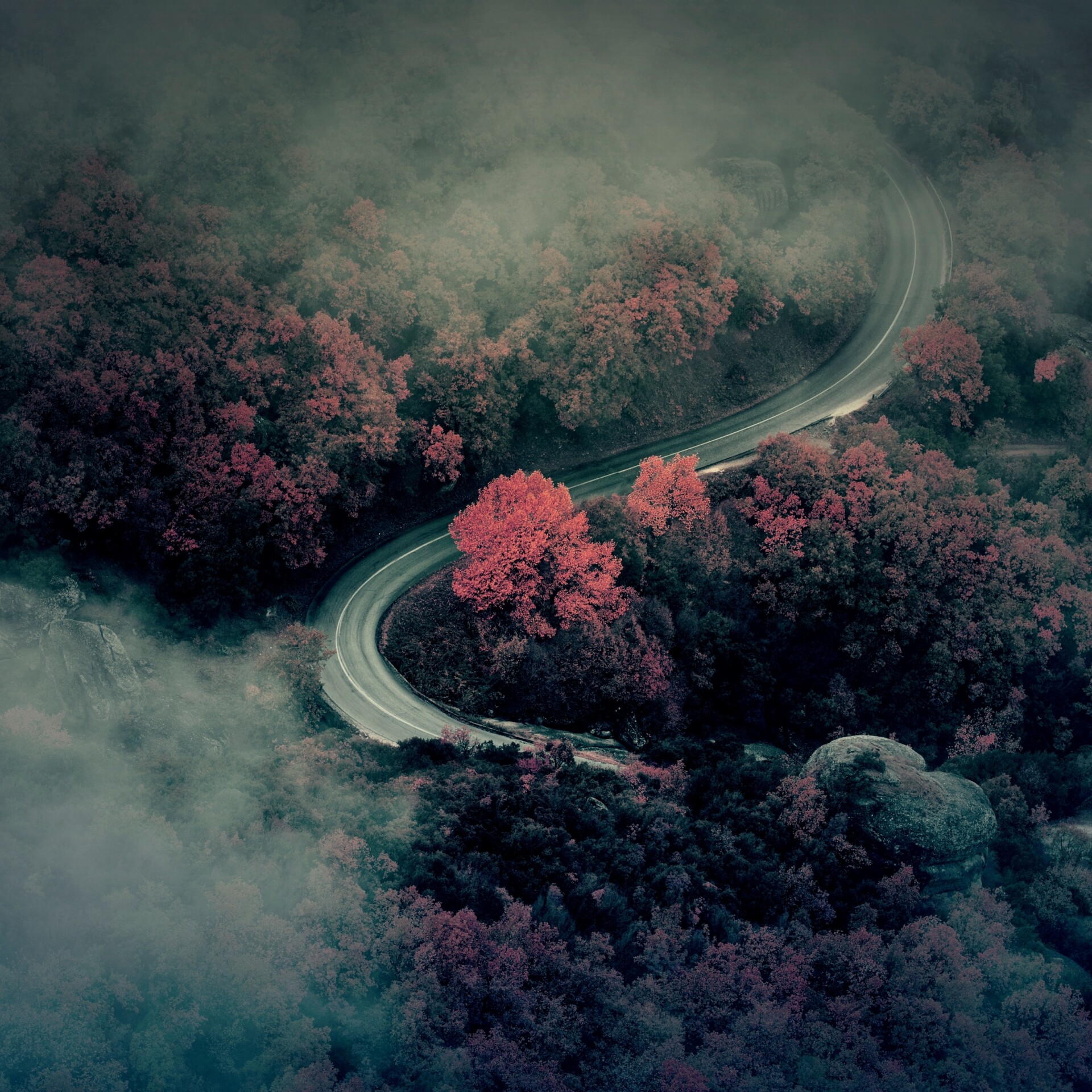
© iStock.com/AlxeyPnferov
Even though a wealth of discoveries has been made since the Scientific Revolution of the 16th and 17th centuries, a lot remains unknown. This applies to a variety of topics such as dark energy in physics, how to solve the Millennium Prize Problems in mathematics, why the final season of Game of Thrones was so bad and what difficult situations an AI based autonomous driving system might encounter. What distinguishes the latter from the others is the risk that is involved, in particular to human lives.
Difficult situations for autonomous driving
What does difficult situations mean in the context of autonomous driving? Exactly the same as for human drivers; namely something unfamiliar, complicated, something we did not encounter before or cannot fully and immediately comprehend. The causes of such situations are diverse: a confusing intersection, unclear traffic regulations, poor visibility or simply being preoccupied. All these factors – hopefully with the exception of being preoccupied – are also critical for autonomous driving systems with AI elements – maybe even more.
Why driving lessons are not enough
Many countries have a minimum age at which driver’s licenses can be obtained. This requirement for a certain level of maturity is designed to ensure that drivers make rational decisions when facing difficult situations. The underlying idea is that such behavior can only be guaranteed beginning with a certain age. How about AI systems? Well, we certainly cannot wait until the AI elements train for the duration of the minimum age required (nor is it necessary). What we ultimately want is that the system makes correct decisions – always. Unfortunately, the always part is not easy to prove. In this case, a principle similar to the driving lessons that people take applies as well. That is, you can only cover a small number of all potential situations and hope the remaining ones will resolve on their own when they arise. However, hoping is not good enough for Artificial Intelligence. This epistemic uncertainty of AI is viewed in a more critical light than faulty human decisions.
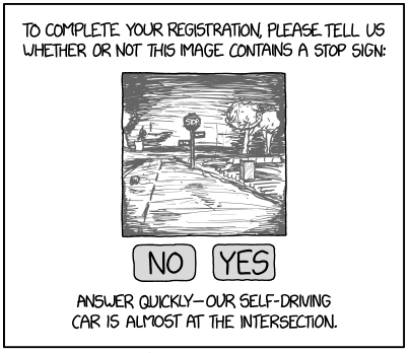
© https://xkcd.com/
(c) xkcd
And even if we assume to have an AI system that works reasonably well from an overall standpoint, what if the input is incomplete or faulty? Here again, neither a human nor an AI system can react to something it cannot sense, even though sensing means something slightly different in the context of AI than it does for humans, of course. One could argue that we are not blind to our own shortcomings and therefore utilize more than just camera systems for sensing. In fact, self-driving cars are equipped with many different sensory perceptions, but as is often the case with technological devices, each has its own advantages and disadvantages. The sheer number and variety of sensors alone cannot mitigate aleatoric uncertainty, meaning a misrepresentation of the actual environment caused by inaccurate measurements.
Fraunhofer IKS uncovers and predicts difficult situations for automated driving systems
We now know what specifies difficult situations and how their individual parts are connected. However, specific difficult situations still remain unknown.
To uncover and predict these unknown difficult situations for automated driving systems, Fraunhofer IKS is developing a solution as part of the research subproject Dependable Environment Perception within the establishment of the institute, which is being funded by the Bavarian Ministry of Economic Affairs, Regional Development and Energy.
Uncovering unknown situations is far from a trivial process. Up to this point, we have been discussing AI elements and sensors. However, autonomous driving systems contain many more components, such as fusion units, GPS localization including maps, V2X and others. All of these components, which accomplish specific tasks, have a huge impact on the automated driving system as a whole.
The principle of our solution is to enable all of the essential components and their connections to be modeled during the design phase. The resulting system is then analyzed using uncertainty propagation, thus revealing difficult situations based on uncertainty. High uncertainty does not necessarily mean the system will categorically make a mistake, but it means that the specific situation generates many different potential outcomes. When developing autonomous driving system architectures, the solution can help during the early phase to identify situations that the system will have difficulty dealing with. Which situations this involves depends on the functionality of the system and its core components. For example, critical scenarios for automated emergency braking systems are different than those for automated parking.
Ultimately, the solution and the results of the corresponding research project will enable users to improve their systems so that previously unknown situations are incorporated into the design, thus working to ensure that safe autonomous driving becomes reality.
This activity is being funded by the Bavarian Ministry for Economic Affairs, Regional Development and Energy as part of a project to support the thematic development of the Institute for Cognitive Systems.