Read next
DEEP series, part 4
The process is directed by DEEP
The previous three parts of our series focused on the technologies “under the hood” of DEEP, the Fraunhofer IKS machine learning toolchain. Now, we take a look at the “big picture” in form of the process steps of the DEEP procedure – how DEEP can be used to get a grip on the problems associated with the use of machine learning (ML) for future flexible quality inspection.
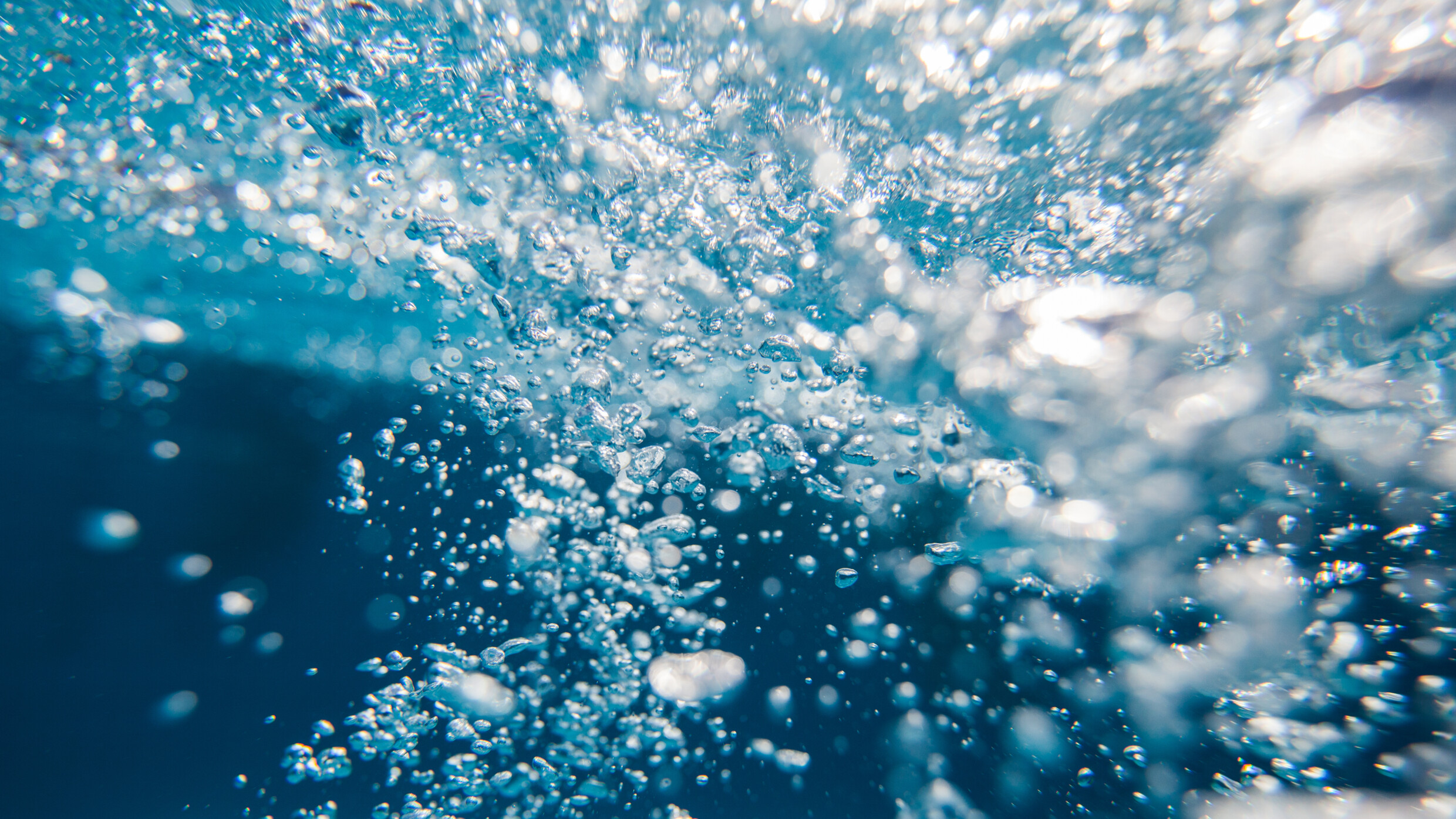
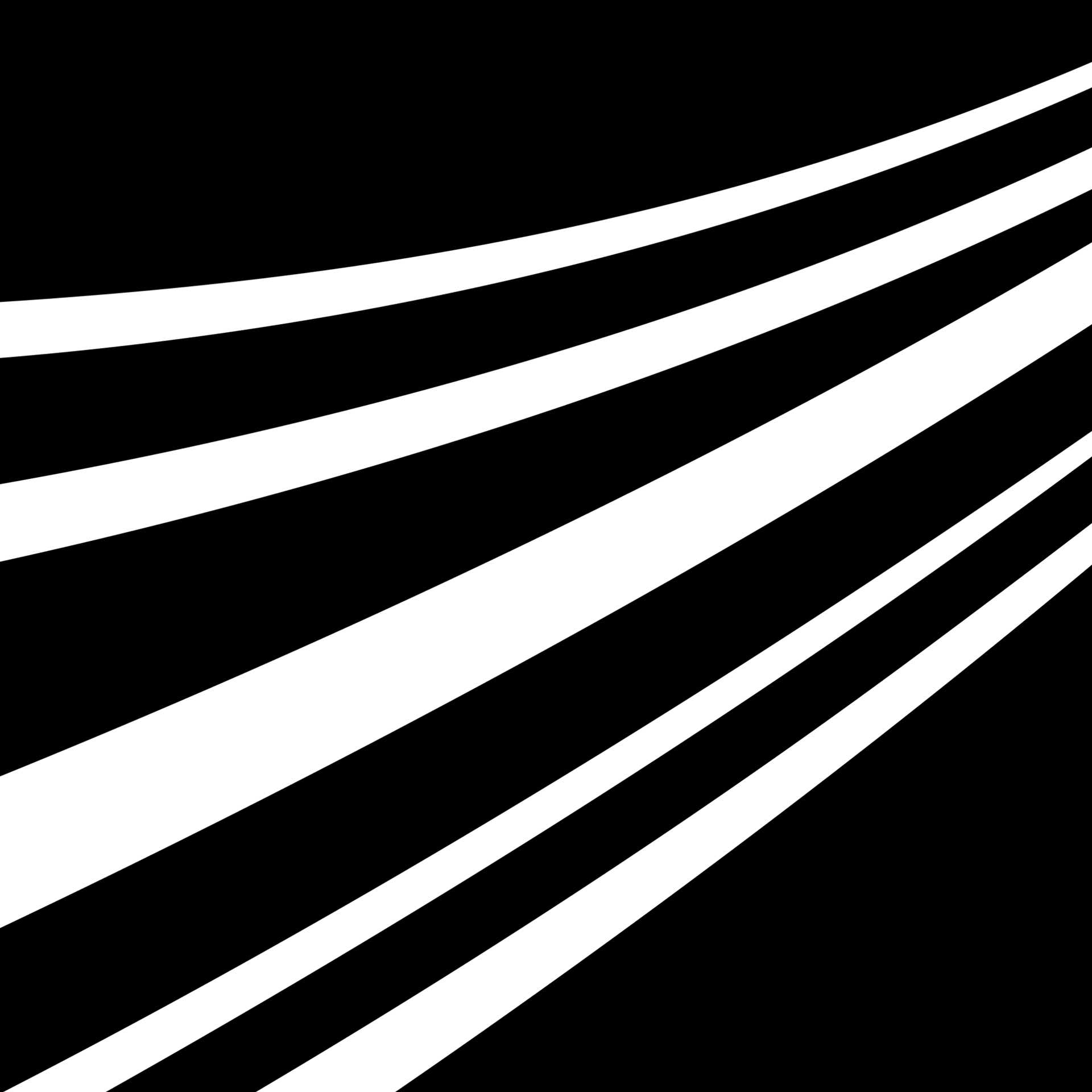
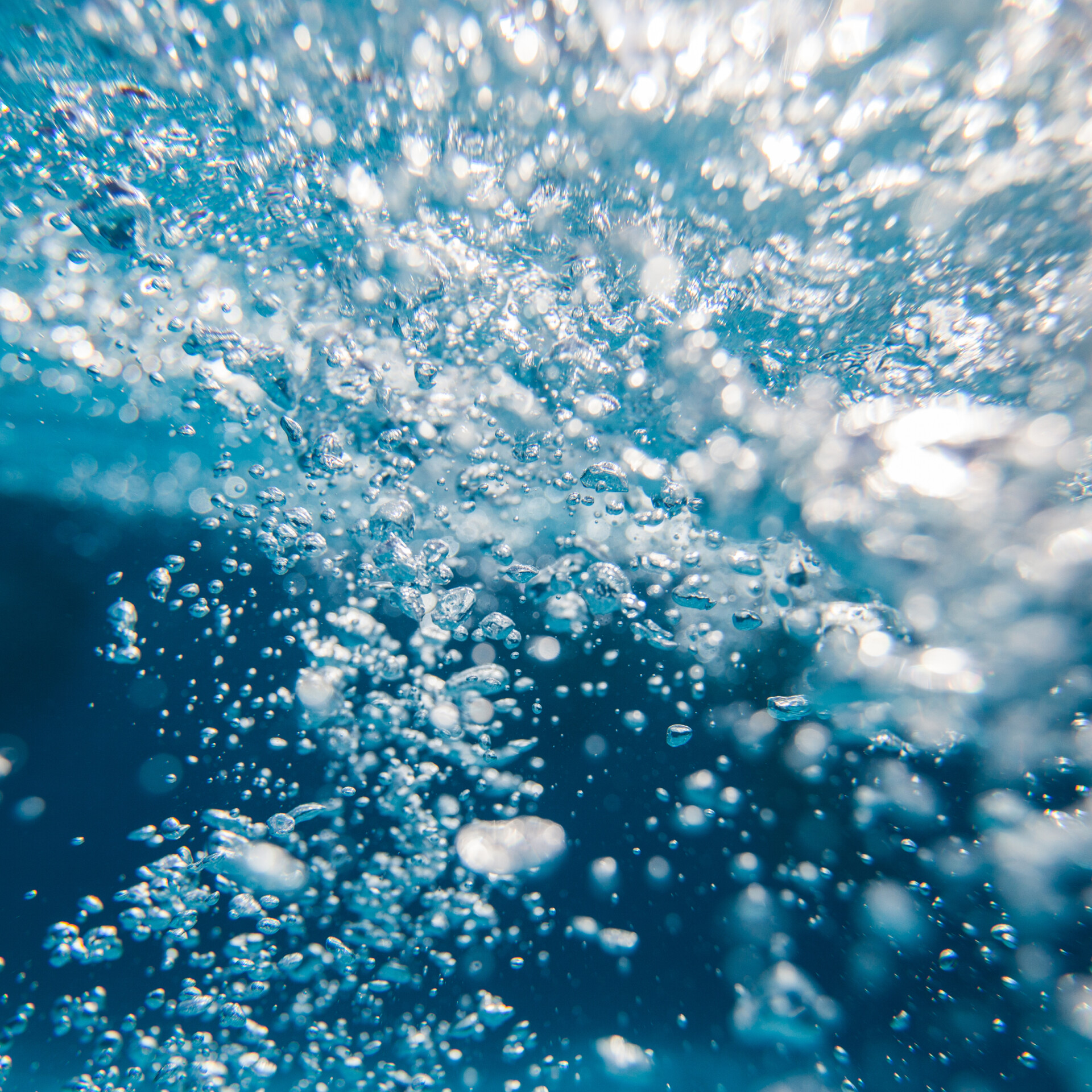
© iStock/maksym
With DEEP (Data Efficient Evaluation Platform), it is possible to evaluate the potential of data for image recognition using artificial intelligence (AI) at the “push of a button” and find out which machine learning (ML) models are particularly suitable for the specific data of an application. To do this, DEEP automatically combines various specific technologies developed at the Fraunhofer Institute for Cognitive Systems IKS, such as FAST, Robuscope and Modular Concept Learning, which have already been covered in detail in the previous parts of this series in the Safe Intelligence online magazine.
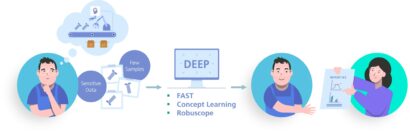
© Fraunhofer IKS
Fig.1: Important components of DEEP (Data Efficient Evaluation Platform), the machine learning toolchain for the reliable AI-supported automation of quality inspection systems, are the technologies FAST, Robuscope and Concept Learning. They are the result of research at the Fraunhofer Institute for Cognitive Systems IKS, as is DEEP as a whole.
Today's quality inspection managers, for example in manufacturing, are faced with the problem of identifying defects reliably from a complex data situation with both sensitive data and often few examples of defects. Methods based on machine learning (ML) can help here, as they specifically allow the automation of process steps. However, identifying and evaluating suitable methods is a major hurdle for their use.
DEEP uses a detailed report of results based on an analysis to provide answers to critical questions that are crucial for the successful implementation of AI in industrial processes:
- How good is the accuracy of image analysis using machine learning based on the available data?
- Which specific models are particularly suitable for analyzing and processing the data provided?
- How robust is the model? In other words, how reliable are the results under different conditions?
By answering these questions, DEEP helps companies to realistically evaluate the feasibility of AI-supported image recognition and to make well-founded decisions about the use of AI in their operations.
How DEEP works
The DEEP process steps are simple. After uploading the image data to the DEEP tool, this data is pre-processed and divided into training data, test data and validation data. Based on the data, the tool develops and tests various ML models with different training parameters and for different application scenarios. This identifies the models that fit the specific requirements and data situation best. Specific models for sub-problems of the data set can be generated as a sub-domain in this step (see article on FAST) and the various concepts and suitable associated models can be identified (see article on concept models). The performance of the various models is analyzed in terms of their accuracy and robustness, using a wide range of common and specially developed methods (see article on Robuscope). Based on these analyses, targeted recommendations are derived as to which models are most suitable for the specific data and summarized in a corresponding report.
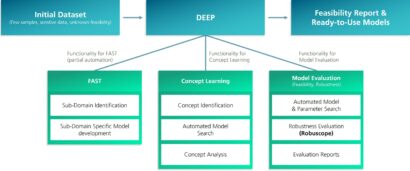
© Fraunhofer IKS
Fig. 2: Process image of the DEEP machine learning tool chain with its FAST, Concept Learning and Robuscope components. The components can be combined, for example FAST for concept models.
Technical areas of application
The field of application of the automation system presented here is far-reaching. A process that can be automated by this system needs to have the following characteristics: It should allow automation decisions to be made by experts, by an external system or by a user interface. The automation decision can either
- be made by an expert “in-process” (example: an expert centrally monitors the products of several production machines) or
- be processed with a delay (example: pre-processing of image data for medical diagnosis, which, if necessary, is processed by an expert at a later point).
Example: Quality control in manufacturing
The aim is to check a component with a longer runtime in production for errors. The component belongs to a new product series, so there is not much training data available yet. In addition, defects are rare and there is the possibility of new types of defects that have not yet been documented. During operation, each component is inspected by using a camera. The camera operates in an open area, which is why environmental parameters such as lighting can change. In addition, the components are recorded with the camera in various angles.
Currently an expert must be present at the production site to inspect the parts. Parts that do not meet the quality requirements get discarded.
Thanks to automation, a camera carries out the inspection instead of the expert. A sorting system/robot arm sorts out problematic parts. The expert has a user interface that shows the parts that have not been processed automatically. He can control the sorting of these parts through user input. The input data and the decision are recorded for future model improvements.
Example: Inspection of screw and plug connections with a robot arm
For a complex component, which has many screw and plug connections, it should be checked whether all connections are correct. To do this, a robot arm moves to the relevant positions and takes pictures of the component. Currently, an expert carries out the inspection visually.
Automation allows him to monitor several of these stations simultaneously. A user interface provides the automation system with feedback for situations in which it cannot make a reliable statement. Input data and the decision are recorded for future model improvements.
Example: Medical preprocessing
In a routine procedure, medical data such as X-ray images are collected. These images must be inspected by a radiologist. This inspection takes place with a delay. In addition, expert knowledge is very cost intensive. Currently, the experts work through the data provided in a sequential manner and decide for instance whether a disease is benign or malignant.
If you have any questions regarding DEEP or the automation of quality inspection in industrial applications, please contact business.development@iks.fraunhofer.de
Due to the automation, the data provided is automatically pre-processed. In all cases in which a reliable statement can be made, the data is automatically processed. Only in cases where no reliable statement can be made based on the given data, the expert has to carry out the inspection and make the decision.
Other use cases may include automated inspection for maintenance work, visual inspection in commissioning or checking compliance with safety measures (such as the wearing of safety equipment).
Conclusion
ML-based automation systems stand in sharp contrast to conventional methods. With DEEP, both partial automation systems and the (re)use of existing concept models can be used as solutions. The aim here is to minimize the development time of these systems up to productive use, the introduction risks and thus also the costs. If it is ensured that these solutions have low error rates, early deployment and incremental improvement are possible.
The focus on sub-domains also makes it possible to use ML models with less complexity. This means that resource limitations, interpretability requirements or permissible error rates can already be taken into account when selecting the model. Early use also increases user acceptance and understanding of the system. The integration of expert feedback during operation makes it possible to subsequently expand the degree of automation without additional data acquisition or annotation. In addition, changes in the data, for example due to changes in the production environment, can be automatically integrated into the system.
By using concept-specific models, not only can development time be saved, but domain knowledge can also be profitably integrated into the process, leading to greater understanding and a more robust overall solution. This modular approach also makes it possible to retain knowledge across changes in specialized concept models.
Small amounts of data and high demands on the reliability of the solution create a challenge in many industrial applications and make an intelligent selection of model and parameter combinations necessary. The DEEP machine learning tool chain provides ideal support in the development and selection of these combinations.
This work was funded by the Bavarian Ministry for Economic Affairs, Regional Development and Energy as part of a project to support the thematic development of the Institute for Cognitive Systems.