Read next
DEEP Series, Part 2
Insufficient Data? No Problem!
DEEP, the machine learning toolchain of Fraunhofer IKS, enables the automation of quality control in production environments, even if the requirements are complex. The technologies FAST and Robuscope, likewise developed by Fraunhofer IKS, are essential components of this solution.
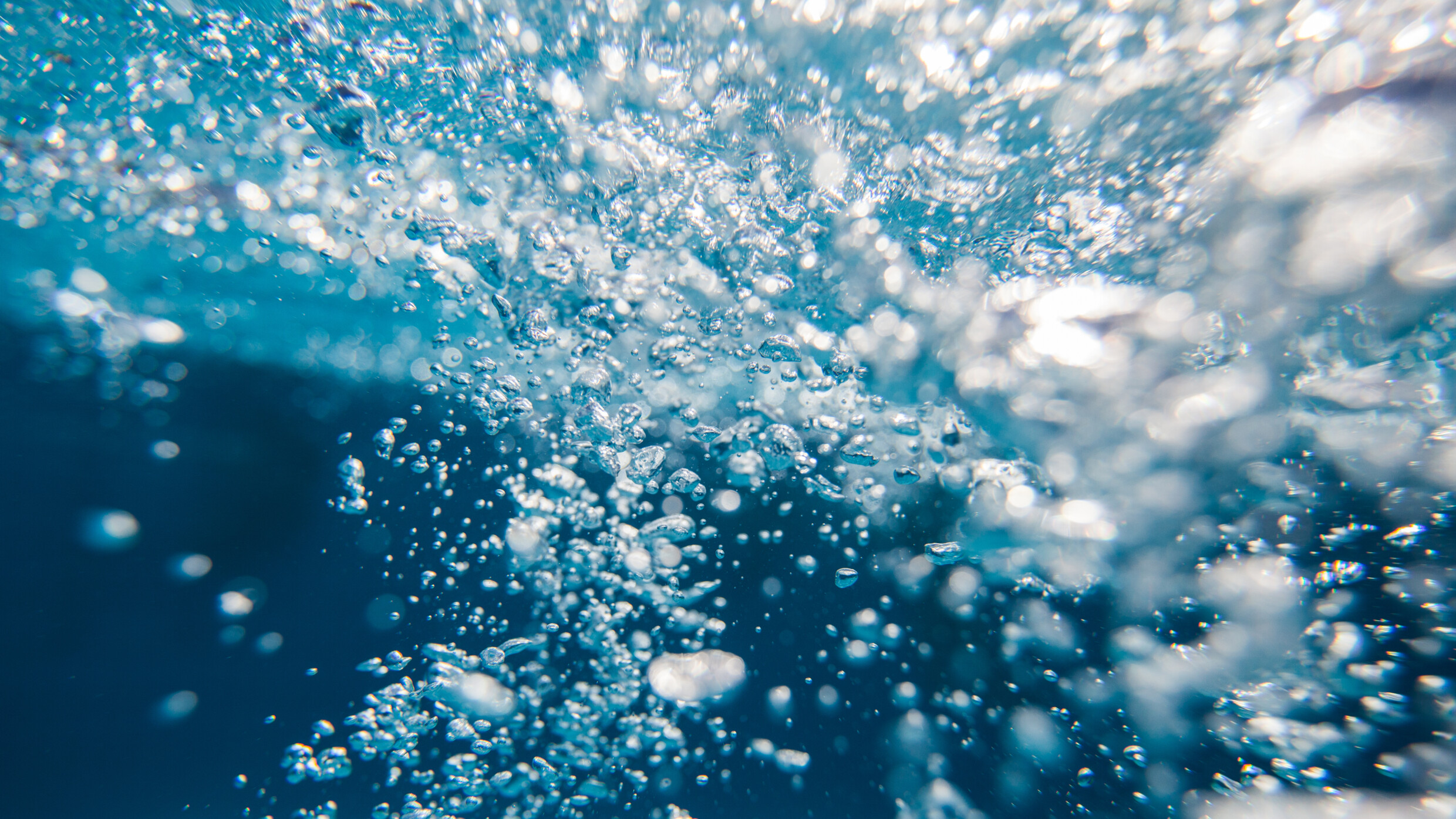
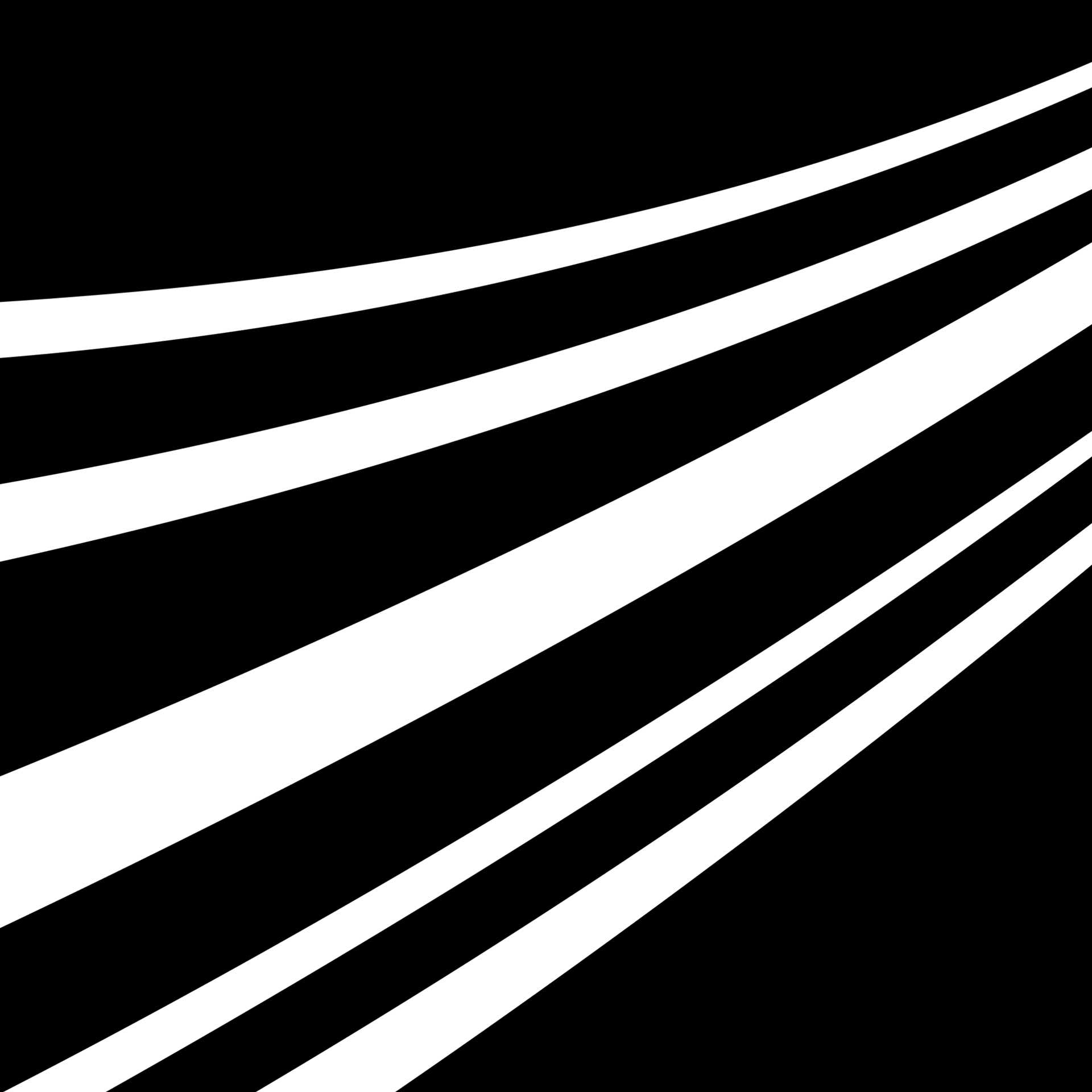
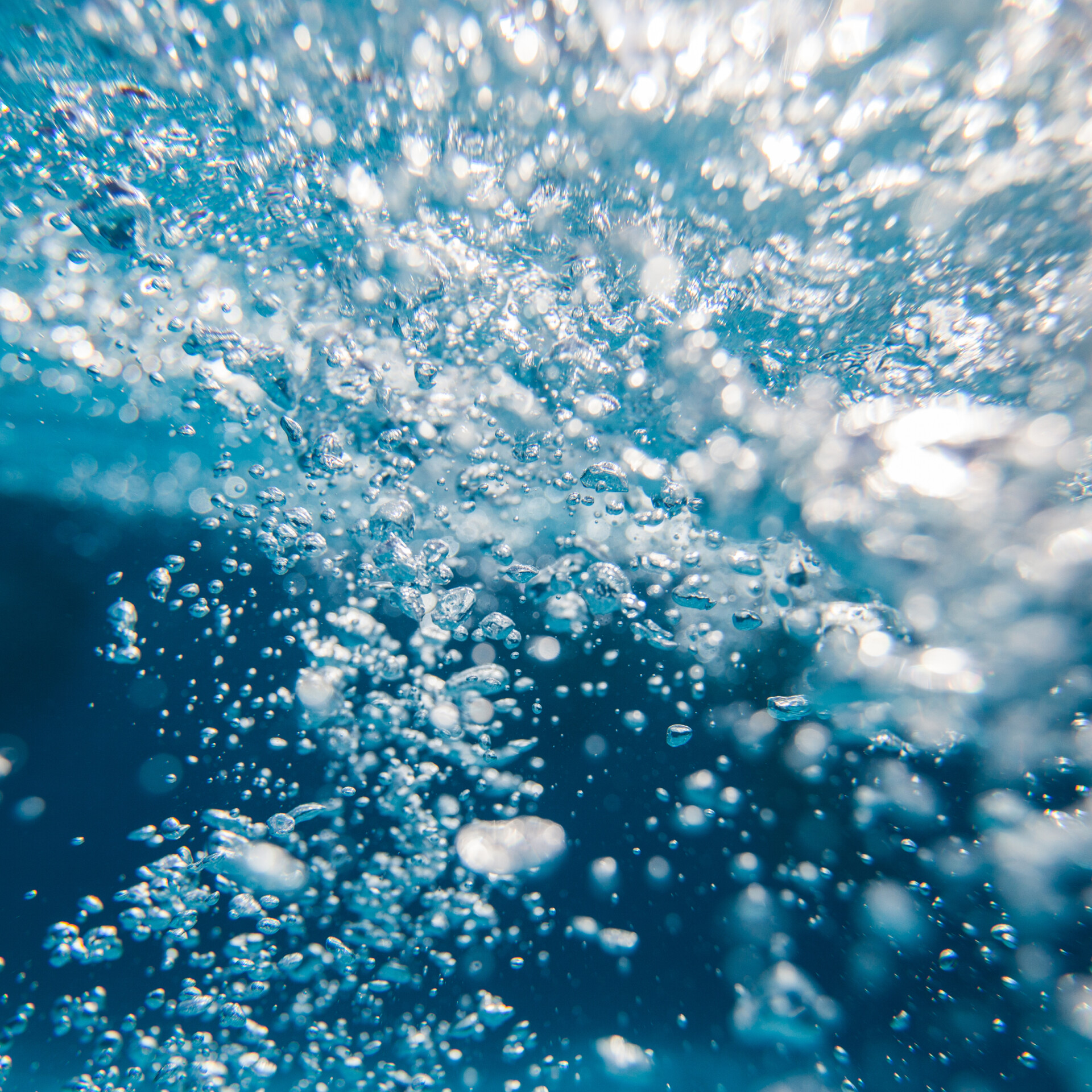
© iStock/maksym
DEEP (Data Efficient Evaluation Platform) is a machine learning (ML) toolchain for reliable AI-assisted automation of quality inspection systems. DEEP enables evaluating the potential of data for image recognition using artificial intelligence (AI) at the click of a button and finding out which ML methods are particularly suited for the specific data of an application. For this purpose, DEEP automates various specific technologies which have originated at the Fraunhofer Institute for Cognitive Systems IKS: FAST and Robuscope, among others.
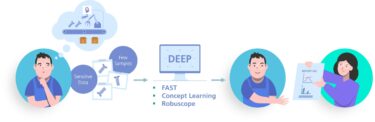
© Fraunhofer IKS
Fig. 1: The technologies FAST, Robuscope and Concept Learning are important components of DEEP (Data Efficient Evaluation Platform), the machine learning toolchain for reliable AI-assisted automation of quality inspection systems. Just like DEEP as a whole, they are the result of research at Fraunhofer IKS.
Fraunhofer FAST: partial automation thanks to subdomain models
FAST (Feedback-guided Automation of Sub-Tasks) is a reliable AI-assisted approach describing a system for the partial automation of decisions based on image data. Partial automation here means that the algorithm decides which input data can be automated reliably and for which data a manual decision informed by expert knowledge is required.
At the core of the approach is an ML system which processes automation decisions reliably, even if the data is insufficient in quantity and quality. This system is based on the idea of reducing the application domain to one subdomain and therefore to one subproblem. The subproblem is chosen based on specific characteristics of the data, so that the subset of data matches a sufficient data quality. As a result, the chosen subproblem can be solved reliably, even if an insufficient amount of high-quality data is available.
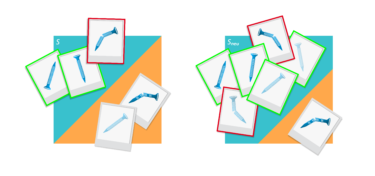
© Fraunhofer IKS
Fig. 2: Automated data selection for the quality inspection of screws; on the left, with initial safe detected quality of screws (cyan-coloured area) and, on the right, with the cyan-coloured area enlarged after improvement of the underlying data
Figure 2 shows an example of this selection process by means of the problem of quality assurance of screws. The figure on the left shows the originally available image data. The presented system now chooses the data points for which sufficient data with good quality is available, shown in the figure on the left as the set 𝑆. Images with low contrast, background artifacts such as shadings or reflections or rarely occurring defects which are not sufficiently represented in the dataset are not selected. The system automates quality control only from images of 𝑆, while the underrepresented images with low contrast, background artifacts or rare defects are forwarded to the expert. In the figure, the automatically rated images are highlighted accordingly in green (no defect) and red (defect), respectively. If, during regular operation, further data e.g. with low contrast is collected and rated by the expert, the set 𝑆 can be expanded with these images as soon as a sufficient quantity of them is available, resulting in the set Snew in the figure on the right. Now, quality control can additionally be automated for the images with low contrast.
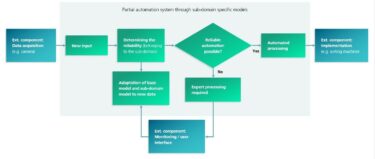
Fig. 3: The system model of FAST in use, including external components
Figure 3 shows an overview of the system model in use after a suitable subdomain was identified. It also lists the external components needed for a complete automation system, such as cameras and a sorting machine. The decision whether reliable automation is possible is at the core of the system model. If this is the case, the external execution component is initiated in order to perform the automation action. In case the input does not allow for reliable automation, expert feedback (annotation of the input) is provided via an external user interface and recorded for later extensions of the subdomain.
Fraunhofer Robuscope: a tool which makes specific suggestions for improvement
For more thorough analyses of a model, Fraunhofer IKS has developed Robuscope as a simple tool to test the robustness of an AI-based rating model. Robuscope is a component of the DEEP workflow. A wide range of established metrics highlights specific error characteristics and helps to develop a better understanding of potential weaknesses of the model.
Specifically, the analysis with Robuscope consists of several subfactors. First, a check is performed whether there are classes which are confounded particularly often and how reliable the AI model is in performing its task. This allows the discovery of systematic errors in particular. The final interpretation, however, strongly depends on the use case. If the majority of mistakes concerns classes which functionally result in similar behaviour, this is less critical. Example: different error patterns in quality control which both result in the rejection of the workpiece. If, on the other hand, a certain type of error is frequently confounded with an error-free workpiece, this poses a serious problem. Increased incidence of errors is often an indicator of a lack of training data – in particular for distinguishing the classes concerned.
Want to find out more?
If you have any questions about DEEP or the automation of quality inspection in industrial environments, please do not hesitate to contact business.development@iks.fraunhofer.de
Based on this, Robuscope analyses the stability of the predictions as well as the calibration of the output probabilities. Stable and therefore robust AI-based models are characterised by a sufficiently large gap between the correct result and the other, incorrect options, so that minor changes do not immediately provide an incorrect result. The calibration, on the other hand, describes how well the predicted probabilities (interpreted as confidence values) correspond to reality. In case of input data for which the model is very confident, it should always be right. Only if this is the case, the confidence can be used as an additional measure of the model’s reliability – e.g. to notify the user in case of low confidence or to use alternative algorithms instead of the model.
In this context, various options for use of the probability distribution of the model predictions are evaluated in order to reduce errors during use of the model. However, this is usually done at the expense of performance. The identification of an advantageous operating point by selecting a suitable threshold – i.e. the point beyond which the prediction of the model should be considered “unreliable” – is made possible by the comparison of residual errors and the remaining accuracy as well as other uncertainty-specific metrics.
Robuscope is also accessible outside of DEEP for all AI users. To do so, the user uploads a file to the tool with the predictions of the model to be tested – a probability distribution across the individual classes – as well as the expected result (ground truth). For this purpose, the web tool has reduced the required input data to the absolute minimum. Access to the trained model or potential sensitive training or test data is not required. In a few seconds, a detailed analysis report is generated directly in the browser, providing information about the quality of the predictions of the model to be used. This report can also be downloaded for further analysis and includes specific suggestions for improvement which are intended to help the application experts with developing more reliable models.
Following FAST and Robuscope as important components, the third part of the series of blog posts will focus on another important technology of the DEEP tool chain: Fraunhofer IKS Concept Learning, which enables the reuse of already trained models and therefore efficient and long-term use for frequently occurring concepts.
This work was funded by the Bavarian Ministry for Economic Affairs, Regional Development and Energy as part of a project to support the thematic development of the Institute for Cognitive Systems.