Read next
Machine learning
Robuscope: Quick check for AI
A straightforward online tool makes it possible to test the robustness of an AI model – and does so within a matter of seconds. The tool is called Robuscope, and has been developed by the Fraunhofer IKS.
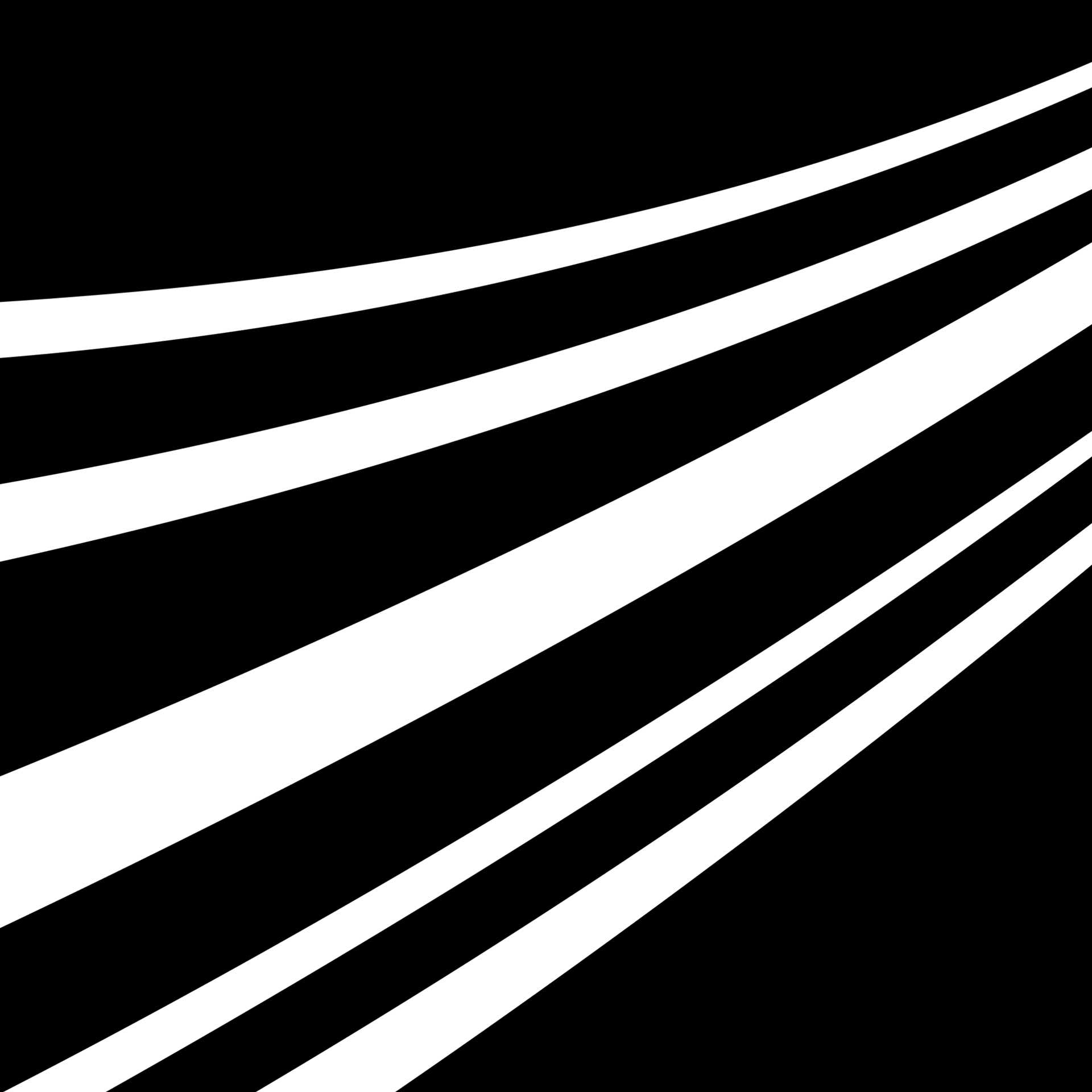
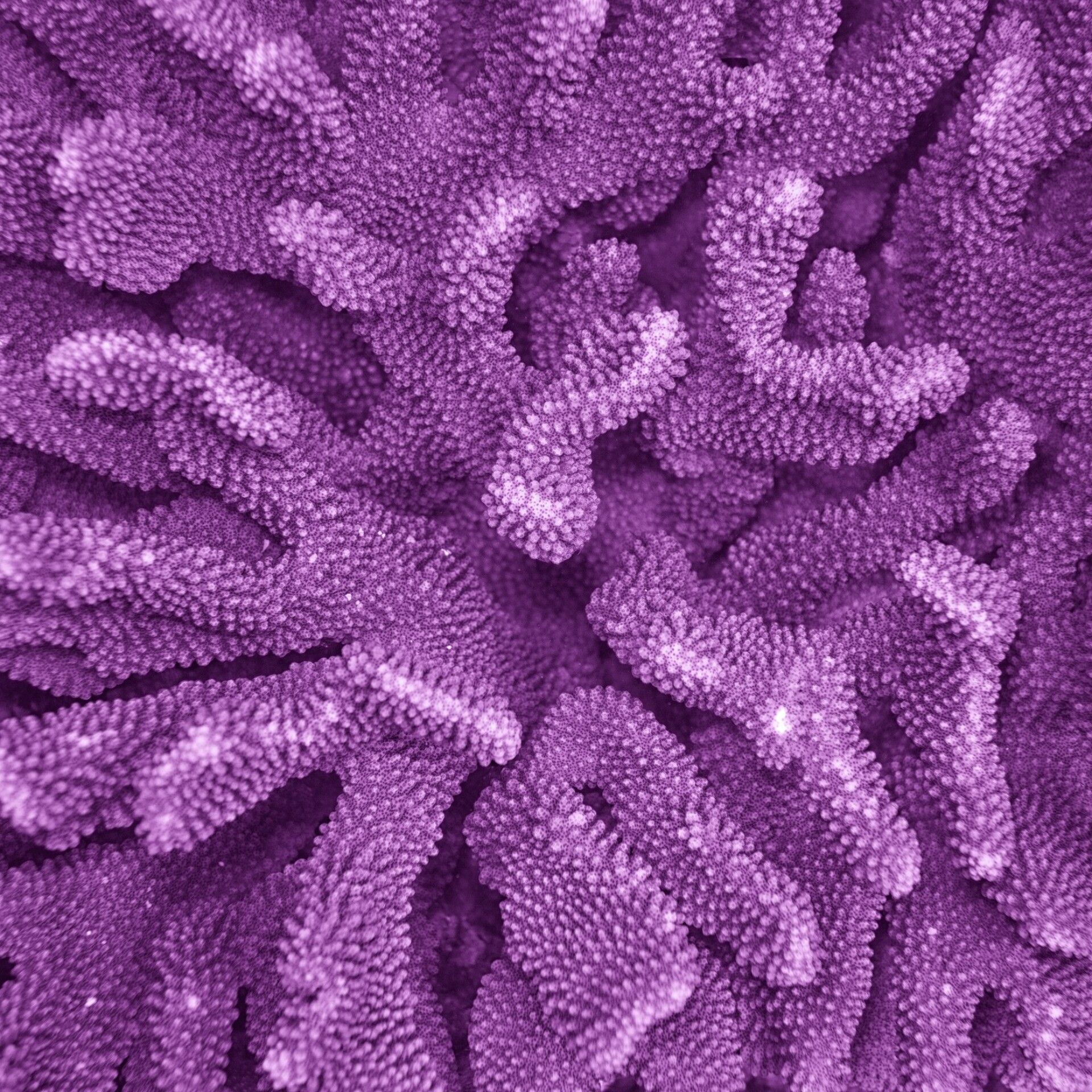
© iStock/tunart
Machine learning and artificial intelligence (AI) are already in use in many areas of application. This involves the training of models on the basis of numerous data points to then be able, for example, to detect defective components on the basis of camera images or predict the further development of disease progressions. These models are generally evaluated on the basis of their accuracy according to selected test data, i.e., the frequency with which the predictions of the model correspond with the actual solution.
In critical areas of application, however, accuracy alone is not a sufficient performance indicator, as it does not allow any conclusions to be drawn regarding remaining cases of error in terms of how these are distributed, or whether the model is susceptible to systematic errors. This means, for example, that despite a very high accuracy, all the errors may only arise in a specific class, in which mix-ups are of particularly critical importance.
A tool that makes specific suggestions
for improvements
In-depth analyses of a model are complex and time-consuming, often require manual work, and the interpretation of the results requires expertise and experience in many different areas of machine learning. For this reason, the Fraunhofer Institute for Cognitive Systems IKS has developed Robuscope: a straightforward online tool for testing the robustness of an AI-based classification model. The user is able to access the experience of our researchers in the testing of AI models in just one mouse click. A wide range of established metrics highlight specific error characteristics and help to develop a better understanding of potential weaknesses in the model. The analysis report also includes specific suggestions for improvements to help the application experts develop more reliable models.
The researchers at Fraunhofer IKS firmly believe that every AI model should undergo extensive testing before entering practical use. To make this possible for all AI users, Robuscope has reduced the required input data to the absolute minimum. Access to the trained model or possible sensitive training or test data is not required. Instead, the user loads a file into the tool (https://robuscope.iks.fraunhofer.de) with the predictions of the model to be tested – a probability distribution across the individual classes – as well as the expected result (ground truth). Within a few seconds, a detailed analysis report is generated directly in the browser on the basis of the data, providing information about the quality of the predictions of the model to be used. This report can, of course, also be downloaded for subsequent analysis.
Stable forecasts required
In detail, the analysis consists of various partial factors. It is first investigated whether there are classes that are mixed up particularly frequently and how confident the model is in doing its work. This allows for, in particular, the detection of systematic errors. The final interpretation is highly dependent on the case of use, however. If most of the mix-ups concerns classes that functionally lead to similar behavior, such as different defect patterns during the quality control, both of which lead to rejection of the workpiece, this is less critical than if a specific defect type were to be frequently mixed up with a defect-free workpiece. Clusters of error cases are often an indicator of a lack of training data – especially for distinguishing between the classes concerned.
Take the opportunity to see for yourself: Give our analysis tool Robuscope a try, and get to know your AI models better!
If you have any questions about Robuscope, please contact Karsten Roscher: karsten.roscher@iks.fraunhofer.de
Following from this, the stability of the predictions as well as the calibration of the output probabilities are analyzed. Stable and thus robust models are characterized by the fact that a sufficiently large distance exists between the correct result and the other options, so that minor changes do not immediately lead to a different result. Calibration, on the other hand, describes how well the predicted probabilities (interpreted as confidences) match with reality. In the case of inputs for which the model is very certain, it should also be correct at all times. Only in this way can the confidence be used as an additional measure of the reliability of the model – to inform the user in the case of low confidence or to use alternative algorithms instead of the model, for example.
The subsequent analysis block makes use of this very idea. In this context, various ways of harnessing the probability distribution of the model predictions are evaluated in order to reduce the errors during the use of the model. However, this is usually at the expense of performance. The identification of a favorable operating point by choosing an appropriate threshold value – i.e., the point from which the model is to be classified as “uncertain” – can be enabled by comparing residual errors and the remaining accuracy as well as other uncertainty-specific metrics.
In addition to valid test data from the area of application of the model (“in distribution”), the user is also able to provide outputs of the model for applications on unknown (“out-of-distribution”) or heavily distorted (“corrupted”) data, if these are available. In the report, such types of data are then considered separately to evaluate the behavior of the model on these very inputs, and to thereby obtain a comprehensive picture of its practicality.
This project was funded by the Bavarian State Ministry of Economic Affairs, Regional Development and Energy as part of the project thematic support for the Development of the Institute for Cognitive Systems.