Read next
DEEP Series, Part 3
Subtasks enable solving complex inspection problems
DEEP is a machine learning toolchain by Fraunhofer IKS for the reliable AI-assisted automation of quality inspection systems. For this purpose, DEEP automates various specific Fraunhofer IKS technologies. This part of the series of blog posts focuses on the specific technological contents of modular concept learning.
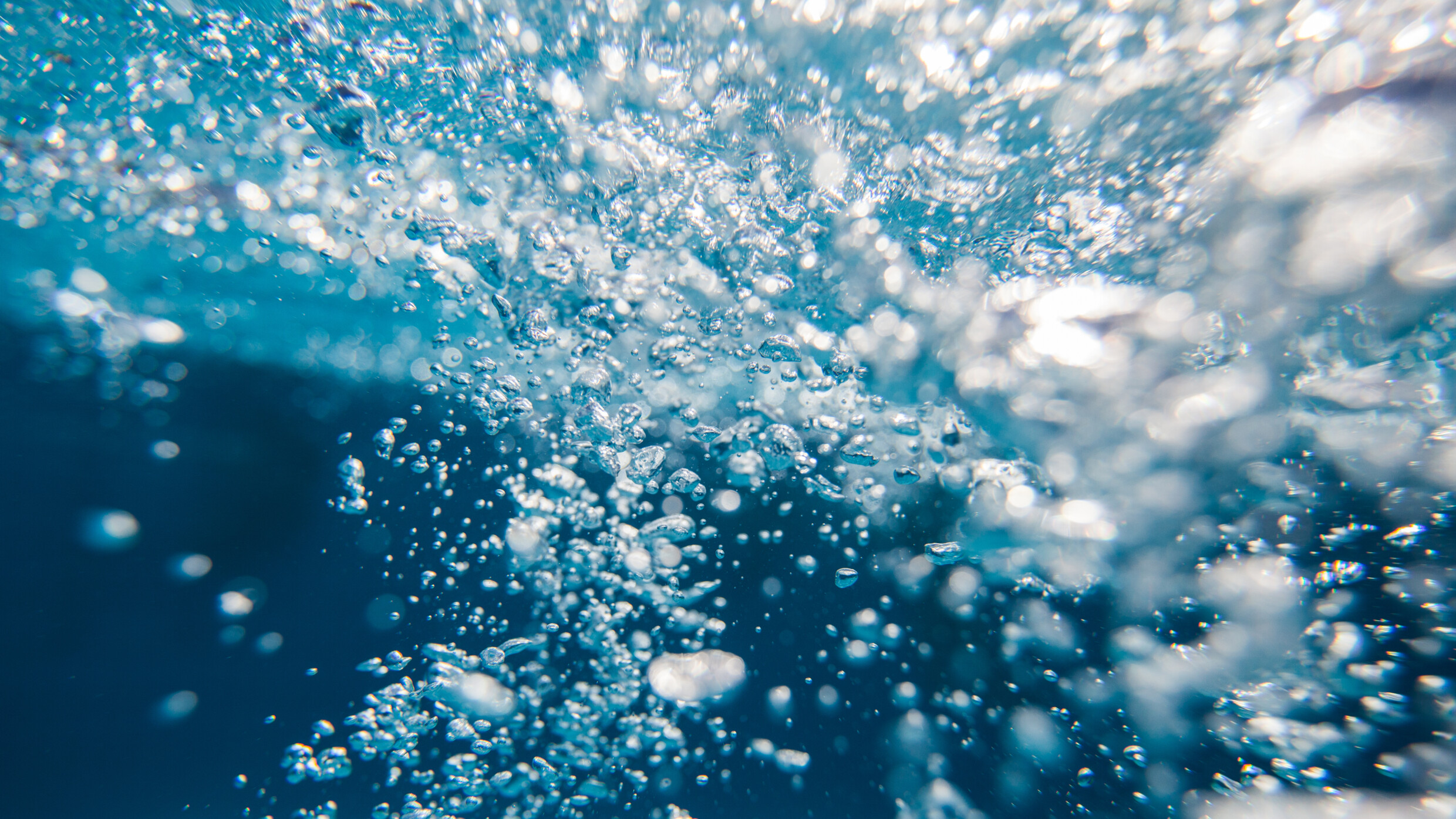
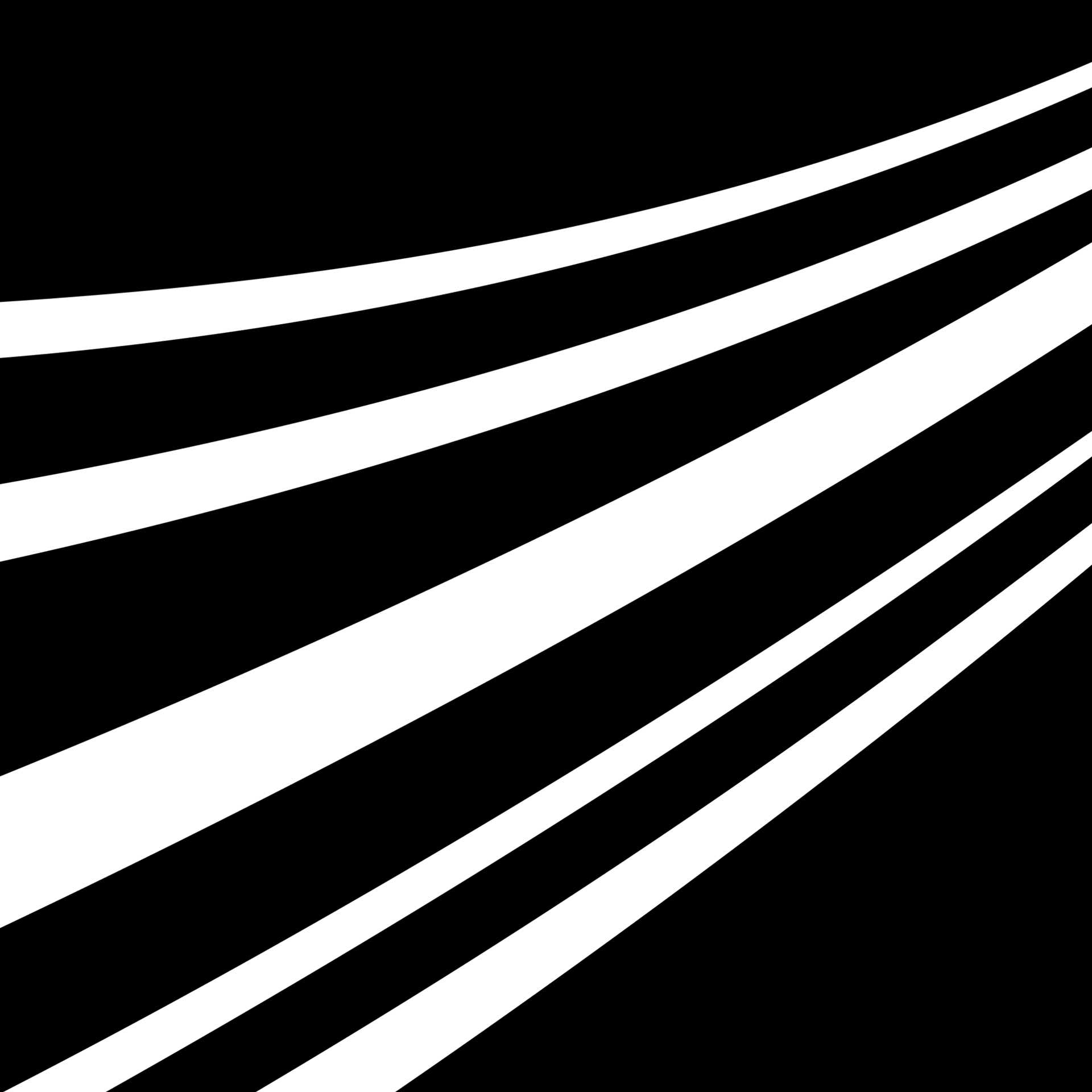
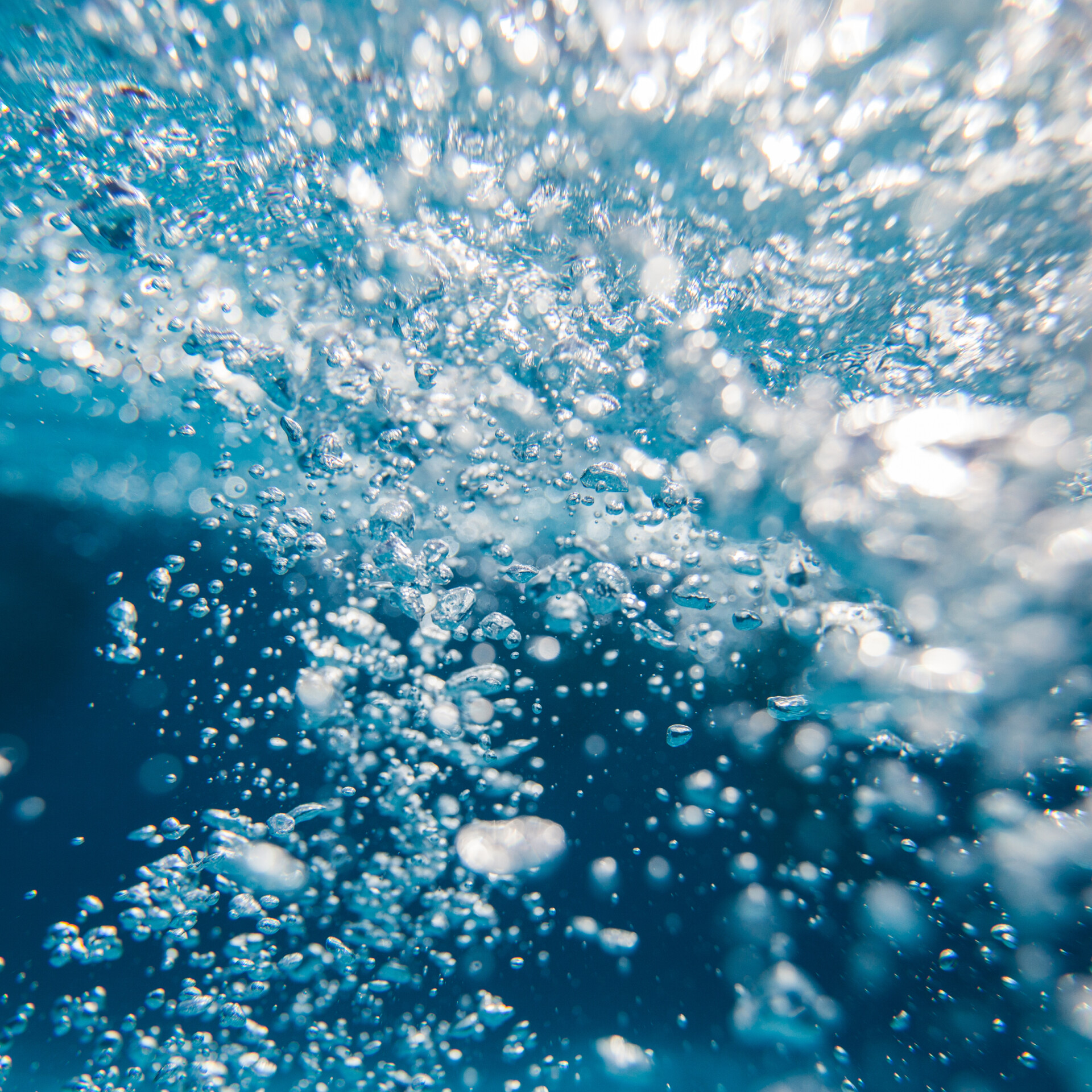
© iStock/maksym
Production processes frequently change, whether due to new product requirements (such as size or material) or the production of new variants in small batches. When machine learning (ML) is used for the inspection of these products, it usually requires retraining the artificial intelligence (AI) model, which is costly and time-consuming.
This is where modular concept learning comes in.
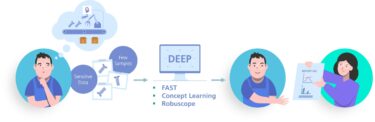
© Fraunhofer IKS
Fig. 1: The technologies FAST, Robuscope and Concept Learning are important components of DEEP (Data Efficient Evaluation Platform), the machine learning toolchain for reliable AI-assisted automation of quality inspection systems. Just like DEEP as a whole, they are the result of research at Fraunhofer IKS.
Models can be reused even when the conditions change
Modular concept learning enables the development of explainable and adaptable AI models for image recognition. Complex tasks of image understanding are divided into subtasks, so-called conceptual models, which allow relevant visual concepts, e.g. recurring patterns or structures in the image, to be recognised and combined in a transparent decision-making process. The modular structure of concept learning as implemented by the Fraunhofer Institute for Cognitive Systems IKS offers two significant advantages: It facilitates reusing the models under changed conditions and makes them both easier to explain and better adaptable to new requirements.
In contrast to conventional, comprehensively trained ML models, which consider image recognition as a single complex task, concept learning is structured more like human perception. To make decisions, humans look for specific features and concepts in images, such as the shape of the ears when distinguishing between dogs and cats, or the colour of a traffic sign to quickly recognise which traffic laws apply. Modular concept learning also breaks down image understanding into sub-areas, such as recognising shapes, textures and colours, and combines the recognised concepts into an overall statement. The association between the visual concepts and the actual task – in this case, for example, a quality assessment intended for automation by the AI model – can be based on manually defined rules (for example, "red colour and octagonal shape is equivalent to a stop sign") or can be achieved by training with existing data.
The modular design of the model improves interpretability, flexibility and adaptability by making the decisions transparent by means of the identified concepts and their relationships. The components can be adapted to the specific requirements of the application, also by a combination of conventional algorithms and AI models. Instead of retraining the entire model, this approach allows the targeted adaptation of individual parts to respond to changes in the tasks or in the dataset. This significantly reduces the effort required for training. In addition, the modularity facilitates a detailed error analysis by making it possible to assign errors to a specific conceptual model and to correct them with pinpoint accuracy. This supports the development of highly targeted measures, such as the collection of additional data on a specific concept.
Modular concept learning as part of the DEEP tool chain
The modular concept learning approach by Fraunhofer IKS maintains knowledge across changes through reusable models that recognise essential and frequently occurring concepts. The aim is to learn these visual concepts in the form of simple and reusable components and to use them sensibly in combination with existing domain knowledge. Modular conceptual models are based on this valuable combination of the conceptual components and expert knowledge. Modular concept learning as part of the DEEP tool chain offers a platform for the provision of the components already developed by Fraunhofer IKS, which can be combined with little effort to solve new inspection problems. In addition, new components can be developed quickly and easily using existing data without the need for prior AI knowledge.
Want to find out more?
If you have any questions about DEEP or the automation of quality inspection in industrial environments, please do not hesitate to contact business.development@iks.fraunhofer.de
The conceptual components remain usable even in the event of changes to the shape of the product, for example. This is helpful because the learned defects can also occur in other parts that are manufactured using similar processes. In addition, it is possible to add new concepts and easily adapt the tasks of the overall model without changing the existing conceptual models. The quality of the components can be assessed on the basis of the detected defects. Since some defects may be acceptable for certain products while others are unacceptable, domain knowledge plays a critical role in selecting relevant defects and linking quality assessment approaches. Other decisions besides conventional quality inspection can also be made automatically based on the recognised concepts. Depending on the production process, for example, adjustments can be made directly to minimise sources of error. This not only ensures quality, but also increases efficiency in the production process.
Application example: Thermoplastic
injection-moulded parts
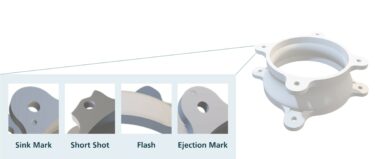
© Fraunhofer IKS
Fig. 2: Modular concepts for production errors using the example of a fan adapter in thermoplastic injection moulding
A suitable example for application of the modular approach by Fraunhofer IKS is the quality inspection of thermoplastic injection-moulded parts, such as electronic housings, medical syringes or fasteners used in the construction industry. During the injection moulding process, various surface defects can occur and can result in critical quality problems. A few examples of these defects are shown in simplified form in Fig. 2: A common phenomenon is overmoulded parts, i.e. parts with flashes or burrs. These occur when excess material adheres to the edges of the moulded parts and results in undesired raised structures. They can impair both the functionality and the aesthetics of the component. Another problem is incompletely filled parts (= short shot). They occur when the mould is not completely filled with plastic, resulting in gaps or areas where material is missing. This may be due to insufficient pressure or incorrect temperature conditions during injection. Other defects are ejection marks, which occur when the ejector mechanisms leave traces on the mould when the component is removed, and sink marks, which occur when the surface of the component collapses or sinks during cooling. These errors sometimes affect the structural integrity of the part and should be avoided through process improvements.
Modular concept learning by Fraunhofer IKS can learn and recognise either these defects directly or the defect characteristics as defined concepts. For quality inspection, this means we can precisely control which defects or types of defects on a part will be considered still acceptable and which ones will result in a failed quality assessment. Besides quality inspection, direct intervention in the production process can also be useful for thermoplastic injection-moulded parts. Automatic reduction of the injection speed, e.g. when detecting an overmoulded part, can prevent this defect in the subsequently produced parts and thus increase the efficiency of the production process. The conceptual models of modular concept learning provide the required defect information for this.
In summary, the modular concept learning approach by Fraunhofer IKS shows how reusable models can be used effectively, even when the conditions change, to increase the quality and efficiency of production. By breaking down complex tasks of image understanding into comprehensible conceptual components, this approach enables flexible adaptation to new requirements and a precise quality assessment. The combination of expert knowledge and modular concepts enables defects to be identified and corrected quickly, which not only ensures product quality, but also optimises the production processes. Modular concept learning therefore represents a pioneering solution to modern inspection problems that helps businesses to successfully adapt to changing market conditions.
In the fourth and final part of the blog post series, we will consider the "big picture" of the DEEP tool chain using the process steps of DEEP.
This work was funded by the Bavarian Ministry for Economic Affairs, Regional Development and Energy as part of a project to support the thematic development of the Institute for Cognitive Systems.