Read next
Production
Showcasing Cutting-Edge Automation at Fraunhofer IKS
To investigate the future of automation and possible transition paths towards it, Fraunhofer IKS is working on extending automation systems for novel applications. Read on to understand how these prototypes have taken shape and what insights they provide.
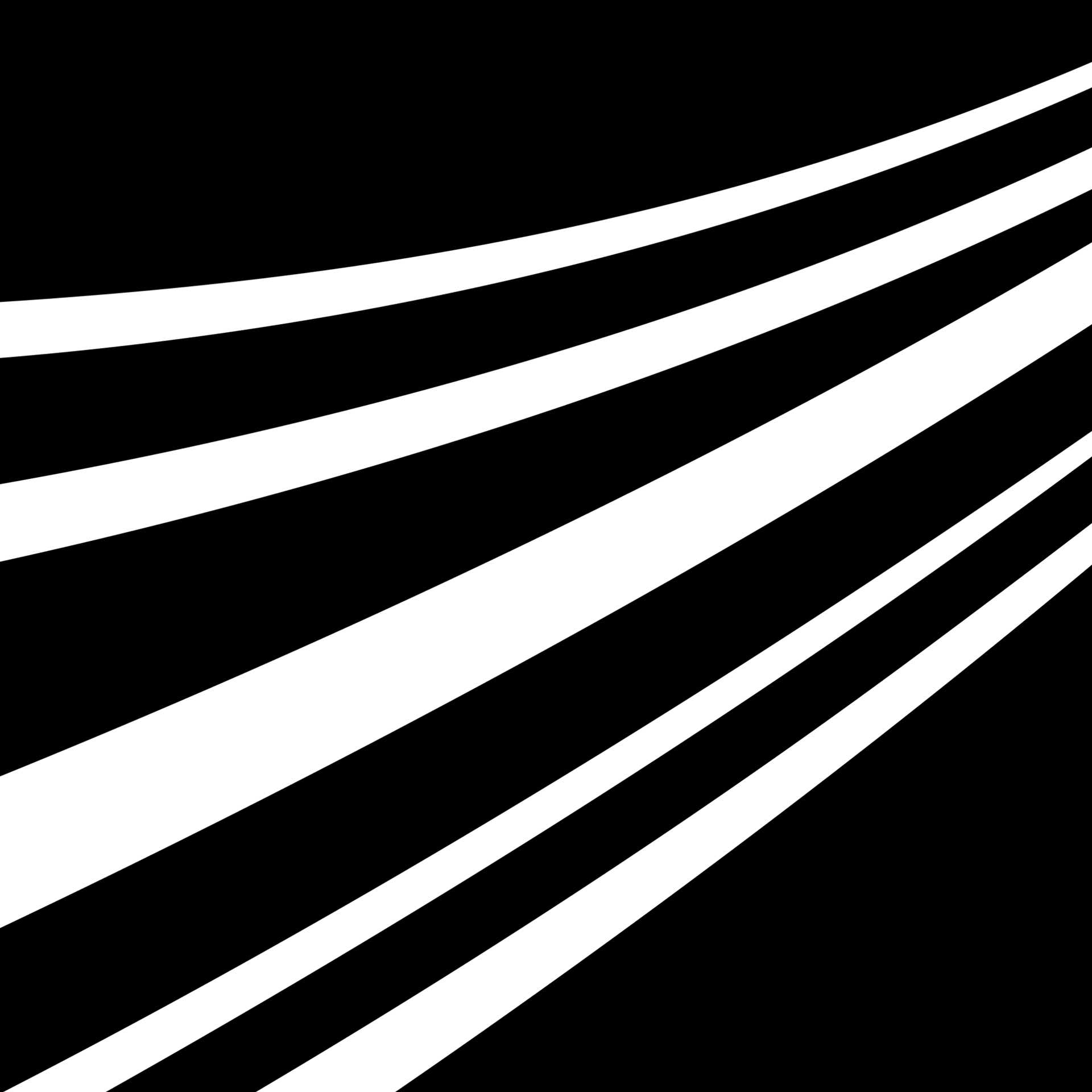
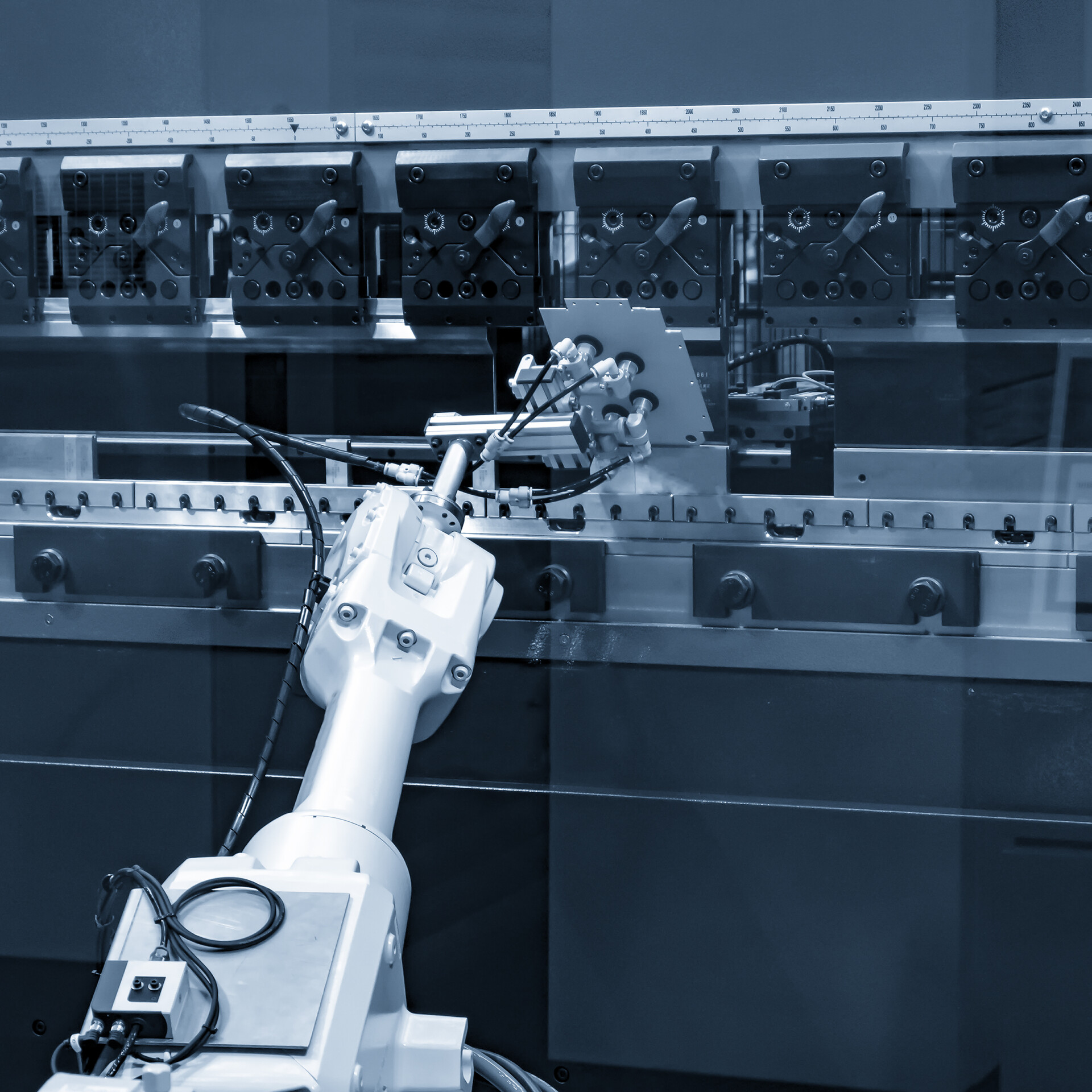
© iStock/Phuchit
As manufacturing businesses strive to remain competitive, they drive the demand for new industrial automation solutions. In particular, there is growing interest in approaches that increase the degree of flexibility within the production line, enabling the manufacturing of highly customized products in small batches. At the same time, companies need machines with improved capabilities to safely and efficiently collaborate with workers on the shop floor, to leverage human skills and maintain high quality in times of skilled worker shortage. To investigate how these requirements translate into practice, researchers at Fraunhofer IKS have built two systems that advance the state of the art on the shop floor.
Flexible Production
The first practical scenario was chosen as an example of lot size one production, where high degrees of variability and flexibility are required during manufacturing. It concerns a modular cap factory (cf. Figure 1), where abstract products consisting of a magazine, one or more rings, and a cap are assembled. An independent production station with the required tooling is responsible for each assembly step, and the partially assembled product is transported from one station to the next by an autonomous mobile robot (AMR). This modular setup increases the possibility of customization (e.g., assembling a product without a ring or a cap, or changing the color of these components) and allows for the use of different stations in parallel (i.e., for processing multiple orders at once).
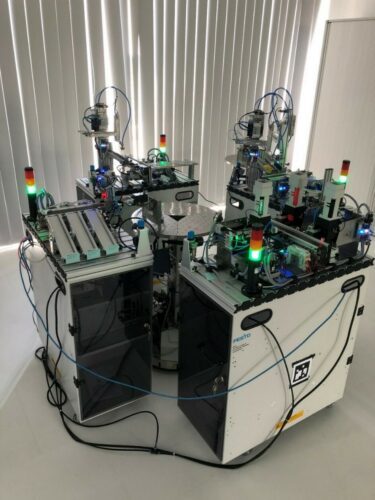
Figure 1: Overview of the cap factory, where independent stations flexibly assemble a product with the assistance of an autonomous mobile robot.
Within this context, a framework that supports the deployment of AI techniques to the shop floor is employed, incorporating the following techniques:
- Digital twins: Monitoring and documenting the status of each production station, including the current availability of components for assembly, requires machine-readable fact sheets of the stations – so-called digital twins. These models are structured using Asset Administration Shells, which are a fast-developing representational standard in the automation domain.
- Contract-based deployment: The framework relies on a service-oriented architecture to ensure its modules – which are packaged as services – can be flexibly deployed. Each service has an associated service contract, which documents the service’s data and resource requirements. By having these formalized contracts, it becomes possible to check ahead of deployment whether the desired host can fulfil the requirements for successfully running the services. This mechanism is also useful when it becomes necessary to dynamically redeploy services in case a host suddenly becomes unavailable.
- AI-based analysis: The framework simplifies the deployment of AI to perform various tasks around the shop floor. For instance, visual quality analysis for products can be accomplished by training and deploying a machine learning model using images captured by cameras on the shop floor. Of course, aside from visual quality inspection, there are many other manufacturing use cases that benefit from the use of AI, including predictive maintenance, inventory optimization, etc.
- Automated model retraining: Over time, AI models employed for analysis may become inaccurate. For example, if using a model for quality control, new product customizations that the model was not trained for may be eventually introduced, which would lead to unreliable analysis results. To address this, the framework can automatically detect when a model’s confidence is low, triggering retraining with more recently collected data.
To support the framework, a dashboard (cf. Figure 2) was employed to provide real-time insights into the state of services, devices, and processes in the production line, as well as overlook the analysis steps for deployed AI models. This also allows production managers to quickly adjust and react to changes from a central place.
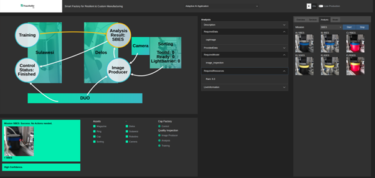
Figure 2. Dashboard for framework, showing production line and service states, as well as information on active AI models.
Human-Machine Interaction
The second scenario was chosen as an example of human-centric production, where the goal is not to replace human workers, but instead to assist them during key manufacturing steps in a new production paradigm. It concerns the collaboration between a human worker and a robot – more specifically, a lightweight robot arm – on the shop floor (cf. Figure 3). The design of these so-called cobots enables humans and robots to share the same workspace.
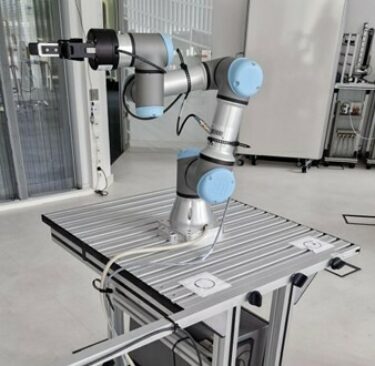
Figure 3: Lightweight cobot for human-machine collaboration in manufacturing.
In the scenario, the arm independently accomplishes a machine tending task, whereas human workers are required at other stages. This means that robots and people must negotiate space on the shop floor, and safety measures – restricting the maximum speed of the robot and triggering safe stops – are required to avoid injuries from collision and entrapment accidents. At the same time, however, these safety measures must not be triggered incessantly, so that reasonable cycle times and throughput can be achieved. To realize this adaptive behavior, a modular safety concept was developed with the help of model-based safety engineering and several key results from our research:
Do you have any questions or would you like to learn more about this topic? Please contact our expert Alexandre Sawczuk da Silva.
- Model-based safety engineering: System safety is argued using an assurance case, which is standard practice in many safety-critical domains such as automotive, railway, or aviation. The assurance case is built in reference to a formalized model of the admissible environment of the system – its so-called Operational Design Domain (ODD).
- Safety assurance for sensing: The reactivity of the system is partially achieved using AI-based perception, which outputs rich information such as the locations of humans and classifications according to their role (i.e., trained or untrained). Having a clearly defined ODD supports the argument for using AI functions in a safety-critical application.
- Human behavior models: The assurance case relies on assumptions about the behavior of the human workers when they are in proximity of the robot. Explicitly modeling these assumptions, as well as expected ways how the workers might deviate from the “correct” behavior – allows to also react adaptively to how humans are behaving.
- Safety concept switching: All these models combined allow for switching between different modes of operation at run-time: Based on the observed data about the human and the environment, the system determines the safety concept which is in effect and derives the appropriate mode of operation.
A simulator was developed to show this setup in action. The robot tends to a machine, while the workspace is monitored for human workers. As humans with different roles and behaviors enter the robot’s workspace, its mode of operation changes accordingly.
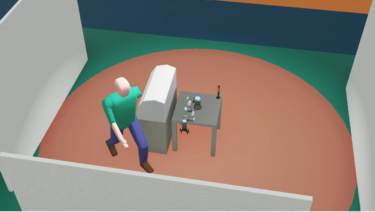
Figure 4: Screenshot of simulation where an untrained worker approaches the robot arm. In this case, a safe stop is triggered.
As shown in Figure 4, if an untrained worker (wearing green) approaches the robot, a safe stop is triggered to avoid collision and entrapment accidents. The safe distance (red circle on the ground) is calculated using a physics-based model, assuring that this distance is sufficient to stop the robot in time before a collision can occur.
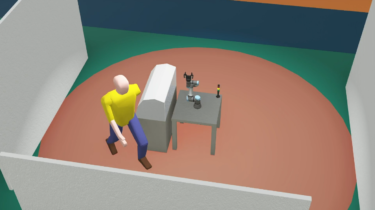
Figure 5: Screenshot of a simulation where a trained worker approaches the robot arm. In this case, the robot continues operating but with a restricted maximum speed.
For trained workers, like the person shown in Figure 5 (wearing yellow), a safe stop does not have to be triggered based on the assumption that the human knows how to behave in proximity to the robot. Thus, restricting the maximum speed is sufficient to assure safety.
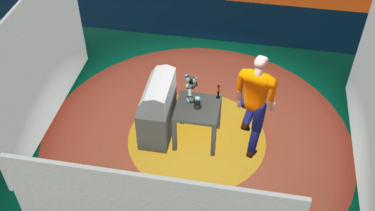
Figure 6: Screenshot of simulation where a trained worker approaches the robot arm at a fast speed. In this case, a safe stop is triggered for the inner safety distance.
However, if another trained worker (Figure 6, wearing orange) violates this assumption by moving too quickly, the robot switches to a third mode of operation. In this case, the robot moves at a restricted maximum speed within the safe distance. Then, once the worker approaches the robot closer than a second safety distance (yellow circle), a safe stop is triggered.
This demonstrator presents our vision of what is necessary for a rationalized future of manufacturing with human-machine collaboration that is both safe and efficient. Safety forms the boundary conditions in which productivity of the manufacturing process is optimized. The robot only implements those safety measures which are strictly necessary for the scenario and otherwise provides maximum utility.
In the Future
The two scenarios presented above are being actively worked on, with plans to further expand their scope. For instance, the use of multiple AMRs simultaneously can be investigated in the flexible production scenario, incorporating the ideas of parallelized order scheduling and fleet management into the existing system. Likewise, the human-machine interaction scenario can be extended to investigate a closer collaboration between humans and robots where the machine directly supports the worker with his or her task. Finally, both scenarios can be merged to address the challenges of handling a very complex shop floor.
Many of the state of the art ideas discussed here can be successfully transferred into industry and scaled up to operate in large industrial environments. To accomplish this, Fraunhofer IKS can help with the immigration towards new concepts, from incrementally extending existing systems to supporting the adoption and evaluation of completely new use cases. If you have any questions about how these techniques can benefit your business, please do not hesitate to contact us. Our experts are happy to provide guidance on how to leverage these innovative approaches to optimize your business.