Read next
Quantum Computing
Application is what drives the development of quantum technologies
As an emerging technology, quantum computing is expected to unleash disruptive potential in numerous industrial application areas. Those are for example in logistics, the pharmaceutical industry and the medical sector, or in all areas where machine learning (ML) is used. In practice, however, it is clear that numerous developments still have to be made in both, the field of quantum hardware as well as the associated software.

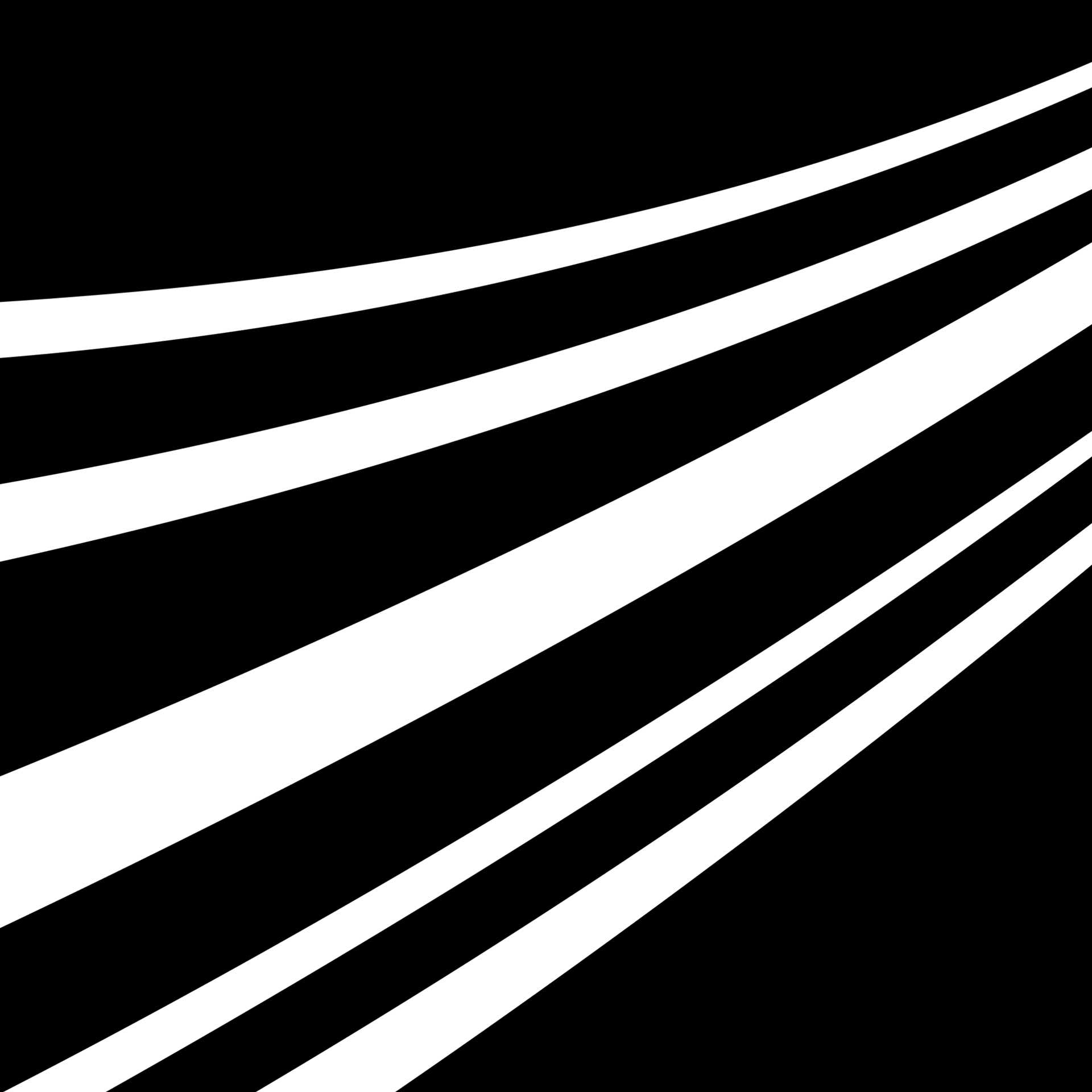
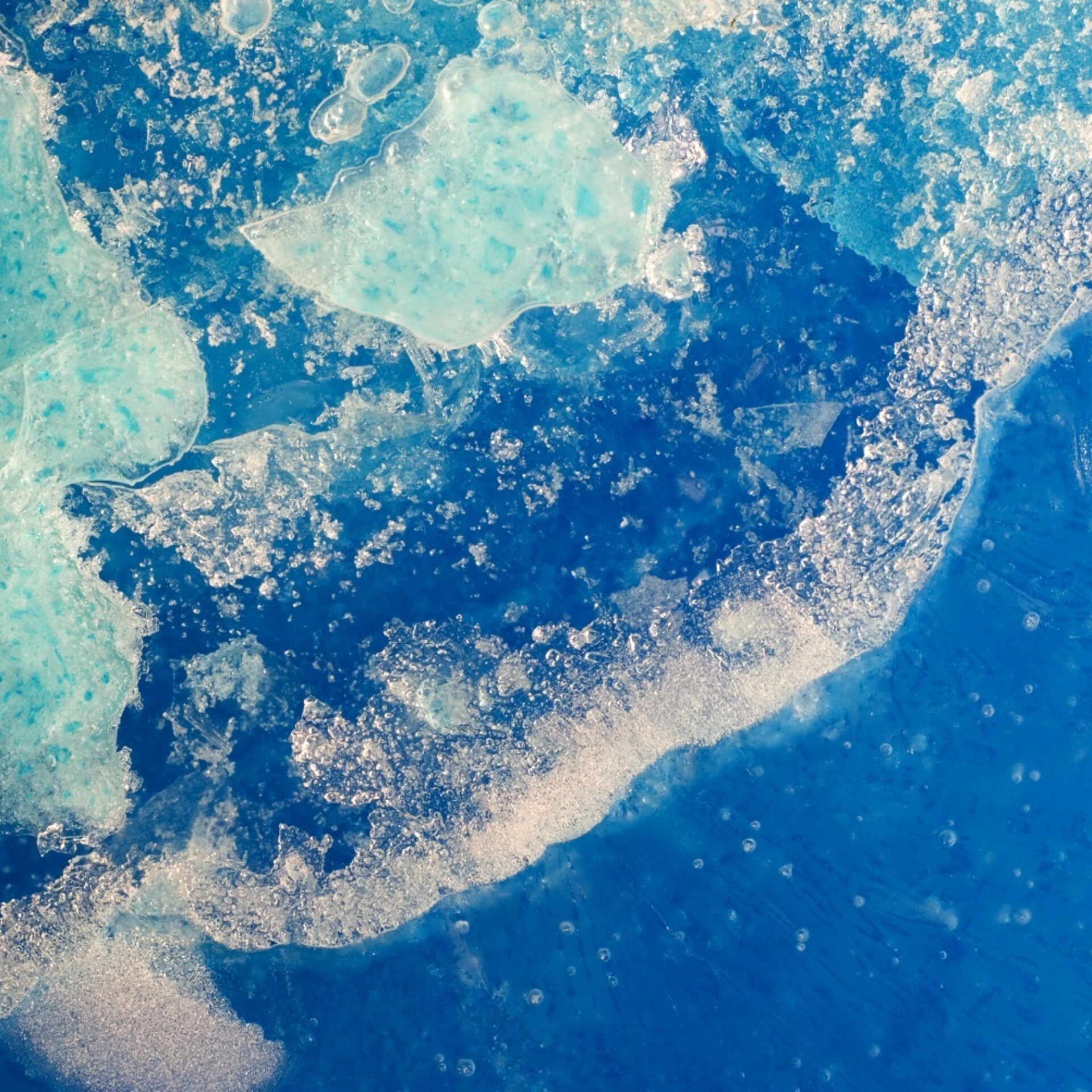
© iStock/yekorzh
Current quantum computers pursue different technology concepts and therefore also place different demands on their operation and use. Quantum computers based on superconductivity, for example, require very low temperatures just above absolute zero to operate, but on the other hand already achieve a relatively high number of qubits (IBM, for example, can already produce chips with 433 qubits).
However, these qubits are still quite prone to errors and, what’s more, not all of these qubits can interact directly with one another, which we refer to as connectivity. This connectivity between individual qubits however, is an essential requirement in order to benefit from the advantages of quantum computation. So, despite their apparent size, industrially relevant applications cannot be computed on these kinds of chips yet.
Ion traps versus superconducting qubits
Realizing other types of qubits involves technologies based on ion traps or cold atoms. In the case of ion traps, for example, charged atoms are confined in electromagnetic traps and can then be manipulated by lasers. The situation is similar for cold, neutral atoms, although in this case a magneto-optical trap constructed by several lasers is used. Quantum computers based on ion traps, unlike those based on superconducting qubits, can perform more accurate computations and have better connectivity. On the other hand, these kinds of quantum computers are slower in the computing steps, and it is much more difficult to realize a high number of qubits.
It can therefore be seen that the different technologies have very distinct advantages and disadvantages: With the former it is possible to realize more qubits than with the other, but it is more susceptible to noise; the latter is faster in performing the computational steps, but instead it needs more computations because of less connectivity. Ultimately, this results in a situation where it is currently unknown exactly which of these different quantum computers should be used for any specific application.
Which quantum technology for which application?
Applications do in fact have different requirements here. A quantum-classical convolutional neural network, as described in the “How quantum computing could be helpful for medical diagnostics” blog post, requires a lot of qubits and very fast execution of the individual computational steps. Optimization problems, on the other hand, which occur for example in logistics (“Solving difficult problems easily – but reliably!” blog post), use algorithms that demand high connectivity.
The situation is different again for the simulation of molecular properties, which quantum computers should be particularly well suited for. This was already stated by Richard Feynman in 1982 “because nature isn’t classical, dammit, and if you want to make a simulation of nature, you’d better make it quantum mechanical, and by golly it’s a wonderful problem, because it doesn’t look so easy.” (Richard Feynman, Simulating Physics with Computers, International Journal of Theoretical Physics 21, 467 (1982)). The accurate simulation of molecules could quite considerably speed up the discovery of new materials in battery development or new medicines. However, this is predominantly about a quantum computer delivering a precise result, rather than computations being performed particularly quickly.
For the simulation of an H2 molecule, my team at the Fraunhofer Institute for Cognitive Systems IKS and I explicitly compared the performance of quantum computers based on superconducting qubits and ion traps in a recently presented pre-publication titled “Benchmarking the variational quantum eigensolver using different quantum hardware”. It turns out that in this case quantum computers based on ion traps provide more accurate results, showing great promise for use in the chemical and pharmaceutical sectors.
For the time being, there is no getting around hybrid solutions
In all these discussed application scenarios, a close connection is needed between computations on a quantum computer and computations on classical computers in what are known as hybrid algorithms, at least in the near future. For example, the classical computer can be used to update parameters in the computation on the quantum computer. Or an ML algorithm can compute parts on a classical computer, and only offload those parts to a quantum computer that directly benefit from the speeding up of a computation by using a quantum computer.
However, the use of hybrid algorithms means that the interaction between classical computers and quantum computers should also be quite efficient, in order to not completely lose any possible speed advantages due to using a quantum computer. The close integration of quantum computers into high-performance computing infrastructures in data centers is necessary to guarantee this.
In fact, quantum computers are likely to only be used for parts of an overall algorithm – exactly where they play to their strengths. For the development of the overall algorithm, it is therefore necessary to ensure that the classical parts of the algorithm are aligned with the quantum algorithm parts, such as a necessary classical optimizer.
Due to the complex interaction of different technologies and software algorithms, it therefore makes sense to assess the performance of the overall algorithm – taking all components into account. More and more application-driven benchmarking procedures are now being developed for this purpose. In these, research is being conducted on specific application scenarios to determine which quantum algorithm is suitable together with which classical algorithms and using which quantum hardware technology.
Symposium
»Applications of quantum computing«
Munich Quantum Valley (MQV) and Fraunhofer IKS are organizing the symposium "Applications of quantum computing". Experts from industry as well as science and research will discuss the potentials of quantum computing from an application perspective.
When: Juli 10, 2023 from 2pm and July 11, 2023, 9 until 1 pm
Where: Institute for Advanced Study (IAS), Garching München
More info and registration:
https://indico.munich-quantum-...
Therefore, as promising as quantum technologies are for the application, all the software stack components and the different quantum hardware technologies still have to be tested for each individual case. However, many companies, especially larger ones, are investigating the potential of quantum computing for various applications together with universities and Fraunhofer institutes for this very reason.
In a symposium on “Applications of quantum computing”, co-organized by Munich Quantum Valley (MQV) and Fraunhofer IKS on July 10 and 11, companies and researchers are invited to demonstrate which applications are already feasible on quantum computers and which developments are needed on the hardware and software side in order to be able to benefit from the use of quantum computing in an industrial context.
This research project is part of the Munich Quantum Valley (MQV), which is supported by the Bavarian State Government with funds from the Hightech Agenda Plus.