Read next
MedTech
How quantum computing could be helpful for medical diagnostics
Quantum computing has the potential to train artificial intelligence in medical diagnostics more efficiently. This could make diagnoses more accurate even when there is little data available. In the future, medical professionals expect to see improvements in the screening, diagnosis and progress monitoring of brain tumors, for example.
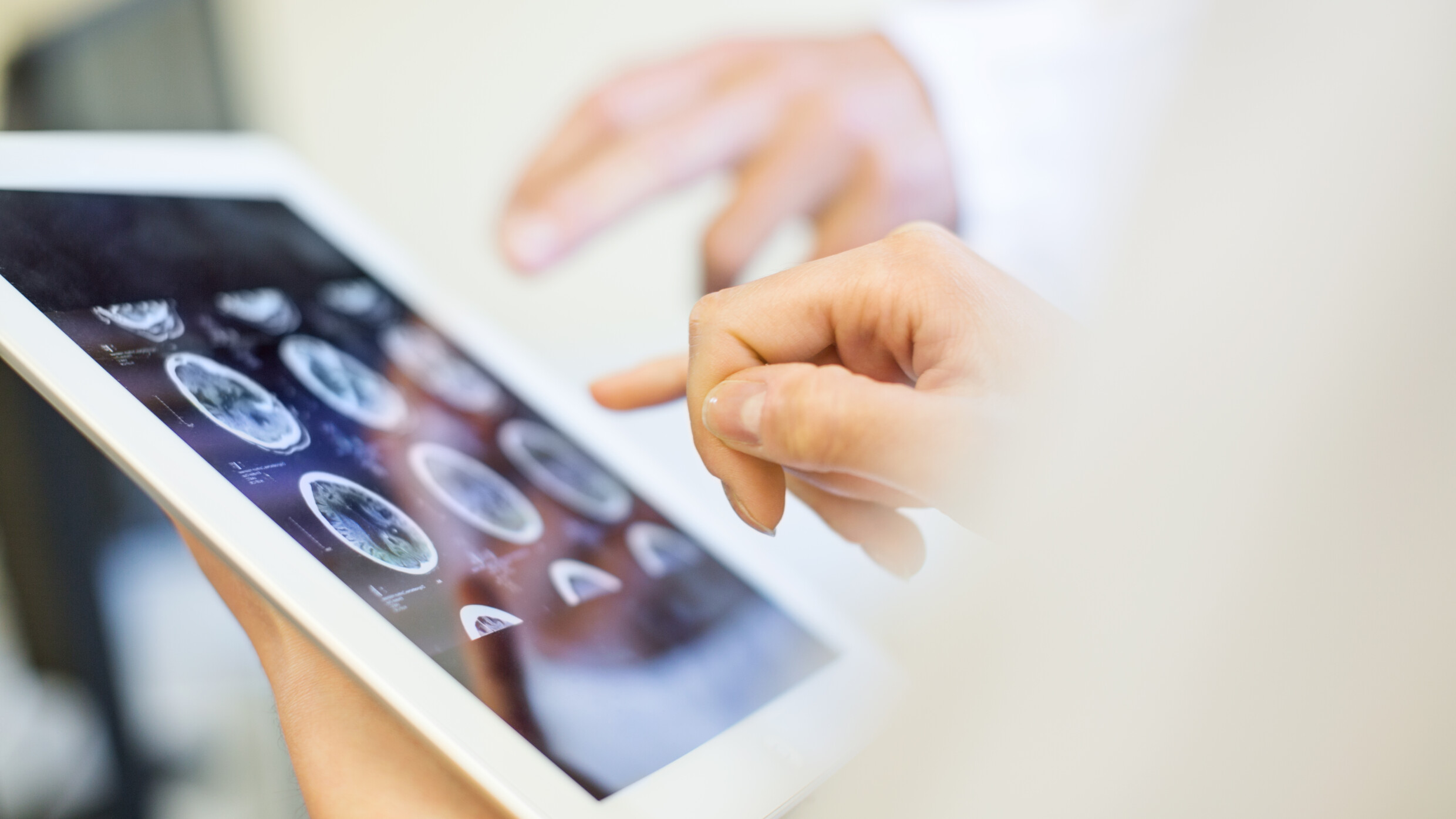
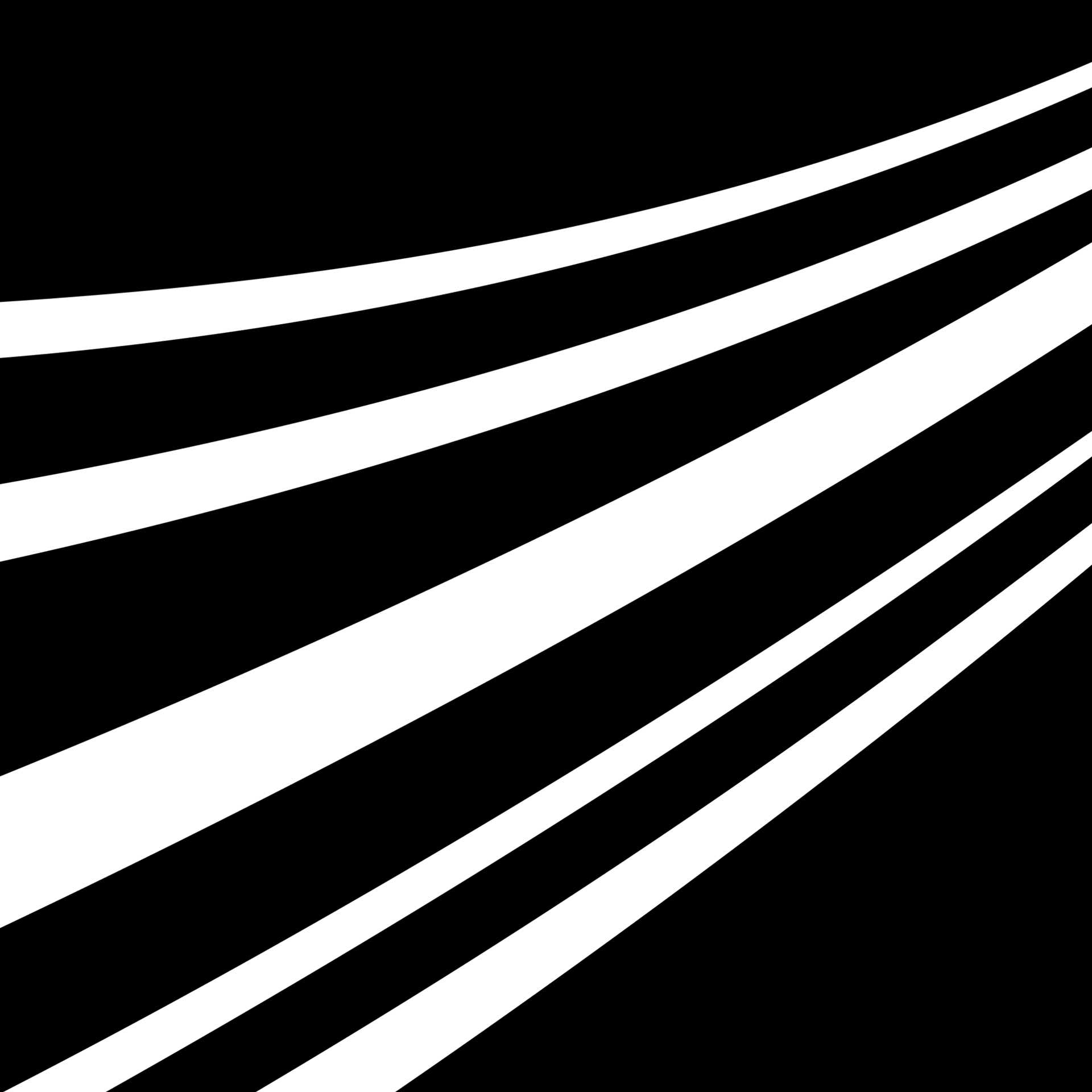
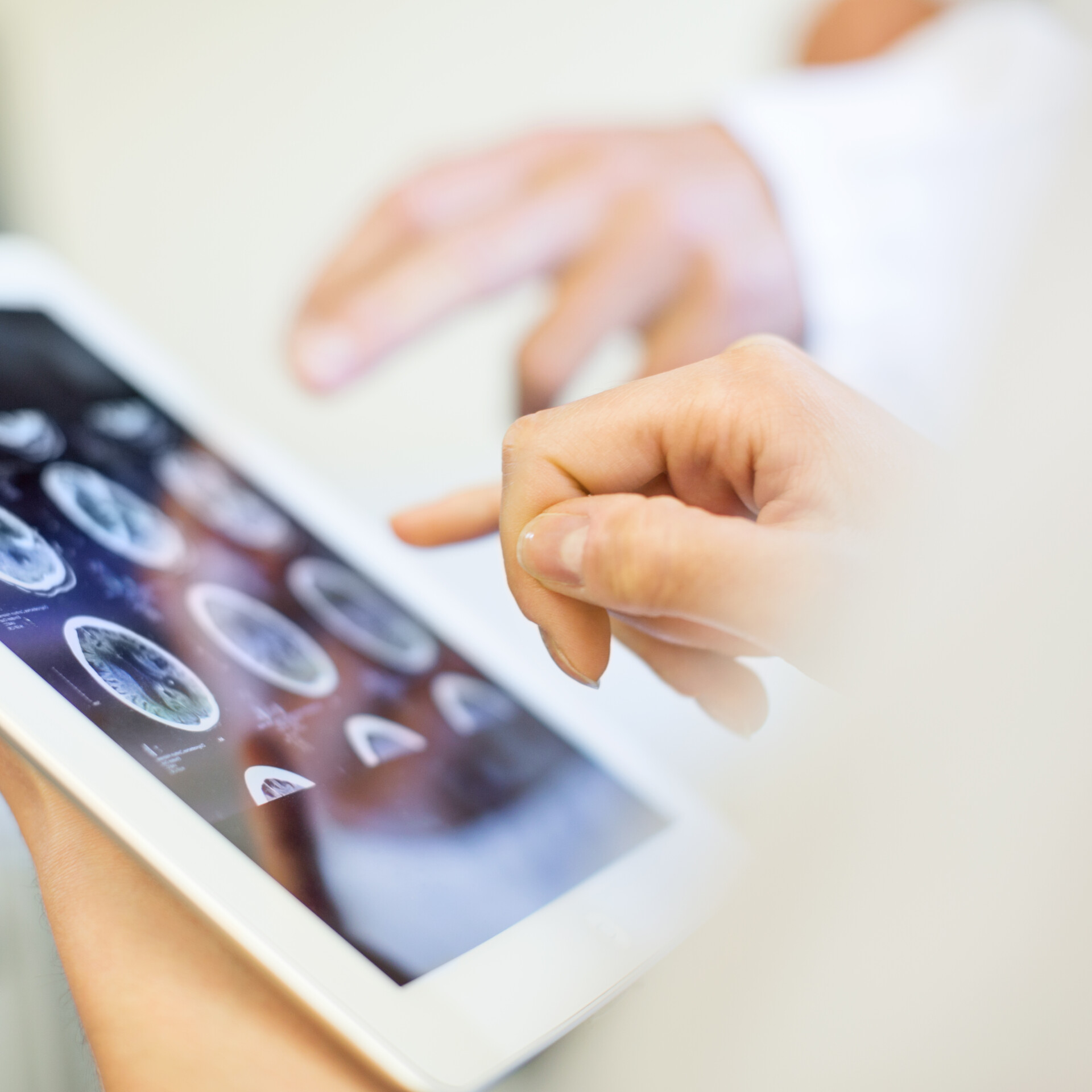
© iStock.com/alvarez
Artificial intelligence (AI) opens up completely new possibilities in the field of medical engineering with regard to care, diagnosis and treatment of diseases. It can be used to screen for tumor diseases, for example. In the field of medicine, however, it is necessary for an AI to deliver highly reliable results. This requires the existence of sufficient high-quality data to train the AI.
But in fact there are typically only limited quantities of data available, for example data from just 100 or 1000 patients. At the same time, the linking of a wide range of information to improve the treatment of diseases is making calculations ever more complex, which calls for increasingly powerful computers.
Quantum computing has the potential to provide support here, as quantum computers are expected to be particularly well suited to solving complex problems.
What is quantum computing?
Conventional computers express all data in bits, and each bit can have a value of either 0 or 1. A quantum computer, on the other hand, uses quantum bits (qubits), which can have any value in between. More accurately, at any one time a qubit is a superposition of 0 and 1. This means that data can be represented much more compactly in quantum computers than in conventional computers.
However, this is not the only reason why quantum computing is believed to be better than conventional computers in certain areas. In addition, qubits can interact with each other, which could cause different qubit states to become entangled and even interfere with each other. Ultimately, these three effects — superposition, entanglement and interference — allow quantum computers to perform certain calculations much more efficiently. This means they need far fewer iterations to achieve a quality similar to that of a conventional AI.
For now, this is only a theoretical expectation that has not yet been demonstrated in practical applications. That is because the quantum computers that are currently available are still relatively small, meaning they have relatively few qubits. For example, the IBM Quantum System One in Ehningen, to which Fraunhofer has exclusive access, currently has 27 qubits.
Today’s quantum computers are still very prone to errors, because the fragile qubit system needs to be almost perfectly screened from the outside world. That is the only way it can keep its quantum mechanical properties, which it needs in order to achieve the improved processing power.
Guaranteeing this is a major technical challenge. However, technical developments in the field of superconducting quantum computers are progressing rapidly, and IBM believes that systems with over 1000 qubits and improved error correction could be available within just a few years. That would put the ability to solve highly complex problems using AI within reach.
Quantum computers are versatile optimization problem-solvers
What types of problem are quantum computers expected to solve better than conventional computers? Some examples can be found in complex optimization problems, where many boundary conditions and a large amount of input data are at play. This applies, for example, to route optimization in logistics or to the optimized production of complex components such as semiconductor chips.
Quantum computers are also expected to be significantly better than conventional computers at simulating molecular biological or chemical systems. This is relevant in the development of new drugs or new types of sustainable battery, for example. They are also expected to make AI training much more efficient and therefore faster. This could, for example, be relevant in medical diagnostics based on imaging data (particularly MRI and CT imaging).
Hybrid algorithms offer a promising future
Because quantum computers are currently still very small and prone to errors, hybrid algorithms are proving particularly promising as a way to produce quantum advantages for industrial and medical applications. These involve a quantum computer interacting with a conventional computer so that only the parts of an algorithm that actually benefit from the more compact and efficient calculations made on a quantum computer will actually be calculated on it. It may already be possible to calculate these parts on the small, error-prone quantum computers that are available today. This means that the benefits of quantum computing for practical applications could be achieved in the medium term.
Quantum convolutional neural networks improve medical diagnoses
A highly promising example of such a hybrid algorithm is a quantum convolutional neural network (QCNN). Standard convolutional neural networks (CNNs) are often used in image recognition applications, such as facial recognition. CNNs only ever consider parts or sections of an image in what is known as the convolutional layer. It is this aspect that is of particular interest for existing quantum computers, given their relatively small size: Replacing a standard convolutional layer with a quantum convolutional layer requires just a few qubits and is to some extent independent of the errors made by the quantum computer hardware. In any case, the quantum convolutional layer is likely to be able to perform a significantly more efficient and compact calculation than a standard convolutional layer. It may require less training data and fewer iterations for a QCNN to achieve the same quality as a conventional CNN.
The Fraunhofer Institute for Cognitive Systems IKS is researching promising uses for QCNNs in medical diagnostics as part of the Bavarian Competence Center for Quantum Security and Data Science (BayQS). In this project, funded by the Bavarian Ministry of Economic Affairs, Fraunhofer IKS is working together with two other Fraunhofer institutes and with colleagues from the Neurosurgery Clinic and the Radiology Clinic at Ludwig-Maximilians-Universität München (LMU Munich), the Technical University of Munich and the Leibniz Supercomputing Center (LRZ). The potential of QCNNs (and of quantum computing-supported methods in general) in brain tumor screening is being researched using MRI and CT images. The improved training behavior of a QCNN allows more accurate AI-supported diagnoses and recommended actions to be developed even when there is little data available. This could enable not only a better and more reliable diagnosis, but also larger-scale screening and progress monitoring.
As well as QCNNs, this project is also researching quantum Bayesian neural networks (BCNNs), which will enable uncertainties to be determined explicitly by a neural network even when the data are complex, unlike with conventional deep neural networks. Thus, they can ultimately contribute to improved monitoring of the uncertainties of a medical diagnosis.