Read next
Artificial Intelligence
Why Safety Matters
Machine learning means a disruptive challenge for safety assurance. Without an acceptable safety assurance concept, many great ideas will not find their way to the market. It’s therefore no surprise that AI safety has gained much more attention over the past few months. A brief series of articles will take a closer look at the importance of safety for AI. The first article focuses on the basic understanding of what safety actually means. It highlights why it is so important to understand that safe AI has less to do with AI itself and much more to do with safety engineering.
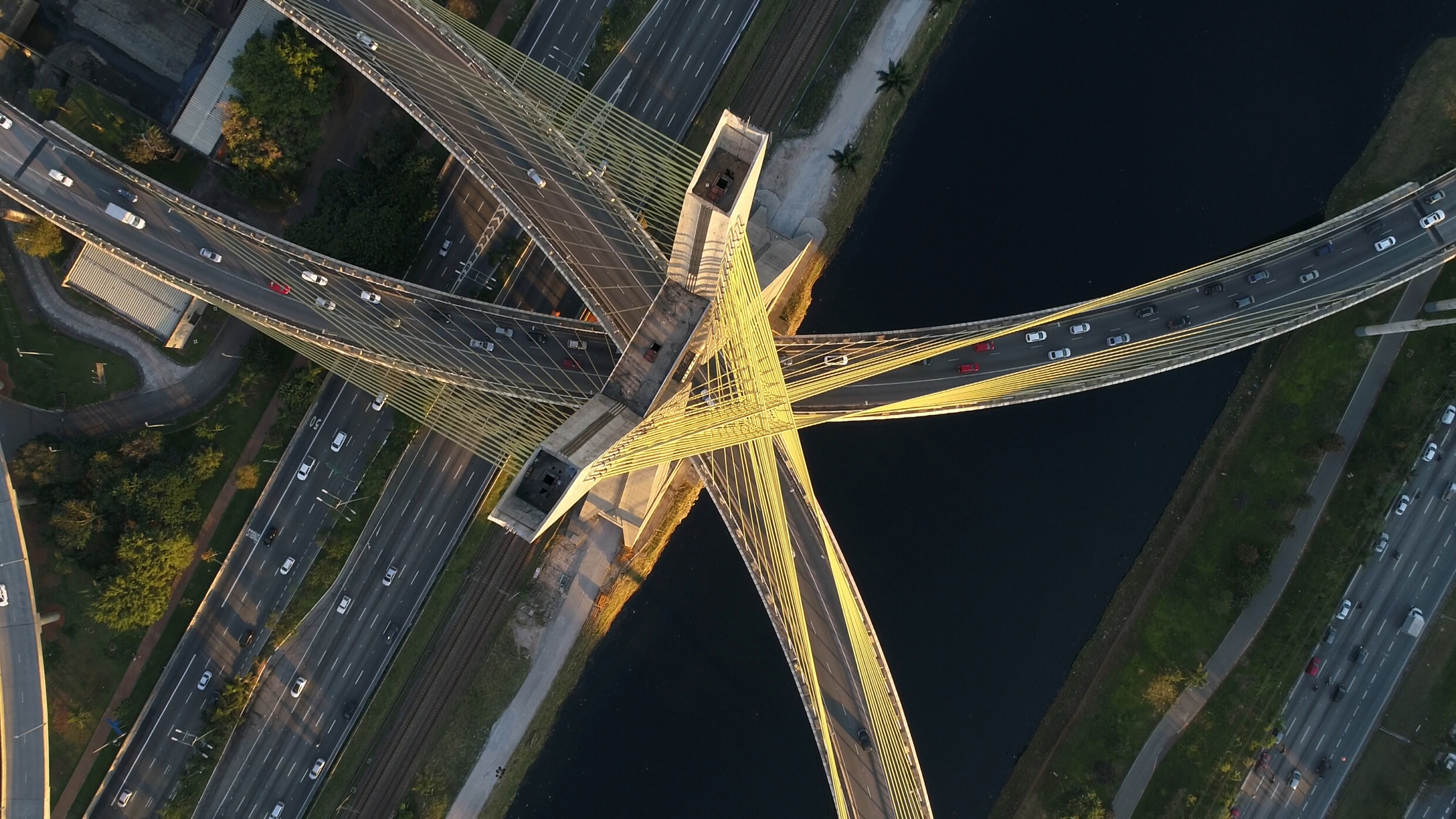
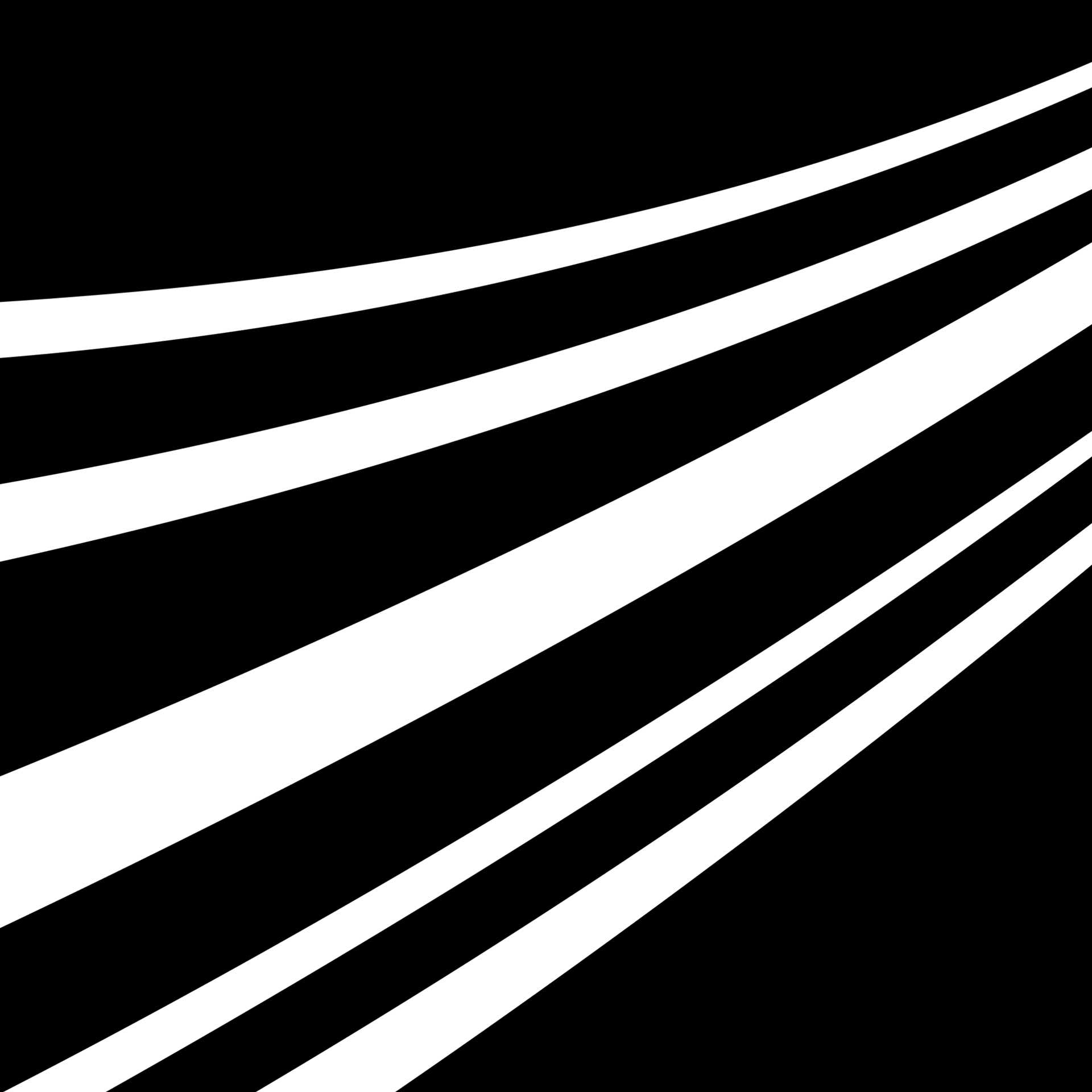
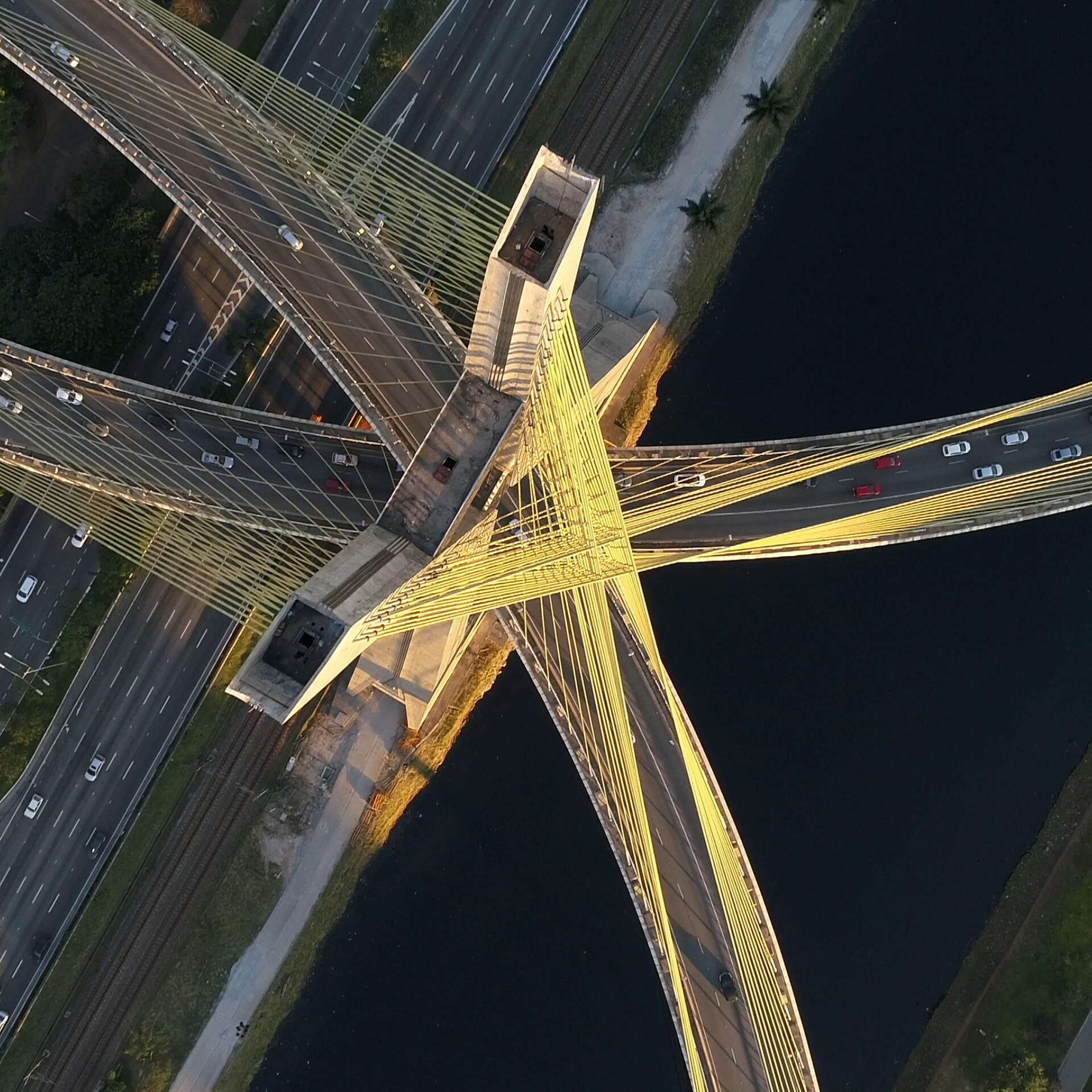
© iStock.com/gustavofrazao
For safety experts it is very clear that machine learning represents a disruptive challenge for safety assurance. To put it in a nutshell, a concept like safe machine learning is technically infeasible today. The only thing we can do is to try to assure safety at the system level; in other words assuring a cognitive system‘s safety despite the use of unsafe machine learning for safety-critical functions. For that reason, it is actually more a safety engineering challenge than an AI challenge.
As safe AI becomes more attractive, I often found myself in discussions in which I am confronted with an alarming misunderstanding of what safety actually means and sometimes even a complete lack of understanding of why safety should be an issue at all.
In spite of its crucial importance and the tremendous challenges for safety assurance, many people – including managers, politicians and AI experts – ask me why we make AI safety such a big issue in the first place. They are surprised at how safety can be an issue when they read that hi-tech companies are manufacturing autonomous vehicles that have been driven millions of miles.
It’s alarming to think that many people seem to accept the fact that a lot of setbacks already lead to personal injuries or fatalities. Risking human health and lives is never an acceptable price for technical progress. This is particularly true since users of AI and autonomous systems are hardly in a position to understand the risk they expose themselves to. Even more importantly, we need to consider the collateral risk. When it comes to autonomous vehicles for example, other traffic participants, particularly vulnerable road users such as bicyclists or pedestrians, are exposed to unacceptable risks as well – without having a choice.
On the other hand, I am also convinced that the answer is not to pin the blame on AI-based systems, or even prohibit them for use in safety-critical applications. When it comes to global competition, AI will find its way into safety-critical applications – one way or the other. Our primary goal should thus be to make sure that we first find a safe and yet economically profitable approach.
Understanding risk, understanding safety
To acquire a better understanding of what we are talking about, let us have a look at what safety actually means. Safety is nothing more than freedom from unacceptable risk. In turn, we measure risk as a combination of the probability of the occurrence of harm and the severity of that harm. The acceptable risk is a threshold implicitly defined by society. Standards or laws try to reflect this societal threshold and to derive explicit measures that ensure that the residual risk of systems that are brought into the market is lower than the acceptable risk.
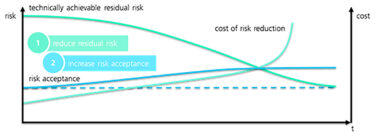
© Fraunhofer IKS
Figure 1: The relationship between residual risk, acceptable risk and cost
At a very high level, this leads to two basic ways of how one could try to achieve safety as shown in Figure 1. Normally, the goal should be to reduce the residual risk to an acceptable level. Regarding the cost however, it often starts to increase exponentially over the required risk reduction. It is therefore not surprising that trying to increase the accepted risk is a tempting alternative. Having a look at the rapidly increasing number of high-glossy marketing material titled something like “safety case”, combined with marketing campaigns, some companies seem to appear to be walking a dangerous tightrope between reasonable elucidation and intentional manipulation. Whatever we may think about that, it is part of the game.
In fact however, the risk acceptance benchmark is quite clear. Without going into detail, it is an intuitive and indisputable principle that introducing new technology must not increase risk. Self-driving cars must provably make traffic less dangerous than today – for every traffic participant. AI-based medical devices must lead to less harm than today’s available devices, and AI-based machines in production must not increase the risk for the workers using them. And if one really wants to open the discussion of balancing fatalities caused by cognitive systems against the number of potential lives that are saved, this will only be acceptable if (if at all) the number of provably saved lives outweighs the fatalities by several orders of magnitude.
Over the last months, it has become even more important to remind people of that benchmark. There are many good and useful initiatives dealing with “certifiable AI” initiated by the AI community. They consider many more things than just safety – including very important aspects such as freedom from discrimination, confidentiality etc. – but they also address safety. I fully support those initiatives. Nonetheless, it is very important that this trend does not lead to a dilution of safety benchmarks. However much I understand the technical challenge of guaranteeing the accuracy of machine learning algorithms, there is still a gap of many orders of magnitude between excellent quality from an AI-perspective, and what we can actually consider safe. Any attempt to gain the upper hand in interpreting what safe AI means by redefining safety is not helpful at all. Even if we accepted a flexible interpretation of the basic principle that new technology must not increase the risk for users, five-to-eight orders of magnitude worse than today cannot be an option. We must not define the benchmark for saving human lives based on technical feasibility.
It’s all about safety engineering
Increasing the risk acceptance level is obviously no reasonable way to achieve safety. And prohibiting machine learning for safety critical systems cannot be an option either. This is why we need new solutions for assuring the safety of AI. While AI research can help to some degree by making machine learning more robust and more explainable, the real challenge is in safety engineering.
Meanwhile, we should have realized that traditional safety assurance approaches are already struggling to make sure that conventional complex software systems are safe. Assuring functionality that is developed based on new, disruptive paradigms, with the same old conservative safety approaches, is just not feasible. In fact, we have to rethink the idea of safety and have the courage to use disruptively new ways of assuring safety. We need something like next-generation-safety, which we will discuss in more detail in the forthcoming articles.
Taking the bull by the horns
Safety assurance is based on a commonly accepted body of knowledge. The same is true for safety standards, which reflect the commonly accepted state-of-the-art. For machine learning however, there is nothing like commonly accepted assurance approaches. It normally takes decades to build up such a basis for safety assurance and safety standards. But we should obviously refrain from an option that reduces the safety benchmark. Neither is it an option to wait several years – which is particularly true since we will not gain the necessary knowledge without having systems in the field we could learn from.
In fact, we should take the bull by the horns. We should not try to define generic safe AI standards. For now, we simply do not have appropriate solutions and defining standards based on best guesses is not recommendable. Instead of general solutions, we should try to assure single systems with very specific solutions tailored to that specific system. This obviously requires significant effort since we have to work through a complete individual safety case, from high level safety goals, down to very specific evidences, which could require extensive effort to provide them.
Additionally, we have to establish a sound and systematic monitoring system to ensure that we detect incidents in the field and assure fast iteration cycles, thus improving the system, all comparable systems, and safety standards, while we build up the necessary body of knowledge step-by-step. While this means that the systems we can bring to the market will have many restrictions at the beginning, we can loosen them step-by-step over time. Safety standards for AI should therefore focus on providing the (legal) basis for individual safety cases, accompanied by an iterative improvement of products and standards over time.
Neither economic reasons nor an AI-driven redefinition of safety based on technical feasibility should drive the progress on safe AI. Instead, it is the task of safety engineers to rethink safety towards a next-generation safety assurance and to establish standards and corresponding frameworks that enable an early market introduction of individual products based on very individual safety cases. And to learn fast without endangering anyone.
This first article of this brief series is intended for the strategic management level, while the subsequent articles will address the topic at a more technical level. Next: Why safety assurance of AI/ML is a challenge.
Subscribe to the SAFE INTELLIGENCE newsletter
You want to be always up to date and receive regular information about Fraunhofer IKS? Then subscribe here to our newsletter (German): www.iks.fraunhofer.de/newsletter