Read next
Safetronic 2023
From Functional Safety to Holistic Safety - A Roadmap in a Nutshell
The safetronic® is the industry's premier conference for the holistic safety of road vehicles. In November 2022, experts from the automotive industry met again to discuss challenges, solutions and trends. Although the safety of automated vehicles and, in particular, the associated safety assurance of AI are currently in the public spotlight, the experts at the conference had a different perspective. For them, a well-known topic is experiencing a revival and is emerging as a new trend topic: model-based system safety engineering (MBSE). Having worked on model-based safety engineering for well over a decade now, I'm personally as intrigued by this trend as I am skeptical about whether it's going to take off this time. That's reason enough for me to take a closer look at this potential trend from my individual perspective in this article.
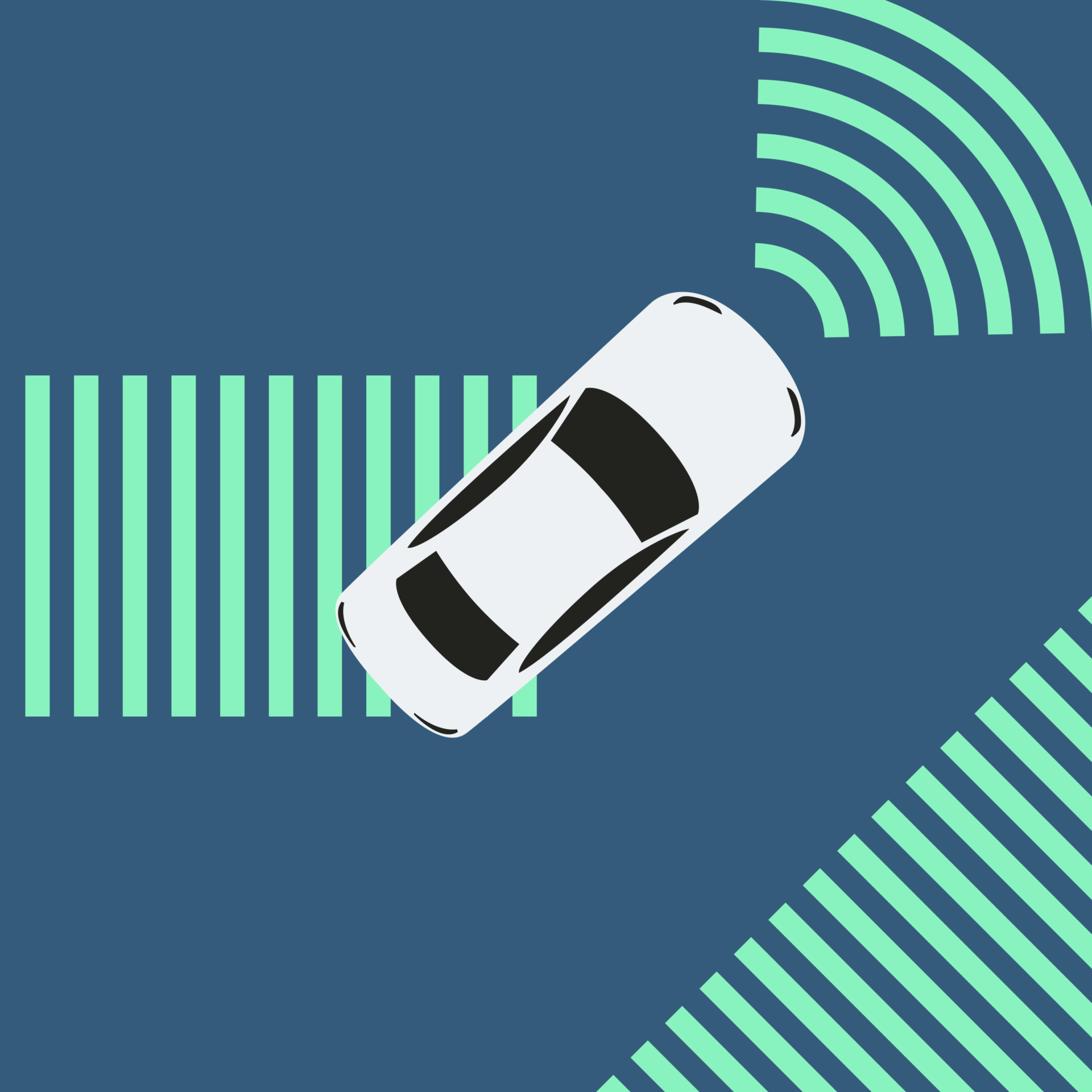
© Fraunhofer IKS
This trend towards model-based system safety engineering (MBSE) is somewhat surprising, since the subject was quite popular about a decade ago, but never made the breakthrough into broad practical application. So over the last years it’s been kind of in the shadows in the sense of: "We tried it, it didn't work". And indeed, the promises were great. But MBSE never really proved its worth in practice. So the interesting question is certainly what is different to believe that it will have its breakthrough now.
To answer this question, let's take a step back and look at today's challenges. As we move from functional safety to holistic safety, we need to consider many different disciplines - not in isolation but considering the massive interdependencies. This is a challenge in itself, as most companies keep the relevant responsibilities and competencies separated in different organizational units.
We also need to assure the safety of systems operating in an open, unpredictable, and uncontrollable world. Limiting the complexity of the world by abstracting it to a small vector of sensor values won't work for automated driving systems and the next generation of Advanced driver-assistance systems (ADAS). We want to use pre-existing software that was not developed according to safety standards and may be of unknown provenance. And we even want to make it possible to download new safety-critical functions in an app-like fashion. In addition, we want to assure the safety not only of individual vehicles, but also of complex V2X (vehicle to x) ecosystems. And with machine learning, we buy into an additional unpredictability that further potentiates the complexity of our endeavor called safety engineering. All this in the face of ever-shorter development and update cycles. In short, the question we hope to answer is, "How are we going to master this ever-increasing complexity in an ever-shorter time-frame?”.
Safetronic 2023
15 to 16 November 2023
in Stuttgart Area
Venue:
FILDERHALLE | Leinfelden-Echterdingen
The promise of MBSE is precisely that: the mastery of complexity. It could be argued that a major drawback of MBSE was the massive effort required to define the models and keep them up to date. This effect, called front loading, was probably a price that many engineers and/or managers were not willing to pay. Now, with a new order of magnitude in complexity, much shorter development cycles, and a lack of alternative answers, the urgency may have reached a level that convinces decision makers to invest in the additional effort needed to bring MBSE to life. Maybe it's just that simple. In fact, however, I think that's quite unlikely. For MBSE to be efficient, it requires that the functional development also follows a model-based approach, an assumption that is still quite unlikely to be true. Integrating all the different disciplines that are required for holistic safety, with all their different approaches and cultures into one model is even less likely. And another drawback remains: a model doesn't know what it doesn't know. And it knows only those aspects that an engineer has thought of and included in the model. Not a good starting point for dealing with unknown unknowns.
Does this mean that MBSE has no chance at all? No, in fact it is one of the most promising approaches. But not by digging the old approaches out of the chest and giving them a fresh coat of paint. Instead, we need to better understand the complexity we are seeking to master, and then re-apply the ideas of MBSE to solve our challenges.
Systems are complicated, the world is complex
Understanding complexity helps us better understand where we've come from and where we are or should be going. The Cynefin Framework [1] (cf. Figure 1) offers us structured guidance. Although it was developed for management and leadership issues, it also answers the question of how people should deal with different types of problems, namely simple, complicated, complex or chaotic. Like any comparison, applying Cynefin to safety engineering is flawed. Rather, it's an inspiring, structured way to change the way we look at our daily challenges. After all, safety engineers are human beings who need to manage simple, complicated, or complex problems. And just like managers, they need different approaches for each of these problem classes. Certainly, MBSE is not the only answer to the challenges of complexity. However, I would like to focus on MBSE as one of the current trends that has emerged during the conference to make complexity more manageable.
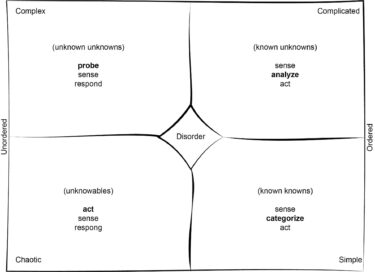
© Mario Trapp
The Cynefin Framework [1] offers us structured guidance for understanding complexity.
If we map the framework onto the type of system we have to consider (the car in its context), the right side of Figure 1 refers to what Snowden calls ordered systems. This is our preferred world of norms and order. For simple problems, there are established best practices that we can easily apply. If the problem to be solved (sense) is a delay failure mode (categorize), then we apply a watchdog to solve the problem (act). This "if-then-else" cookbook style of recipes is a very popular way to approach safety, especially for less experienced safety engineers. With some experience, we should have learned that the problems we have to solve are not so simple that simple recipes would suffice.
In fact, most systems present complicated problems. This means that there is no single best practice, but there are several good practices that might work. It's not possible to find a good solution directly, but we must analyze the problem first (sense-analyze-act). This requires experienced experts, who follow systematic methods or even perform more or less formal analyses. That's usually what most functional safety engineers do for a living. And most likely, most functional safety problems are no more than complicated. The more complicated the system gets, the more rigorous and formal analyses are required. And when we extend functional safety to other disciplines, such as chemistry, the resulting space of possibilities leads to an even more complicated problem.
Here, MBSE comes into play. MBSE promised to provide a formal basis for analyzing even highly complicated systems. But what makes a system complicated is not just its size, structure, or highly sophisticated behavior. It's also its variability. It may be that we need to assure hundreds of versions of a system per year, or that the system is constantly evolving over time. With each variation, a safety model erodes, and it takes considerable effort to keep it up to date. Nevertheless, there are several ways to make MBSE more efficient. Although it may sound paradoxical, the use of AI can help us to keep the model up to date and to include aspects from various sources such as code, test results, safety analysis, etc., where AI is good at finding correlations that we miss.
Call for Papers
Deadlines:
- Abstract submission: 10 May 2023
- Notification of acceptance: 23 June 2023
For information on the submission process and requirements, please refer to the website:
So if we want MBSE to have a future, it is important to reduce the manual effort required, to make it more intelligent, to make it a safety engineering companion that teams up with human safety engineers. If we can take MBSE to the next level, then it will be a great tool for mastering safety across various disciplines.
Mastering Complexity
However, when we include safety of the intended functionality (SOTIF), we are likely to see complex problems instead of complicated problems. The 19th-century philosopher William James once called our world a "blooming, buzzing confusion" – it’s constantly in flux and full of unexpected surprises. When we enter the realms of SOTIF, we enter the world of unordered problems, the world of the unknown unknowns. We can't control the environment by simplifying it to a small vector of sensor signals. The car must "understand" the world around it, and with that we are suddenly confronted with the full complexity of our real physical world. We have to consider people and their behavior, which is unpredictable. The regulatory landscape, new disruptive technologies, driving market forces, customer expectations - all are in flux. What works today may not work tomorrow. What we know in hindsight does not help us in foresight.
Now we're at the heart of our challenge: how to manage complexity. Following the ideas of Cynefin, we must acknowledge that we don't know the cause-and-effect relationships. And even if we did, what is valid today may be invalid tomorrow as the context is in constant flux. We must accept that we can only understand why things happen in retrospect. We can't prescribe a course of action and say, "If you follow this plan, your system will be safe.”. Cynefin recommends a probe-sense-respond strategy for complex problems. This means identifying emerging patterns that work. To do this, managers should use fail-safe probes, sense whether they are working, and respond by strengthening them when they have a positive impact, and suppressing them when they have a negative impact.
Applying these ideas of Cynefin to safety, it is important to set clear safety barriers within which new solutions can emerge. To this end, we need to take a step back to remember the real meaning of risk, of what a system must or must not do to be considered safe. We're trained to automatically translate safety into compliance or adherence to safety requirements, such as not using pointers and ensuring MC/DC test coverage. But these are the pre-digested analysis results for a complicated system. Because these measures have been effective in hindsight, we expect them to be good advice for the future.
But this conclusion is false because we have a different, ever-changing context. What we actually need to ensure is that the probability of a vehicle causing harm is acceptably low. It's about the behavior of the vehicle. What specific properties and measures lead to safe or unsafe behavior is what we still need to figure out. And we will have to do that all the time, because the appropriate solutions may change with changing contexts. Approaches such as RSS [2] are certainly not a perfect solution, but they are pointing in the right direction toward realizing safety barriers within which a vehicle can safely operate.
Gradually improving performance
Within these boundaries, we need to learn how to incrementally increase the performance of systems using a probe-sense-respond approach. In fact, approaches such as shadow-mode operation, safe ops, and the like already follow this paradigm. We limit the operational design domain and/or the deployed functionality to what we can guarantee. At the same time, we run various functions in shadow mode, we try to detect unknown situations, we try to collect safety evidence in the field, and we even move to the iterative development of safety norms to name but a few examples. All of these examples are based on probes that don’t pose an unacceptable risk if they fail. Our goal is to learn from these observations and gradually improve performance or even relax safety barriers. Continuous learning across all lifecycle phases, from early development to field experience supporting DevOps, becomes paramount.
In fact, the unordered world of emergent results is the home of machine learning. And so it is tempting to use statistical concepts based on correlation rather than causation for safety assurance. Even quality benchmarks from the world of machine learning are sometimes seen as a sufficient bar to clear for a system to be safe enough. However, cars are neither smartphones nor chat bots, and the fact that we have a complex problem to solve does not justify relaxing the level of what we accept as residual risk. But established approaches are not going to get the job done either. That’s why we need to build a bridge between the two worlds. This is where MBSE comes into play again.
There are three main areas where MBSE can play an important role in mastering complexity. First, because we can't plan solutions to a complex problem top-down, we have to learn them using the probe-sense-respond scheme. But learning does not mean using deep neural networks. Instead, we can train safety models. This leads to intelligent MBSE (iMBSE). Rather than defining the complete model manually, we can leave open gaps that can be learned, just as we can learn fully interpretable fuzzy models or mathematical equations. The models can be learned during the design phase, based on simulations and other existing training data, as well as during the entire V&V phase. But in combination with a shadow-mode architecture or the like, we can also safely train them in the field.
This leads directly to the second strand: By making models available at runtime, they provide great support for SafeOp approaches. Simply collecting data dumps and huge log files will lead to cognitive overload for the engineers involved in the SafeOp cycle. They'd have little chance of understanding the problem, let alone finding a solution. If we use safety models to reflect pre-processed data about unknown situations, incidents and the like in an iMBSE-supported SafeOp approach, it is much easier for engineers to understand the problem and derive appropriate solutions. They can easily continue their manual engineering process based on the safety models that have been learned in the field.
Stay up to date!
Would you like to be informed about further details on the Safetronic?
Then please sign up for our Safetronic information mailing list:
In a third strand, we can go even one step further. We can shift some of the responsibility of safety engineering to the system so that it can dynamically adapt to a concrete situation. While it is hardly feasible to manually consider all thinkable and unthinkable situations at design time, using runtime adaptation reduces the complexity to finding a solution for the current concrete situation. Of course, such safety intelligence must also be assured. Thus, if the safety intelligence is grounded in interpretable reasoning based on safety models, we have a promising foundation. In this way, iMBSE also opens the door to more sophisticated approaches such as adaptive safety control at runtime.
Summary
You may be wondering about the fourth category, chaotic problems. We enter this category when something unpredictable happens. More pragmatically, you'd find yourself in a chaotic situation when a car you're responsible for is involved in an accident or incident that makes negative headlines in the press. Then you'll be in a troubleshooting mode with an uncertain outcome. So, of course, this is something we have to avoid. But it will inevitably happen if we don't manage the complexity of the problem. In particular, trying to solve a complex problem with our planned, top-down approaches that we are used to solving complicated problems can eventually lead to chaos. And if you really try to treat it like a simple problem with safety cookbook recipes, you will inevitably find yourself in a chaotic situation.
Therefore, we must quickly learn how to deal with complex problems. MBSE is not the only ingredient, but it is a key ingredient. However, we need to extend the idea of MBSE to intelligent MBSE to deal with complicated cross-discipline problems, and at the latest to give approaches such as SafeOps a powerful yet formal foundation. Then they will also open the doors to safe run-time safety approaches. Ten years ago, MBSE was just a nice gimmick. Now we're facing new challenges that make approaches like MBSE imperative. We're not at the end of the evolution of MBSE, we're at the beginning.
References
[1] D. J. Snowden and M. E. Boone, “A leader’s framework for decision making. A leader’s framework for decision making.,” Harv. Bus. Rev., vol. 85, no. 11, pp. 68–76, 149, Nov. 2007.
[2] S. Shalev-Shwartz, S. Shammah, and A. Shashua, “On a formal model of safe and scalable self-driving cars,” ArXiv Prepr. ArXiv170806374, 2017.