Read next
The future of production
On the way to Industry 5.0?
Industrial production is undergoing radical change. With constant technological advancement and societal change, industrial automation must also reinvent itself to meet changing requirements and challenges – with the keyword here being adaptive production.
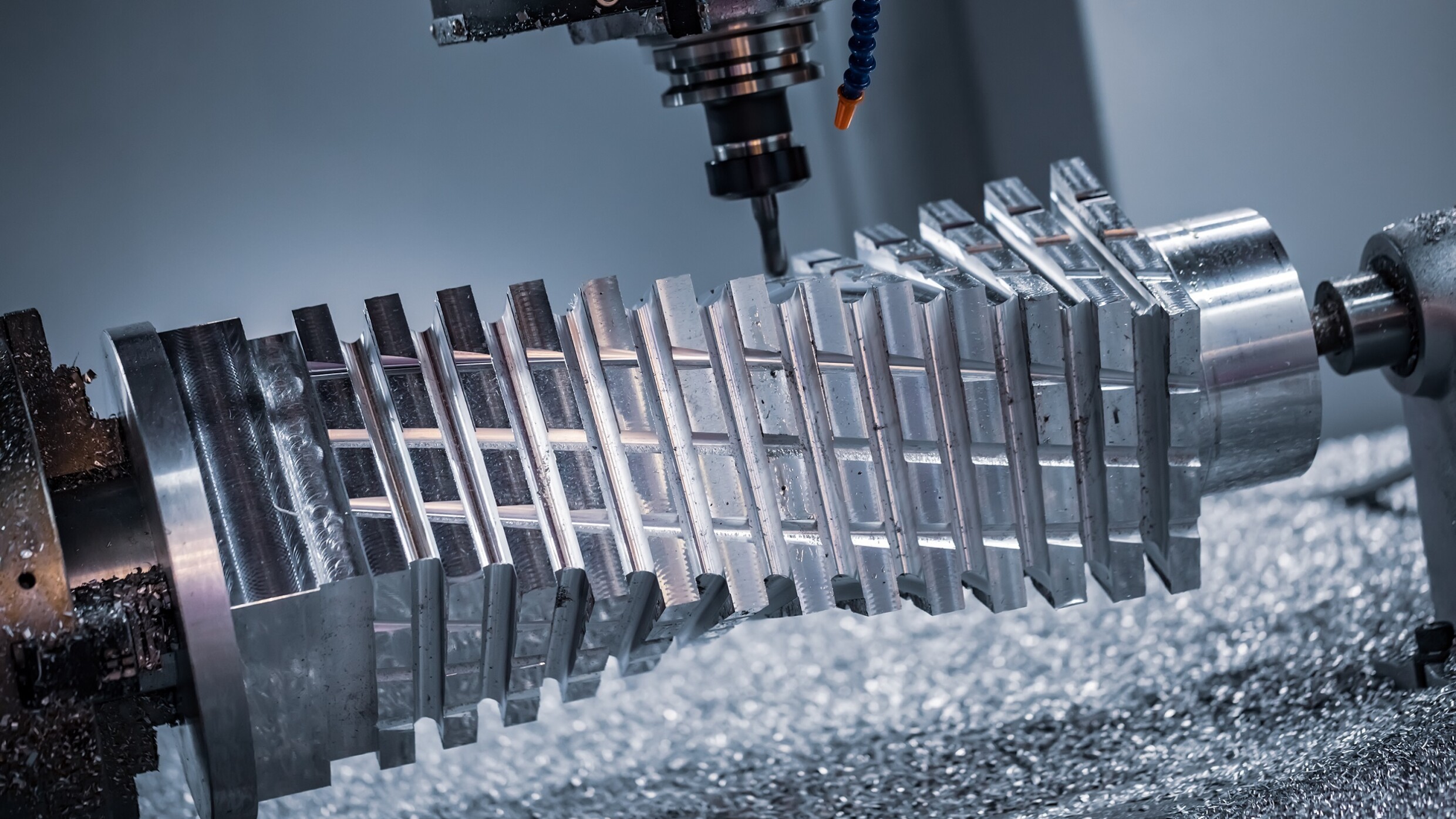
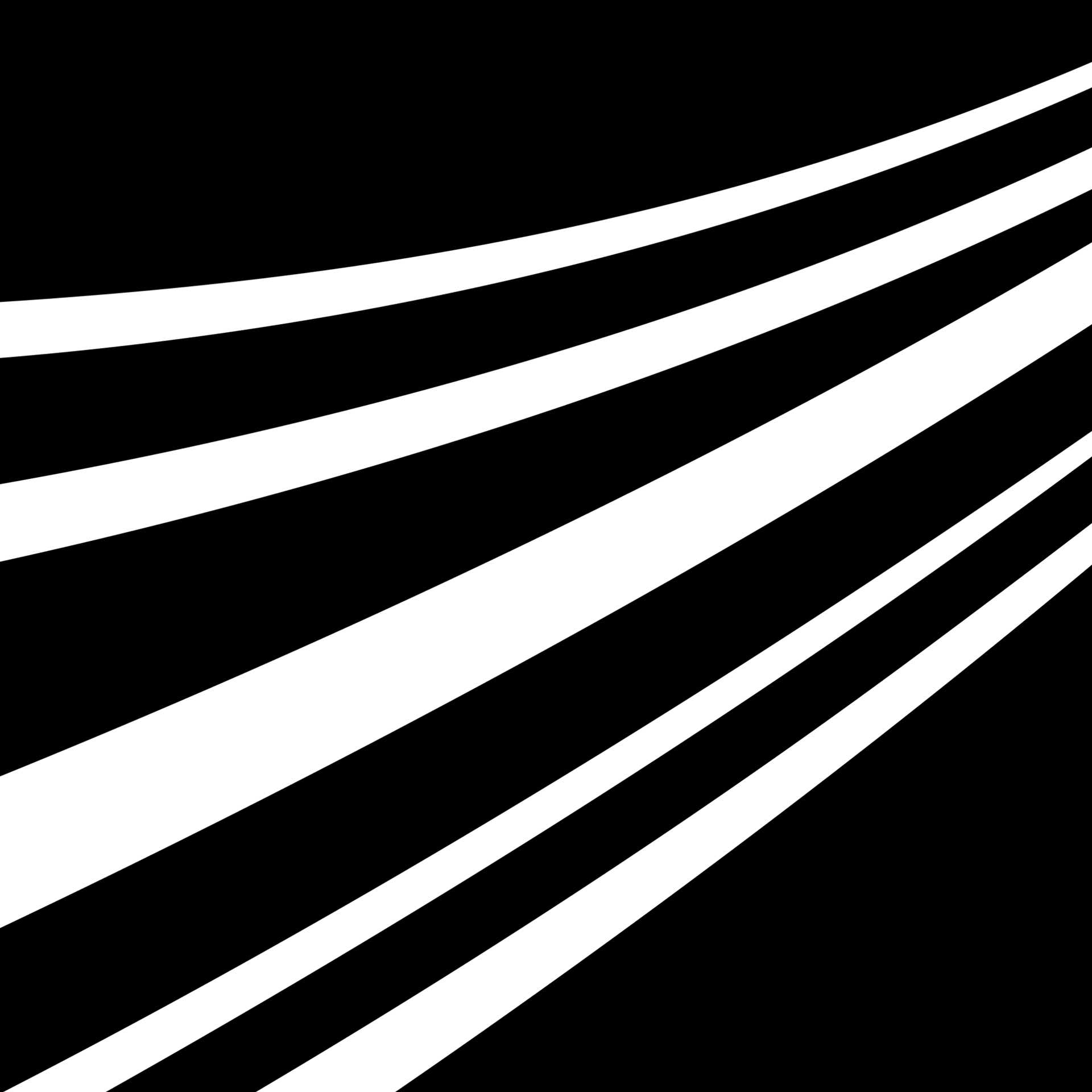
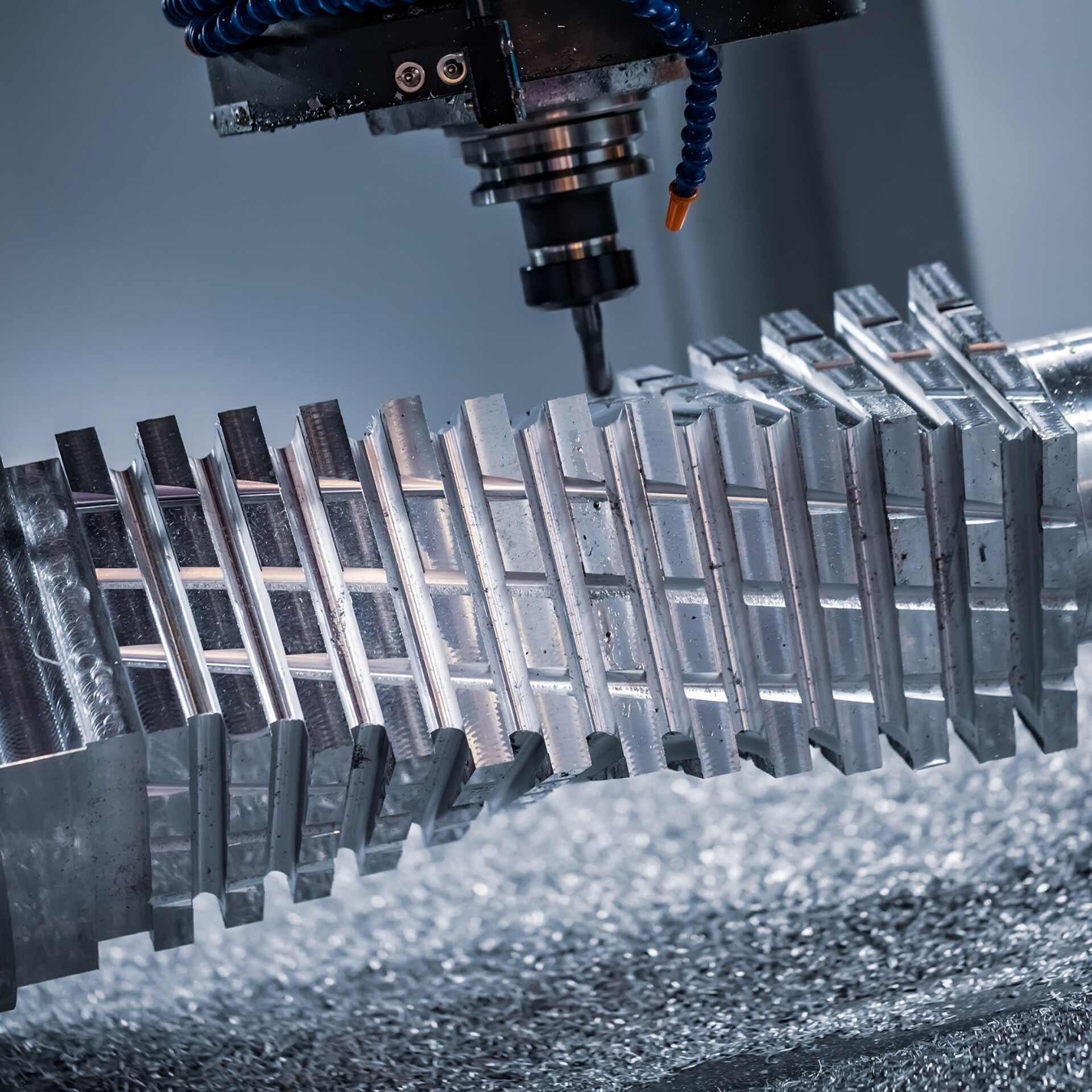
© iStock/cookelma
The Industry 4.0 approach has already significantly changed the way we produce goods. It opens up the possibilities for making production processes more flexible, automating and optimizing them through the intelligent networking of machines and processes. The next developments in industrial production will be primarily about bringing humans into the center of attention and enabling sustainable and resilient production.The EU even refers to this evolution as Industry 5.0[1], others take a more critical view of the term.
Anyway, as the pressure to innovate increased through digitization and advances in artificial intelligence (AI) in the form of machine learning (ML), the challenges in production are also changing[2].
ChatGPT and Other Chatbots
as a Game Changer?
Artificial deep neural networks (DNNs) are already capable of solving complex problems today. For example, Large Language Models (LLMs) such as ChatGPT are already being used to support humans, although the results produced by the bots stills needs to be revised by humans as those responsible for it. The implication here is that, already in initial approaches, the programming of a PLC (Programmable Logic Controller) can be simplified by generating program code or AI can also serve as an interface to the production system for simplified analysis. However, in order to be able to use such ML methods in safety-critical areas of production, further measures must be taken [2].
For example, to allow greater efficiency and flexibility, physical barriers such as mesh cages in the production environment should be removed. At the same time, ensuring workplace safety remains critical, despite the elimination of physical safety measures. Here, too, machine learning can assist, for example, through camera-based detection of persons near hazardous areas, such as a robotic arm in action. The key in these cases is that such detection must be sufficiently reliable and acceptable from a safety perspective. For this, it is helpful to explicitly consider the environmental conditions, the so-called Operational Design Domain.

© Fraunhofer IKS
Figure 1 Schematic representation of the safeguarding of a robotic arm using AI-assisted person detection in a defined ODD leading to safe shutdown ("OFF").
With such constraints and further measures to safeguard the ML components, such as ML engineering, monitoring or cross-validation, the potentials of inherently unsafe ML can also be exploited in production environments. The use of mobile autonomous robots in cooperation with humans also requires more comprehensive and adaptive safety concepts as we are researching at the Fraunhofer Institute for Cognitive Systems IKS. These concepts ensure high utilization while guaranteeing safety.
Digital Twins as Virtual Guinea Pigs
Further possibilities are offered by the use of what is called “digital twins”, which is a digital copy of the real elements. The Asset Administration Shell (AAS) is a standardized concept that enables such a representation. Numerous manufacturers already offer AAS for their products, so that a simple use of the data is possible.
In addition to the static information about a component, dynamic data generated during operation can also be used to obtain a digital image that is as comprehensive as possible. Such information in the form of an AAS runtime model is the basis for flexible adjustments such as those required for batch size 1 problems - i.e. the manufacturing of individual products.
Without Flexible and Adaptive Architectures It Simply Does Not Work
The underlying architectures of highly dynamic systems must become more flexible and adaptive. In production technology, we are now seeing how standard information technology (IT) is increasingly merging into operational technology (OT), i.e. the production-related control of all operational processes.
One example of this is the decoupling of physical dependencies by means of IT solutions. For instance, virtual PLCs, i.e. virtualized controllers, in principle allow control code to be executed in a distributed manner somewhere remote in a data center, so that these controllers can be purchased like a service according to production demand and utilization. Virtualized software components using container solutions such as Docker, which are common in PCs for developers, are also increasingly finding their way into the OT area and allow flexible exchange and installation of software.
Among other things, due to the long lifecycles of systems in the production area, this creates heterogeneous landscapes
that require individual technological solutions. Here, the integration of new technologies such as AI into existing production systems and the combination of new and old technologies pose a major challenge
Therefore, Fraunhofer IKS supports industrial partners in becoming more flexible and efficient with adaptive approaches and the use of new software architectures. For example, the framework developed by Fraunhofer IKS allows for the simple and flexible integration of AI through ML services into existing production systems[3], from the cloud to deep integration into control systems. Based on the AAS, a real-time image of the system can thus be generated as a digital twin and used to make optimal use of available or newly added computing resources.
This makes it possible to react adaptively to changes. One example is the altering of product variants, which leads to the AI model no longer functioning correctly, requiring it to be re-trained. The framework provides the basis for this so that this can be detected automatically, the AI model re-trained in the cloud, and returned to use at the appropriate quality control system. Another example is the simple retrofitting of a camera-based inspection with an ML model, which can detect production errors and can also be easily adapted.
© Fraunhofer IKS
Figure 2 Installation of camera-based AI monitoring on a production line (left) with a displaying of the current status in the dashboard (right).
High demands on flexibility and resilience can thus be realized cost-efficiently.
We are happy to support you in the development and implementation of such adaptive concepts as well as in the integration of AI in production. Contact our business development team: business.development@iks.fraunhofer.de
In sum, the potential of digitalization and AI for industrial automation is enormous. However, it is also not without its challenges, which companies must master if they wish to remain successful. With the approaches and solutions of the Fraunhofer Institute for Cognitive Systems IKS, users are enabled to ensure high flexibility, resilience and support for humans in production as well as safety in complex scenarios.
A part of the work is carried out in the framework of the project “REMORA – Multi-Stage Automated Continuous Delivery for AI-based Software & Services Development in Industry 4.0”, which is funded by the Bavarian Ministry of Economic Affairs, Regional Development and Energy and supported by Bayern Innovativ – Bayerische Gesellschaft für Innovation und Wissenstransfer mbH.