Read next
Industry 4.0
The Parallel World of Digital Twins
Digital twins are designed with the goal to create precise replicas of our physical world, but how exactly are they being used? For example, in medicine, vehicle construction and industrial production? Read on to find out.
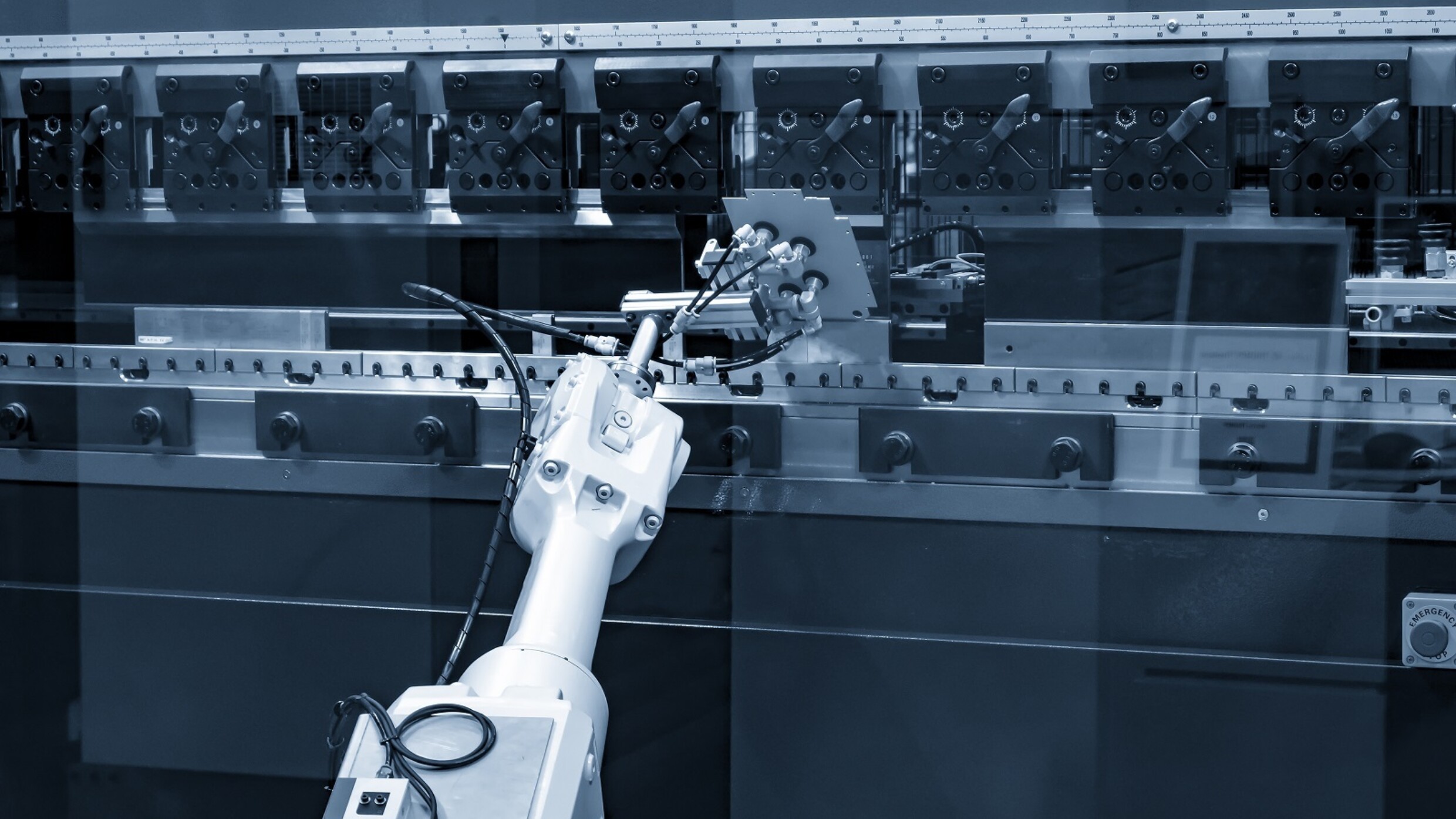
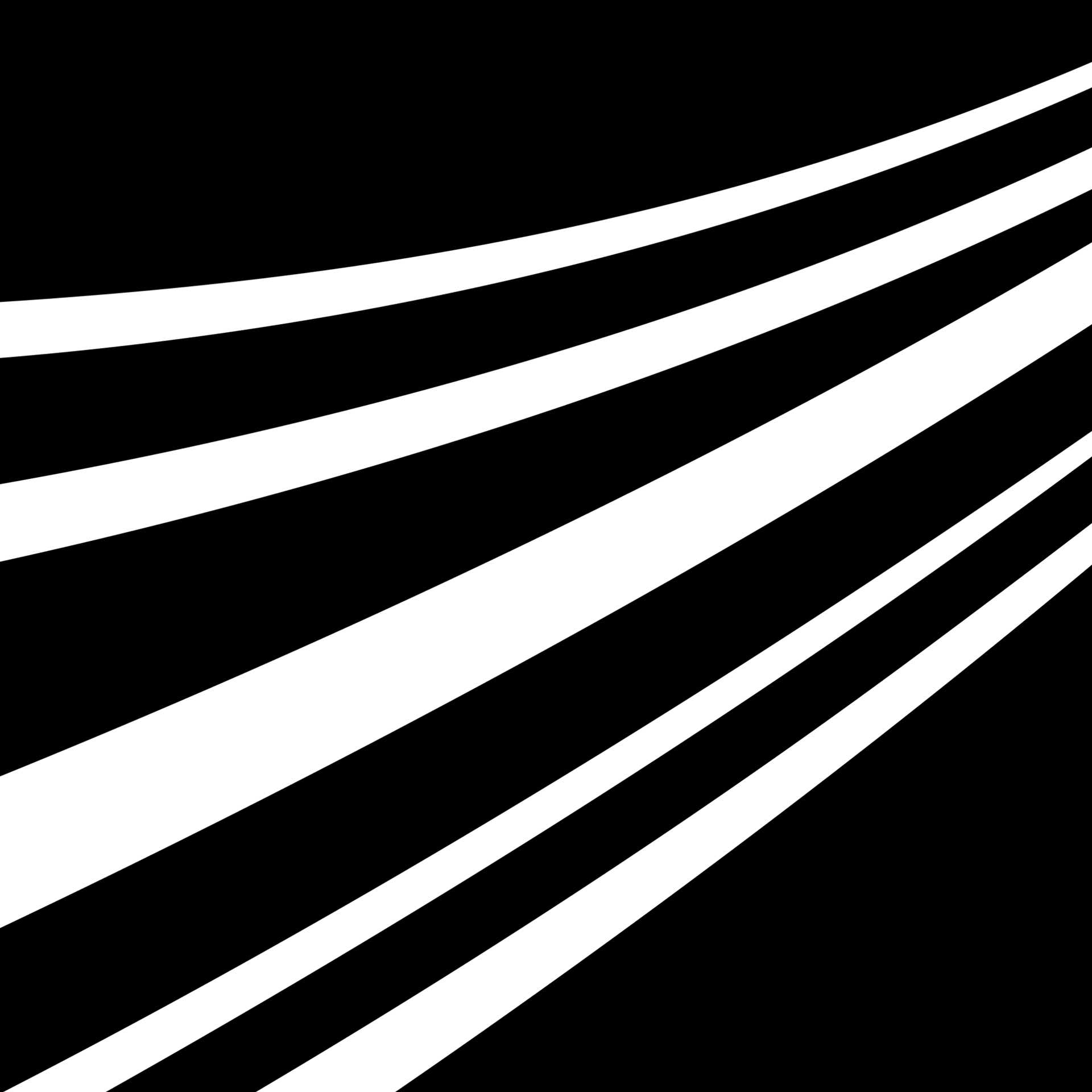
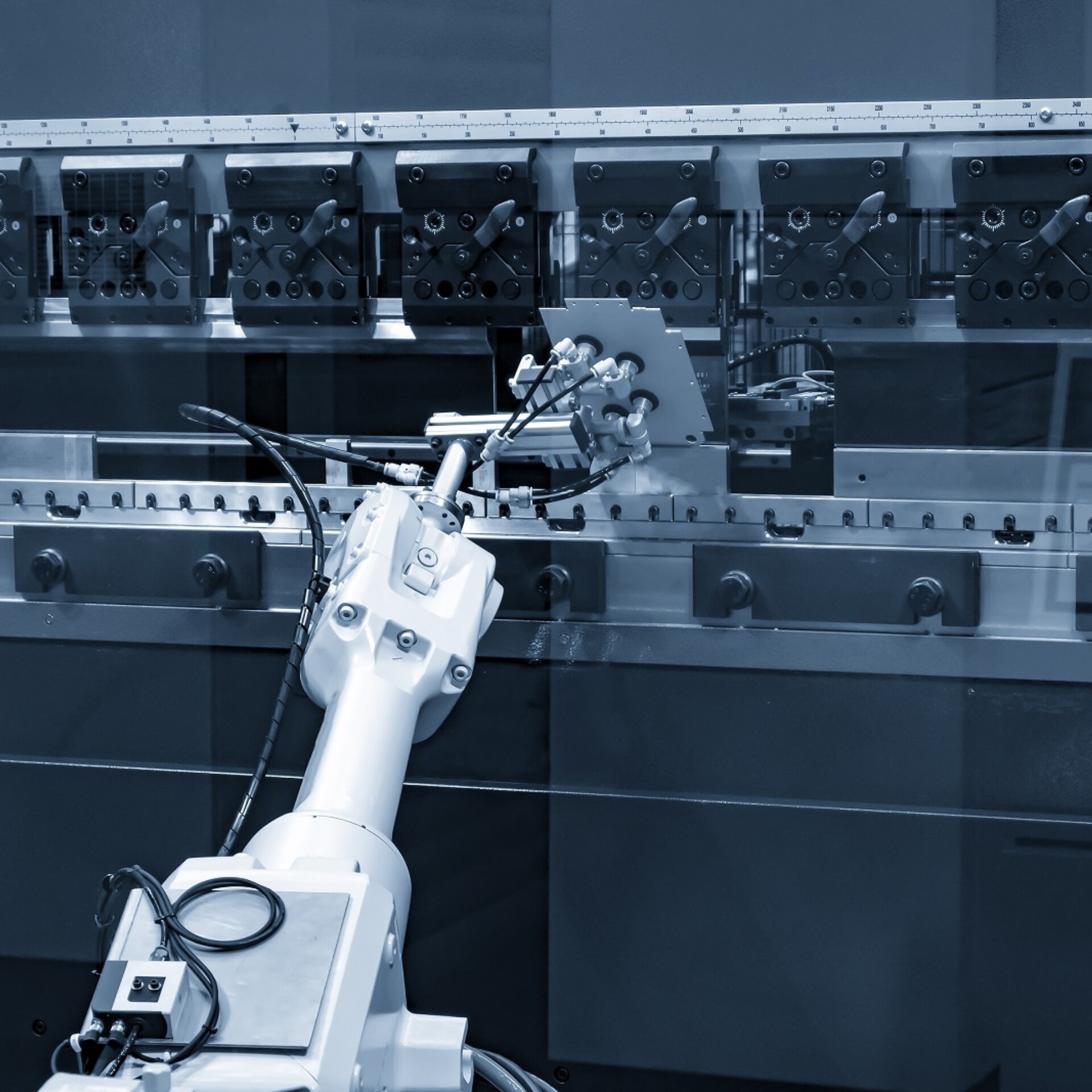
© iStock/Phuchit
The ongoing digitalization wave continues to impact businesses in many areas of research, development, and production. Pharmaceutical companies, for instance, are now benefiting from new techniques for streamlining their drug development process. These techniques focus on cutting down clinical trial times by using technology which mimics patient responses to new substances. It may sound a little like science fiction, but the idea is to create a digital twin (i.e., a virtual representation) of a patient, then simulate the effects of a drug on this substitute.
For this to work effectively, these digital twins must be created using extensive data from real-life patients, as well as simulation environments that accurately capture chemical and physiological processes within the human body. Once all this clicks into place, it becomes possible to speed up observation times and even predict trial outcomes. Ultimately, this technology could shave years off notoriously long pharmaceutical trials, in turn saving companies millions in investment.
What does a digital twin look like?
The example above highlights the advantages of using digital twins, but it does not explain how exactly they are structured. As previously stated, a digital twin is a virtual representation of a physical asset, useful for simulating the impact different processes may have on the given asset. In practice, a digital twin is a software model that abstracts some key information about an asset. The nature of this information depends on the purpose this model is trying to fulfil, which is informed by the broader context.
For example, a digital twin may be developed by an automotive company to represent a vehicle, with the goal of tracking the unit’s power consumption in various situations. In this case, it may not be necessary to model all vehicle components (i.e., its nuts and bolts) in detail, since this information is likely not useful for the calculation of the overall power consumption. However, if the focus is on optimizing the assembly process for the vehicle, then carefully modelling all moving parts suddenly becomes crucial. This change in perspective is not an issue, since a single digital twin can hold multiple complementary models which are applicable for different contexts. Another key aspect this example highlights is that digital twins do not only hold static information about an asset, but can also dynamically simulate its behavior in diverse scenarios.
Uses in Industry 4.0
Clearly, digital twins can be used in many different areas, but the common thread discussed thus far is manufacturing. With the digitalization of production lines, it has become possible to collect data from all aspects of fabrication. This includes not only product attributes (e.g., the energy consumption of a vehicle or the onset time for medication), but also information about the machines used for manufacturing these goods. This smarter production paradigm is often referred to as Industry 4.0 (as an allusion to a fourth industrial revolution aiming to increase data exchange and automation), and digital twins are at the center of this concept.
The ability to thoroughly monitor the production line and collect timely information unlocks compelling possibilities for improving the manufacturing process. For instance, digital twins in Industry 4.0 can be applied for predictive maintenance, which consists of analyzing data from equipment to determine when maintenance should be carried out. The goal in this case is to prevent the failure of machines during peak production times, which would financially impact the business. As another example, having information about the behavior of machines over time can uncover opportunities for process optimization, which helps close productivity gaps (e.g., occasional idle periods for equipment) in the production line and improves the overall yield. Of course, these are only two of the many possible ways in which industrial digital twins provide tangible benefits.
The Asset Administration Shell
Due to the popularity of digital twins among Industry 4.0 players, an implementation standard – the Asset Administration Shell (AAS) – has been developed for modelling devices (but also products and processes) in the production line. The AAS is a technology-agnostic specification that allows the state of a device to be dynamically monitored and controlled via a unified interface. To model different facets of the device, the AAS provides different submodels, each supporting a different use case. An example of such a submodel is the digital nameplate, which shows all relevant information about the device, e.g., safety documentation or the next scheduled maintenance date. This is known as a "passive AAS", and it is useful because it creates a digitalized one-stop shop for all records related to a device (accessible by scanning a QR code on the machine). However, researchers have also been developing the "active AAS", which is when changes to the submodels in the Asset Administration Shell are directly reflected on the underlying device.
Current uses at Fraunhofer IKS
Given its versatility, the Asset Administration Shell is also being used at the Fraunhofer Institute for Cognitive Systems IKS. Specifically, the Fraunhofer IKS team is developing a flexible framework for the integration of AI into manufacturing systems. The framework is structured using a service-based architecture, meaning that the different pieces of AI (Artificial Intelligence) functionality are encapsulated within services and then deployed to suitable devices. This is where the AAS comes in: By modelling all devices on the production line using Asset Administration Shells, it becomes possible to check where services can be deployed to, where data for AI is coming from, etc. Additionally, the use of AAS can be extended in several directions, from tracking the internal state of devices (rather than only the hardware status) to simulating their performance under different configurations.
Next Steps
For businesses interested in creating and using a digital twin, the first step is to decide which aspects of the underlying device must be modelled for the envisioned usage. This can be accomplished either manually or programmatically, depending on the complexity of the model and on the preferences of the team. Then, the next step is to ensure that the corresponding AAS is available on a dedicated server (and registry), thus ensuring access to its information. Once this infrastructure is in place, it becomes possible to write code that interacts with the AAS and leverages its data to improve the manufacturing process, for instance. Fraunhofer IKS can help with all these steps, supporting the setup and exploitation of digital twins for the best outcomes.