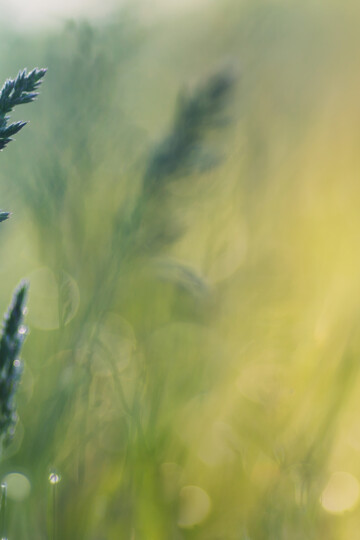
Machine Learning
Metrics as an indicator of AI safety: is that enough?
The performance of machine learning (ML) models is typically assessed using metrics such as accuracy, precision, recall, F1 score, etc. But how trustworthy are these single point estimates really? Such metrics reflect reality only partially and can provide us with a false sense of safety. Especially in safety-critical areas, this can be dangerous. Fraunhofer IKS develops a formal framework for the quantification of uncertainty which aims to make the residual risk “more computable”.