Read next
Quantum Computing
Advancing Medical Diagnostics with Quantum-Powered AI
AI has paved the way for better and faster diagnostics in the realm of medical imaging. However, challenges specific to the field of medicine, notably the scarcity of data, can impede its performance. This article looks into the enhancement of AI algorithms using quantum technology for the improvement of medical diagnostics, showcasing how quantum computing can tackle existing challenges in AI and offer unique opportunities for healthcare.

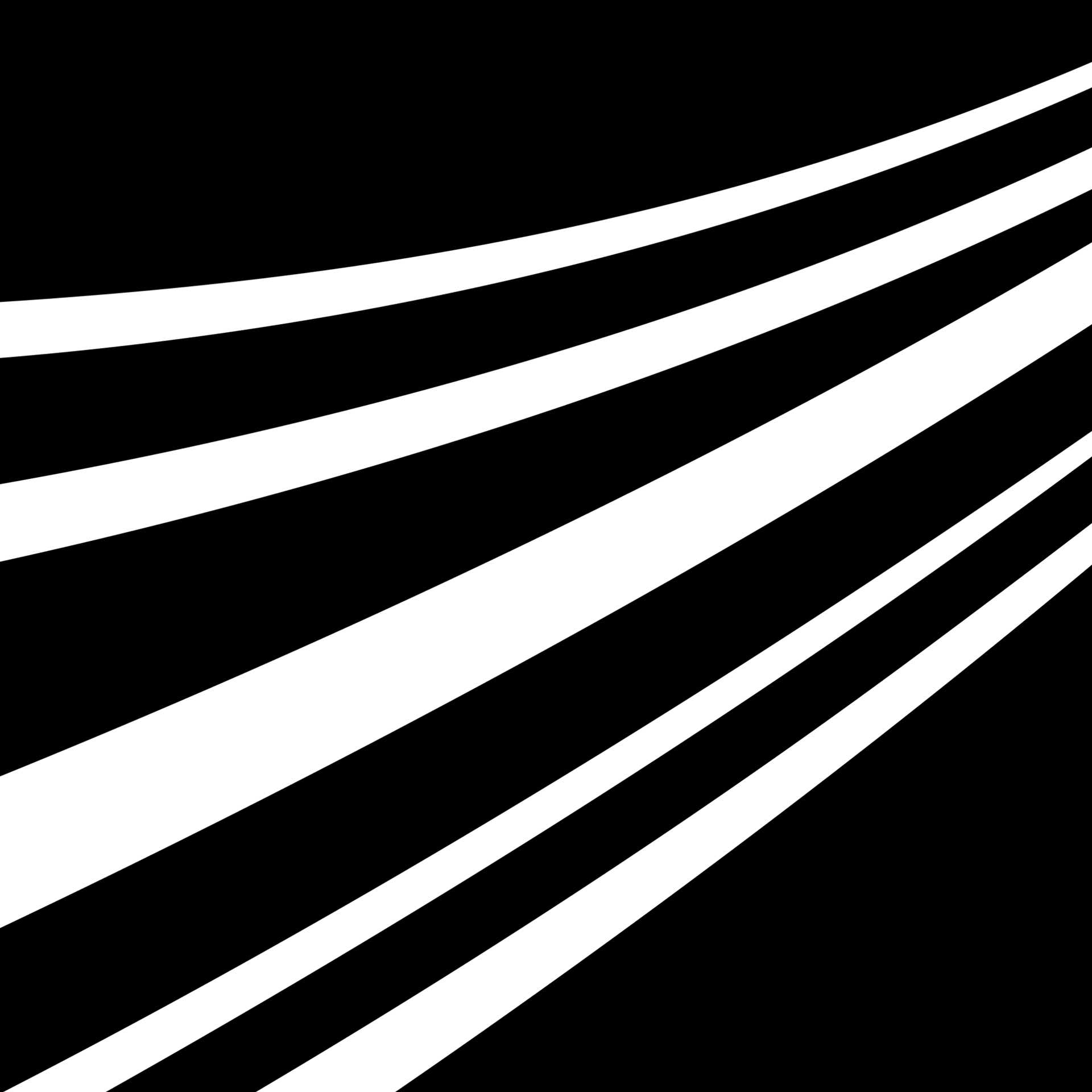
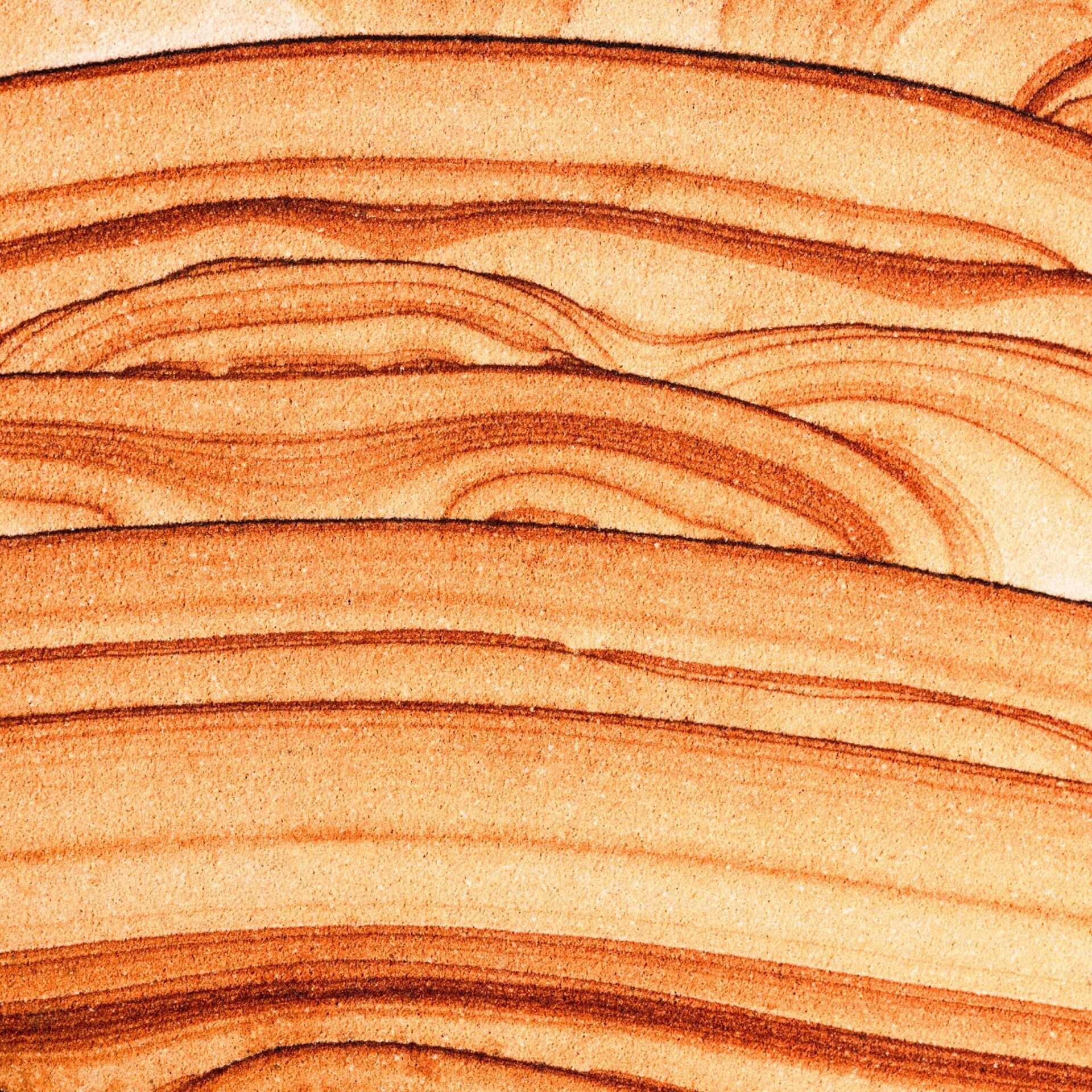
© iStock/ georgeclerk
AI methods, particularly Convolutional Neural Networks (CNNs), have demonstrated remarkable performance in various imaging tasks. In the medical field, AI has garnered significant attention for achieving human-level performance and revealing novel insights. CNNs are commonly employed for analyzing medical images. They work by extracting distinctive features in the images using convolutional layers, and then categorizing them through fully connected layers.
A practical application for the medical field lies in the analysis of computerized tomography (CT) scans. These scans are built by combining X-ray images taken from different angles to acquire three- dimensional representations of the examined area. They are used in radiology to diagnose diseases and plan medical treatments. The promise of AI is to assist the medical expert in the diagnosis, thereby enabling faster and more accurate diagnostic and treatment strategies.
Dealing with Data Challenges in Medical AI
While AI holds immense promise, its effectiveness strongly depends on access to extensive training datasets. Training algorithms to achieve high prediction accuracy requires a sufficiently large data library., Medical imaging tasks face a unique challenge when it comes to acquiring sufficient data due to the expenses tied to imaging procedures, expert annotations, and privacy considerations. This problem is even more pronounced in cases of rare diseases, where patient numbers are limited. Existing high-quality datasets are relatively small, often ranging from a few hundred to a thousand samples. To realize the full potential of AI in medical imaging, it is therefore imperative to address the efficient utilization of such limited datasets and overcome these challenges. This involves ensuring high accuracy, establishing trustworthy algorithms, and navigating the complexities of medical imaging intricacies.
This is exactly where quantum technologies can help, as it has been shown that quantum-enhanced AI algorithms are able to perform well even when a limited amount of training data is available. The Fraunhofer Institute for Cognitive Systems IKS is exploring QCNNs (Quantum-enhanced Convolutional Neural Networks) as part of the Bavarian Competence Center for Quantum Security and Data Science (BayQS), together with the Radiology Clinic and the Neurosurgery Clinic at Ludwig-Maximilians-Universität München (LMU Munich) in a project funded by the Bavarian Ministry of Economic Affairs.
Does Quantum-Enhanced AI make a difference?
In our paper Quantum-classical convolutional neural networks in radiological image classification [1], we introduce a hybrid Quantum-Classical CNN (QCCNN), where the classical CNN algorithm is enhanced by a quantum kernel. The underlying motivation is that the quantum kernel has the capacity to find more complex patterns in the image compared to its classical counterpart. The rest of the CNN remains classical, making this algorithm truly hybrid. Today’s quantum computers, so called NISQ (Noisy Intermediate Scale Quantum) devices, contain a limited number of qubits and are susceptible to errors due to environmental factors.
Given these constraints, hybrid models that leverage quantum capabilities for specific parts of the algorithms are most promising for contemporary quantum algorithm design. We therefore design different QCCNNs varying by the encoding scheme for encoding classical data into quantum data, and by the design of the quantum circuits. These circuits consist of sequences of quantum gates which create the entanglement and superposition phenomena intrinsic to quantum mechanics, allowing the algorithm to perform certain calculations much more efficiently.
We apply this hybrid algorithm to identify tumors in ultrasound images of the breast and to 2D and 3D CT scans to classify organs and find cancerous lesions in the lung. We benchmark our algorithm against a fully classical model and observe that the performance of the hybrid algorithm is similar, or in some cases even better, than the classical model. We conclude that such algorithms are promising for solving the challenges of the medical field where a good performance is required, and a low amount of data is available.
We follow up on these findings in Pooling techniques in hybrid quantum-classical convolutional neural networks [2], where we move an additional part of the classical CNN algorithm to the quantum computer: the pooling mechanism. In a CNN, the pooling operation typically follows the convolutional layer where the features are extracted and it is crucial as it down-scales the input to a smaller data size and thereby ensures that the learnt image features are robust.
In our work, we compare four different quantum strategies for implementing this pooling operation in the quantum world, while still designing hybrid algorithms which minimize the number of qubits involved and the length of the circuits. The selected medical use case is the recognition of breast tumors in ultrasound images. We find that most of the quantum models outperform a comparable classical baseline and propose a quantum metric to determine in which cases such a quantum advantage is observed. Our results, obtained through simulation using libraries for running quantum machine learning algorithms, show significant potential for further studies of hybrid quantum-classical CNNs, as those might be suited for presently available NISQ hardware.
We will present this latest work in the IEEE quantum week 2023 this September and would be delighted to engage in a more detailed discussion about it during the conference.
[2] Maureen Monnet, Hanady Gebran, Andrea Matic-Flierl, Florian Kiwit, Balthasar Schachtner, Amine Bentellis, and Jeanette Miriam Lorenz. Pooling techniques in hybrid quantum-classical convolutional neural networks, 2023.