Read next
Artificial Intelligence
What happens when AI fails to deliver?
Artificial intelligence (AI) has to be able to handle uncertainty before we can trust it to deliver in safety-critical use cases, for example, autonomous cars. The Fraunhofer Institute for Cognitive Systems IKS is investigating ways to help AI reason with uncertainty, one being the operational design domain.
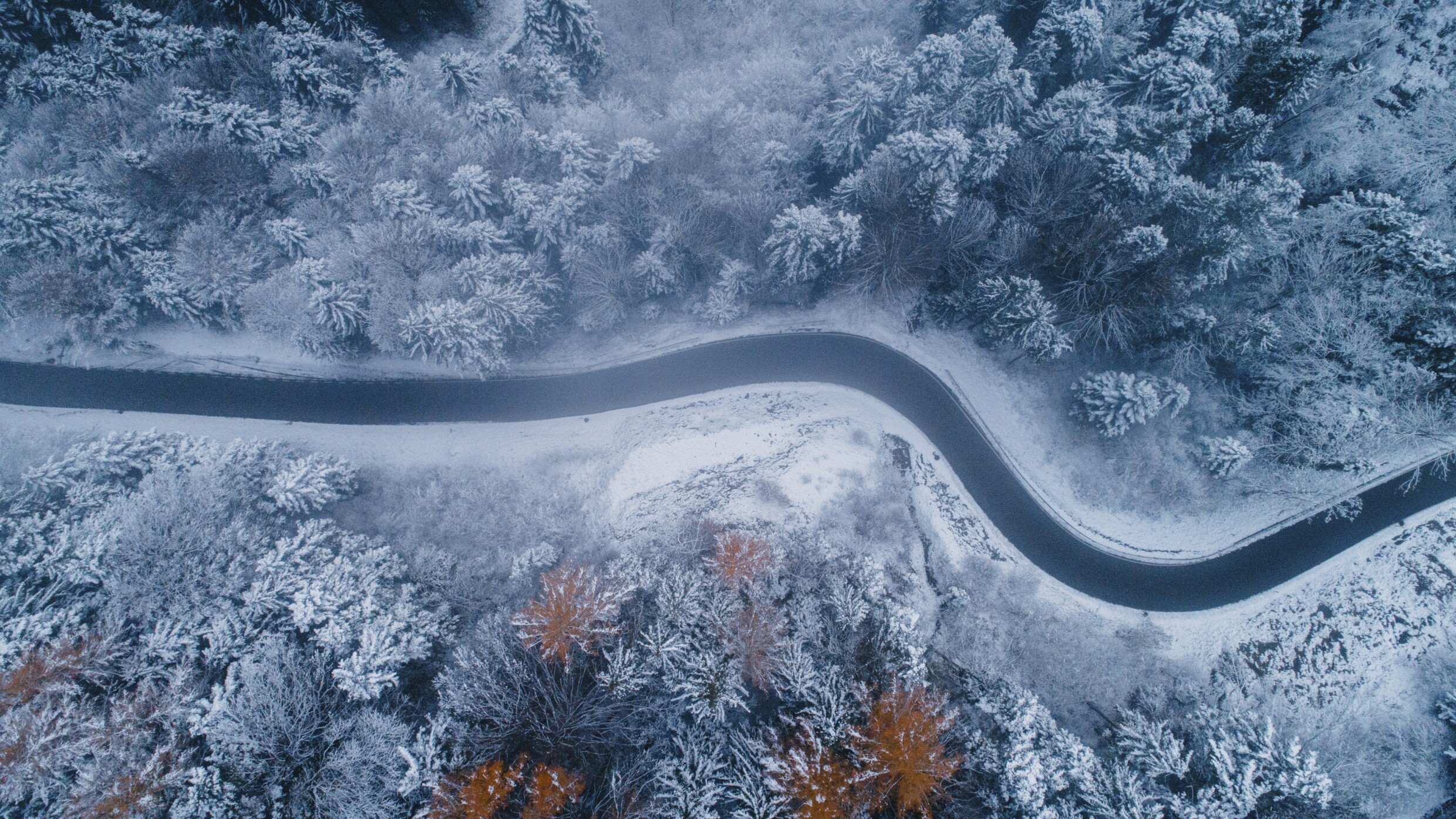
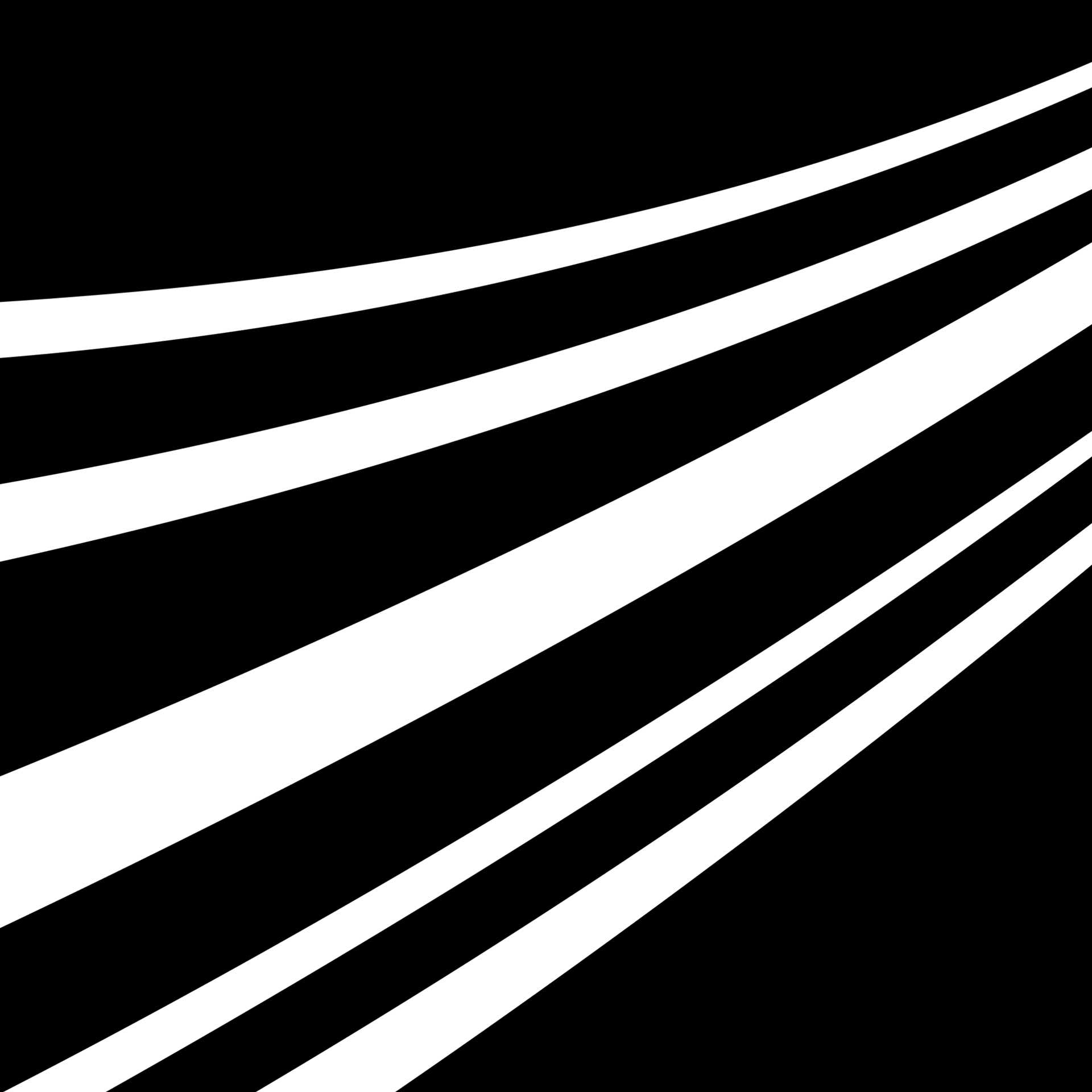
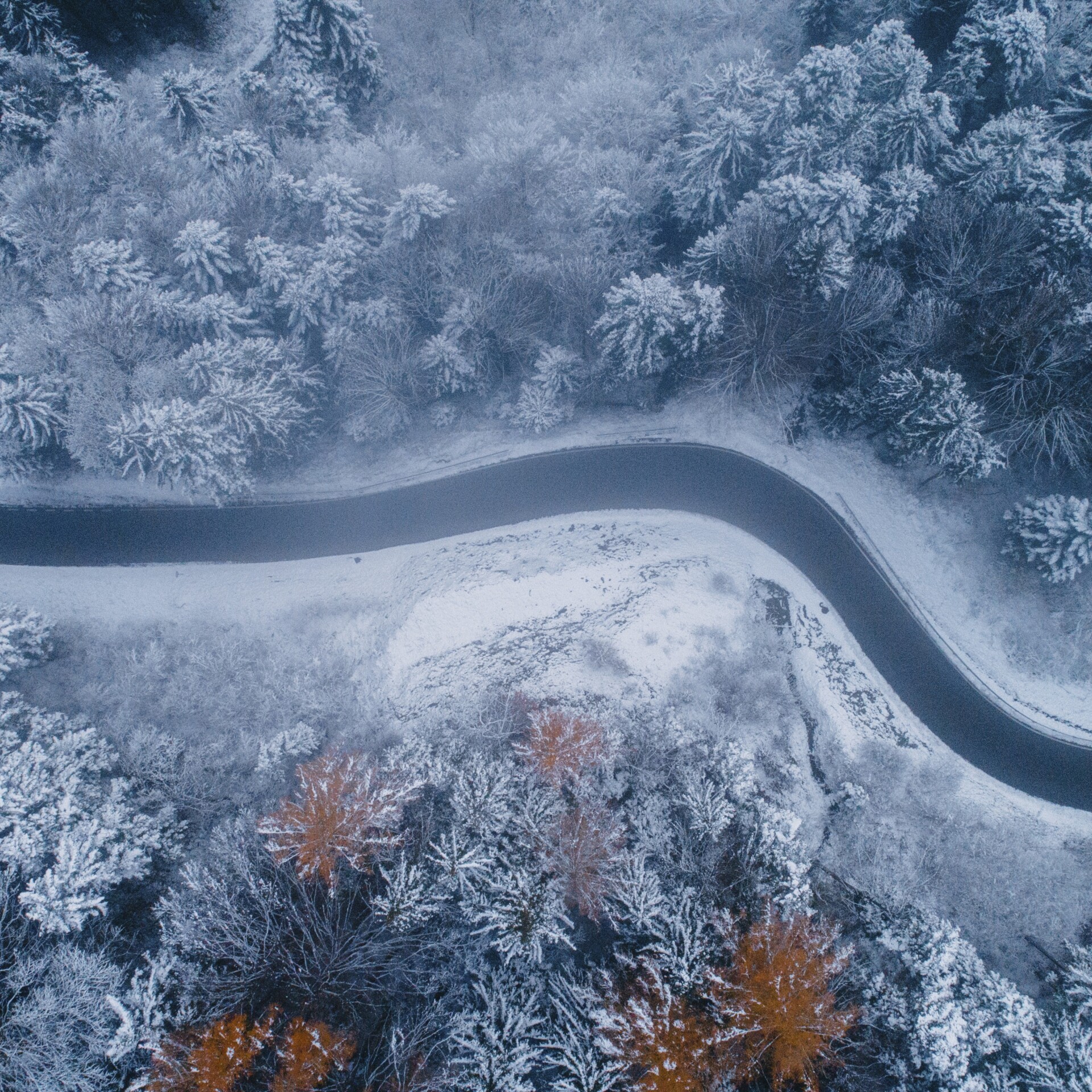
© iStock.com/Oleh-Slobodeniuk
Recent advances in artificial intelligence are certainly impressive. One AI can distinguish between a hotdog and a not-hotdog. Another can play chess well enough to go toe-to-toe with grandmasters. Still another is revolutionizing medicine by demystifying protein folding mechanisms.
In light of these extraordinary feats, we can hardly be faulted for our great expectations of AI-powered systems. But have we really arrived at a point where we can contently snooze behind the wheel while an AI-powered car drives us to our destination? Those of us who have seen self-driving taxis operating in urban neighborhoods would be inclined to say yes.
Would the answer be the same, if that taxi had to navigate the hairpin turns of a winding mountain road amid a torrential downpour? Probably not. Doubts about self-driving cars’ safety are not unfounded given incidents like the fatal Uber crash that made headline news. Much of the blame for these accidents goes to AI’s inability to assess certain situations accurately, but some of it is down to us humans grossly overestimating its capabilities.
AI fails at certain tasks that humans perform effortlessly. Here’s why
Deep neural networks (DNN) are an attempt to emulate the human brain’s workings. Although DNNs do approximate the structural characteristics of our networked neurons, they fall short when it comes to replicating the aspects responsible for the brain’s semantic understanding.
A case in point: A convolutional neural network (CNN) tasked to categorize the objects in images reasons from the bottom up to identify patterns that suggest pedestrians, road signs and other objects. Humans do that too, but we also reason from the top down. We can readily deduce partly snow-covered road surface markings, stop signs and the like from visible markers, but the CNN does not have that line of reasoning to fall back on.
Our grasp of the world around us is the product of lifelong learning. Instilling this understanding in a DNN is difficult, if not impossible. Despite all its sophistication, AI still lacks a semantic understanding of the world in which it operates.
We have outlined the overconfidence, the inability to recognize novel inputs and various other issues that cloud a DNN’s perceptions in another article. The research at Fraunhofer IKS addresses these challenges in an effort to improve AI’s performance. But even an unreliable AI component can serve a use case well when integrated into a system using the operational design domain (ODD). A closer look at ODD follows.
Building trust in self-driving vehicles with ODD
Building trust in the Automated Driving System (ADS) and its AI components is all about substantiating its safety. This calls for a mechanism that guarantees fail-safe operation without compromising performance. One way is to limit the scope of an ADS’s operations to a smaller set of operating conditions (OC) that have been tested and validated to be safe. Engineers can add OCs to this set as the ADS matures and new tests verify its safety.
The best approach is to factor the ADS’s designated environment, capabilities and limitations into the equation and then determine the conditions under which the system is safe to operate. For example, a low-speed autonomous taxi’s could be confined to roam a specific area that has been thoroughly tested; a smaller safe-zone with well-defined routes and time-of-day conditions. The Society of Automotive Engineers (SAE) has come up with a term for the conditions under which an ADS is designed to work – the operational design domain (ODD) as specified in SAE-J1306.
Once engineers determine the ADS’s capabilities and limitations, they can define an ODD specification that this ADS will support. Finally, they monitor the operating environment to pinpoint OCs that the supported ODD does not cover.
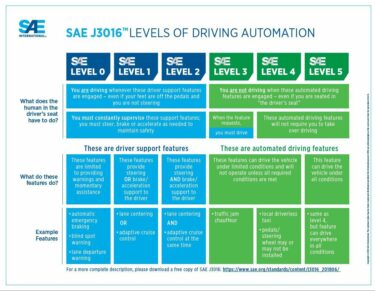
© SAE J3016 (https://www.sae.org/news/2019/01/sae-updates-j3016-automated-driving-graphic)
This is how the levels of automated driving are defined including the role of the driver and the features offered.
An OC violation triggers an ODD exit. Then appropriate steps can be taken as required for the given automation level, SAE 3 or 4. Driver hand-off is an option for SAE Level 3: The driver takes control and navigates the situation safely at his or her discretion. Driver hand-off is not an option for SAE Level 4. The appropriate response in this case could be a fallback dynamic driving task (DDT): The vehicle slows down and finds a safe spot to stop, the idea being to deescalate to a minimal risk condition (MRC) – in other words, to park the car. This is why the ODD and the need to monitor it are so crucial to building trust in ostensibly unreliable AI-based systems.
What is an ODD?
SAE J3016 defines ODD as “Operating conditions under which a given driving automation system or feature thereof is specifically designed to function, including, but not limited to, environmental, geographical, and time-of-day restrictions, and/or the requisite presence or absence of certain traffic or roadway characteristics.” The PAS1883 standard provides the taxonomy for defining an ODD. It specifies three major categories of operating conditions:
- Environmental conditions – weather, illumination, particulates, connectivity, etc.
- Scenery – zone, drivable area, junctions, special road structures, etc.
- Dynamic elements – traffic, subject-vehicle, etc.
OpenODD is an ongoing standardization initiative to develop a first concept for an ODD specification format. Fraunhofer IKS is on board for this OpenODD project.
With the benefit of an ODD specification, engineers can test the ADS in actual trials and assess its safety within the scope of this designated ODD. If they find that an ADS is operating in an environment other than one it was designed for, they can take remedial action. This is why an ODD specification and its monitoring at runtime are so crucial to building trust in the system.
This activity is being funded by the Bavarian Ministry for EconomicAffairs, Regional Development and Energy as part of a project to support the thematic development of the Institute for Cognitive Systems.