Read next
Machine learning in medicine
Data-driven diagnostics improve the health of premature babies
Babies born prematurely, i.e. before their organs have fully developed, often suffer from various health problems, known as morbidities. These rarely manifest alone, but often occur simultaneously. Researching connections or even patterns in their co-occurrence helps to develop more effective and more personalized care for premature babies. A project report.
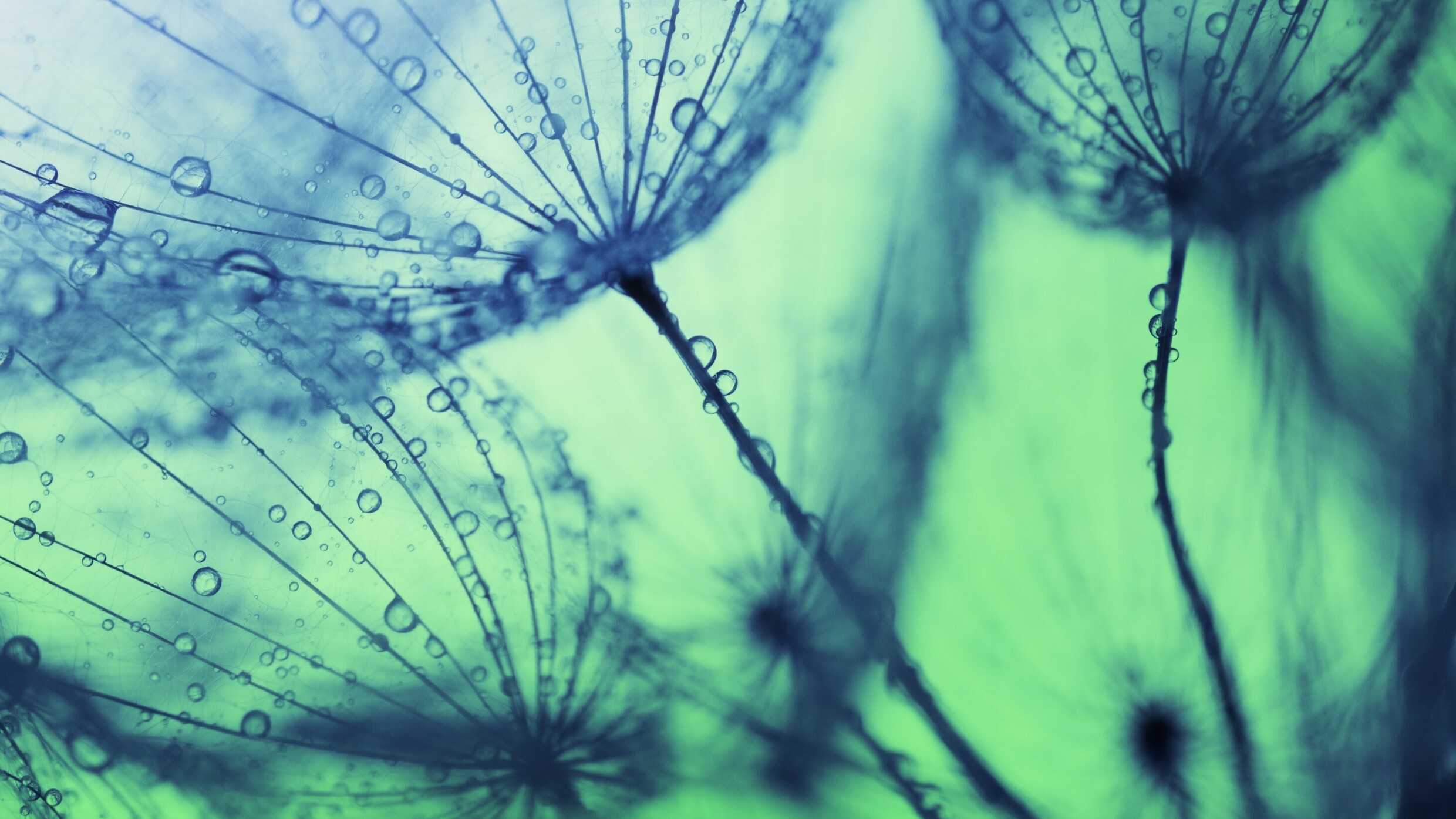
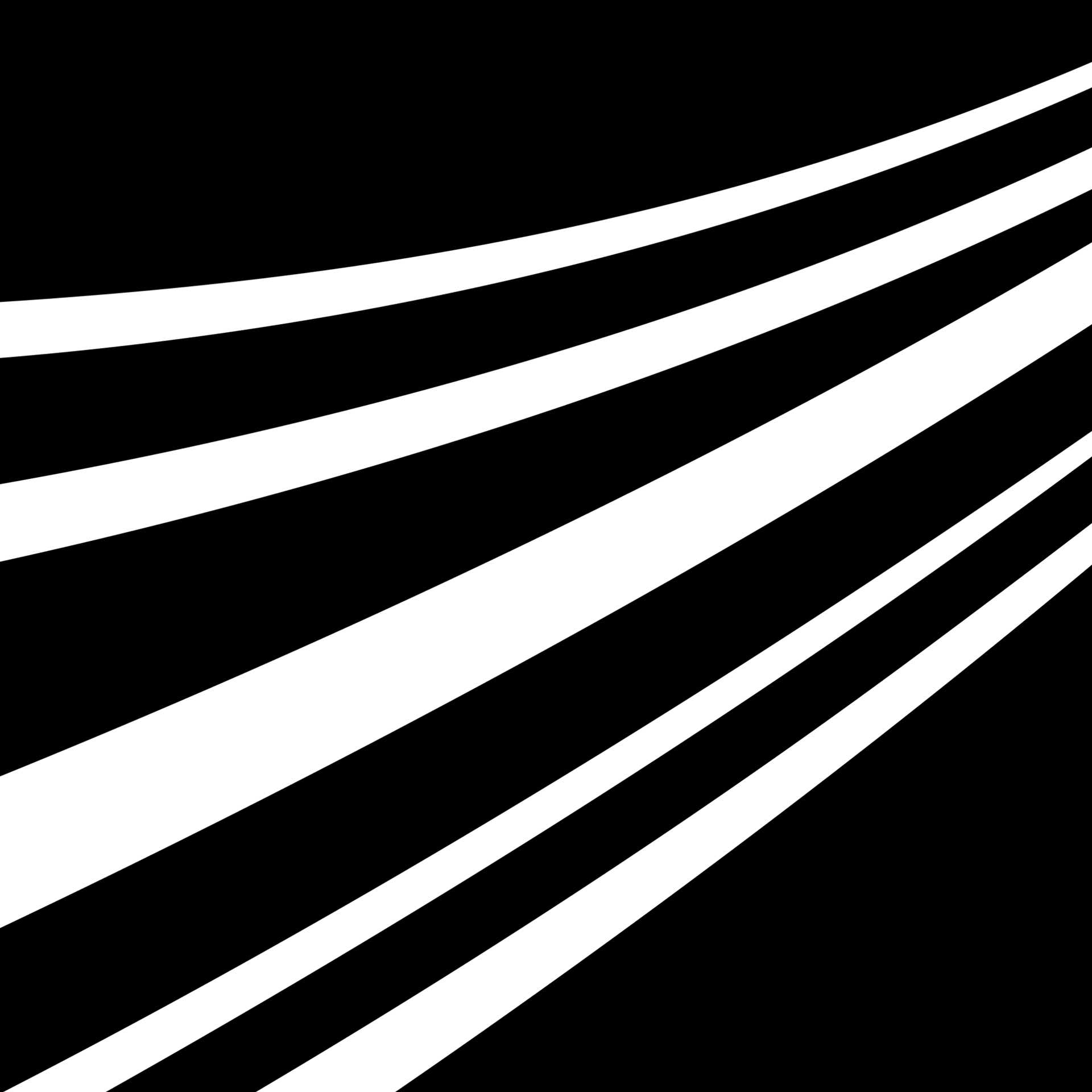
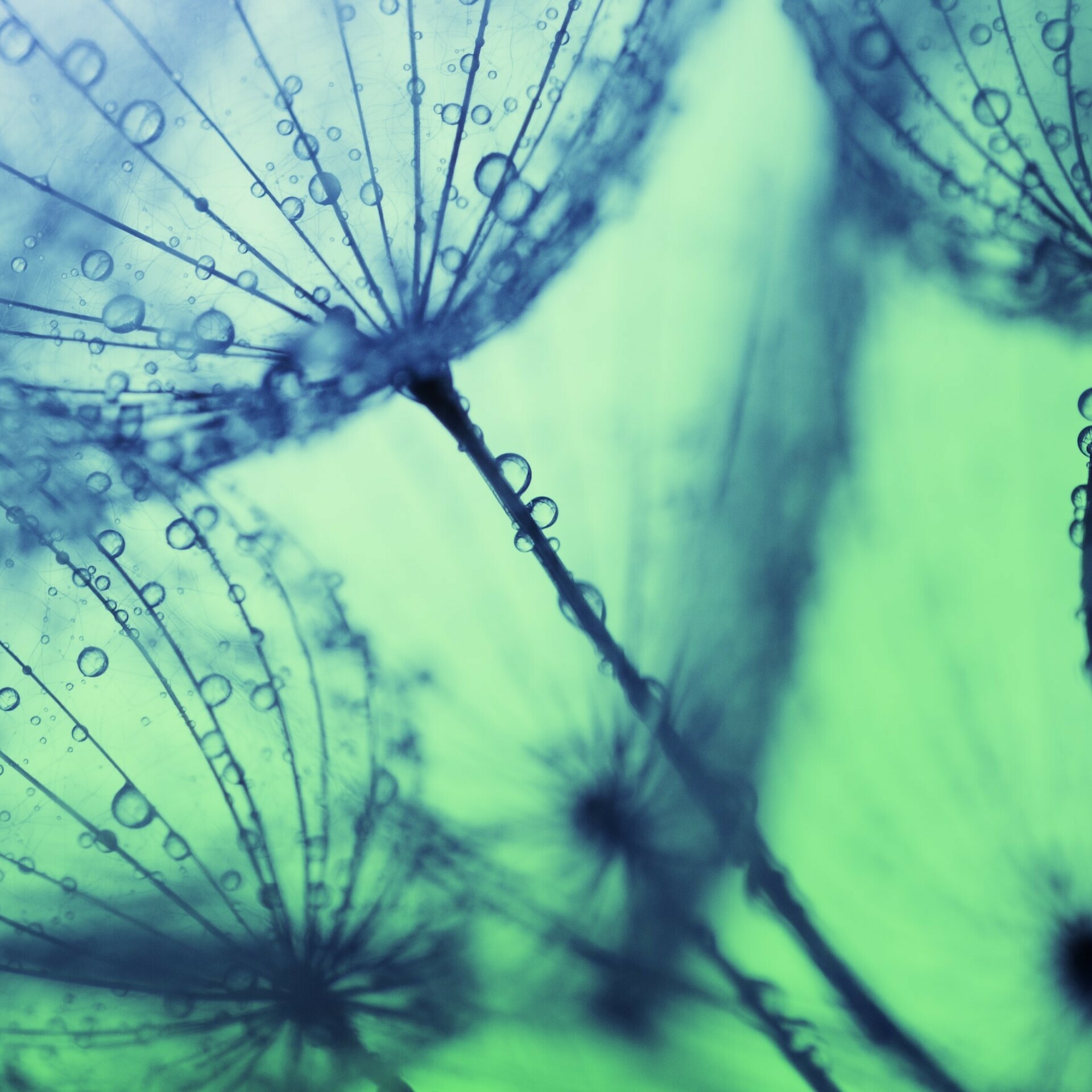
© iStock/Drbouz
According to statistics from the World Health Organization (WHO) for 2020, more than 1 in 10 babies worldwide were born too early, which places prematurity as a leading cause of death among children under 5 years of age [1]. Born after fewer than 32 weeks of gestation, very preterm infants are not adequately prepared for life outside the womb, as their organ systems are still underdeveloped.
As a result of the immaturity of their bodies, very preterm infants often develop morbidities, which predominantly impact their cardio-respiratory and central nervous systems. These morbidities affect not only their overall health, but also influence the development of further complications that can have long-term impact on their lives later on.
The fragility of Premature Babies
Morbidities have frequently been observed to manifest together in various combinations rather than in isolation. This can sometimes be explained by a shared underlying cause. Additionally, their simultaneous occurrence might influence each other’s progression.
Investigating the associations between morbidities and identifying clinical morbidity patterns can provide valuable insights into characterizing the infant’s health. Previous studies have focused mainly on individual morbidities or on isolated pairs, but the understanding of the complex overall picture remains incomplete.
Identifying Patterns: A Data-driven Approach
In collaboration with the Ludwig-Maximilians-University (LMU) hospital, researchers from the Fraunhofer Institute for Cognitive Systems IKS developed a data-driven approach based on two cohorts of very preterm infants, encompassing a total of 530 infants. Of these, 173 infants were from the AIRR study (“Attention to Infants at Respiratory Risks”, LMU hospital) and 357 infants from the NEuroSIS study (“Neonatal European Study of Inhaled Steroids”, University Hospital Tübingen). In this undertaking, the Fraunhofer IKS team went beyond pairwise correlations and analyzed morbidities collectively, aiming to provide a comprehensive understanding of the morbidity landscape. The research work focused on five main morbidities affecting the cardio-respiratory and central nervous systems:
- Bronchopulmonary dysplasia: lung disease affecting preterm babies, who often must be supplied with supplemental oxygen to help them breathe
- Pulmonary hypertension: condition characterized by high blood pressure in the blood vessels of the lungs
- Mild cardiac defects: conditions of the heart diagnosed via echocardiography
- Perinatal brain pathology: abnormalities in the brain detected using cranial ultrasound or brain MRI
- Retinopathy of prematurity: eye disease affecting vision of premature infants

Five important morbidities that occur in very premature babies.
The Fraunhofer IKS research team employed an incremental data-driven approach, starting with a close-up analysis of morbidities and gradually moving towards a broader perspective. In the first step, they looked at morbidities two by two, quantifying to what extent their occurrence is correlated. Then the perspective was broadened to encompass all morbidities simultaneously and identify co-occurrence patterns. Finally, the researchers categorized infants according to their morbidities to identify groups exhibiting similar risk profiles. In the presented approach, Fraunhofer IKS employed statistical analysis as well as unsupervised machine learning techniques.
Key Insights into Morbidity Patterns
The analysis provides a comprehensive characterization of morbidity patterns in preterm infants. Below are some of the key findings:
- The most notable link between morbidities: The highest correlation was observed between bronchopulmonary dysplasia (lung disease) and retinopathy of prematurity (eye disease). This suggests that preterm infants with lung disease are at higher risk of developing eye disease as well. This might be an indication of a shared underlying cause, such as inflammatory processes in the body or genetic factors that make babies more susceptible to both conditions.
- Lung disease – a key factor but not the whole story: Considering that bronchopulmonary dysplasia (lung disease) is often seen as a key factor in the development of other morbidities, we compared the risk of morbidities across patient groups having different severities of lung disease. This revealed that while bronchopulmonary dysplasia is important, it alone doesn’t capture the full picture of an infant’s health.
- Isolated morbidities: Around 20-25% of the patients in the cohorts exhibited isolated morbidities. However, pulmonary hypertension (cardiovascular disease affecting the lungs) was never observed to manifest alone, but always appeared alongside other morbidities.
- Morbidity load: By using unsupervised machine learning, groups of patients with similar morbidity patterns were identified. The Fraunhofer IKS’ researchers use the term “morbidity load” to refer to both the number and severity of morbidities (where no morbidity load describes the absence of morbidities, and a high morbidity load describes the presence of at least four out of five morbidities). The team identified different levels of morbidity load across groups. For instance, around a quarter of patients fell into the group of low morbidity load, meaning they have fewer and less severe health problems. Meanwhile, heart-related health issues were found to be more likely to be seen in groups with a higher morbidity load, i.e. in patients with more severe and numerous health problems.
Paper
Further results of the analysis described here can be found in the following paper:
»Delineating morbidity patterns in preterm infants at near-term age using a data-driven approach«
These findings provide an important step towards better understanding the health challenges of preterm infants. Identifying morbidity patterns and understanding the complex picture of morbidity paves the way for the development of personalized follow-up strategies that could significantly improve the health outcomes of preterm infants
[1] World Health Organisation, "Preterm birth," 2023. [Online]. Available: https://www.who.int/news-room/...