Read next
Artificial Intelligence
Can Generative AI Revolutionize Modern Healthcare?
Artificial intelligence and LLMs in particular are seen by many as a beacon of hope for the overburdened healthcare system. Above all, AI-based automation could quickly provide relief for knowledge management routine tasks. Until that happens, problems with security and safety must be solved and legal requirements fulfilled. Fraunhofer IKS research is addressing both of these issues.
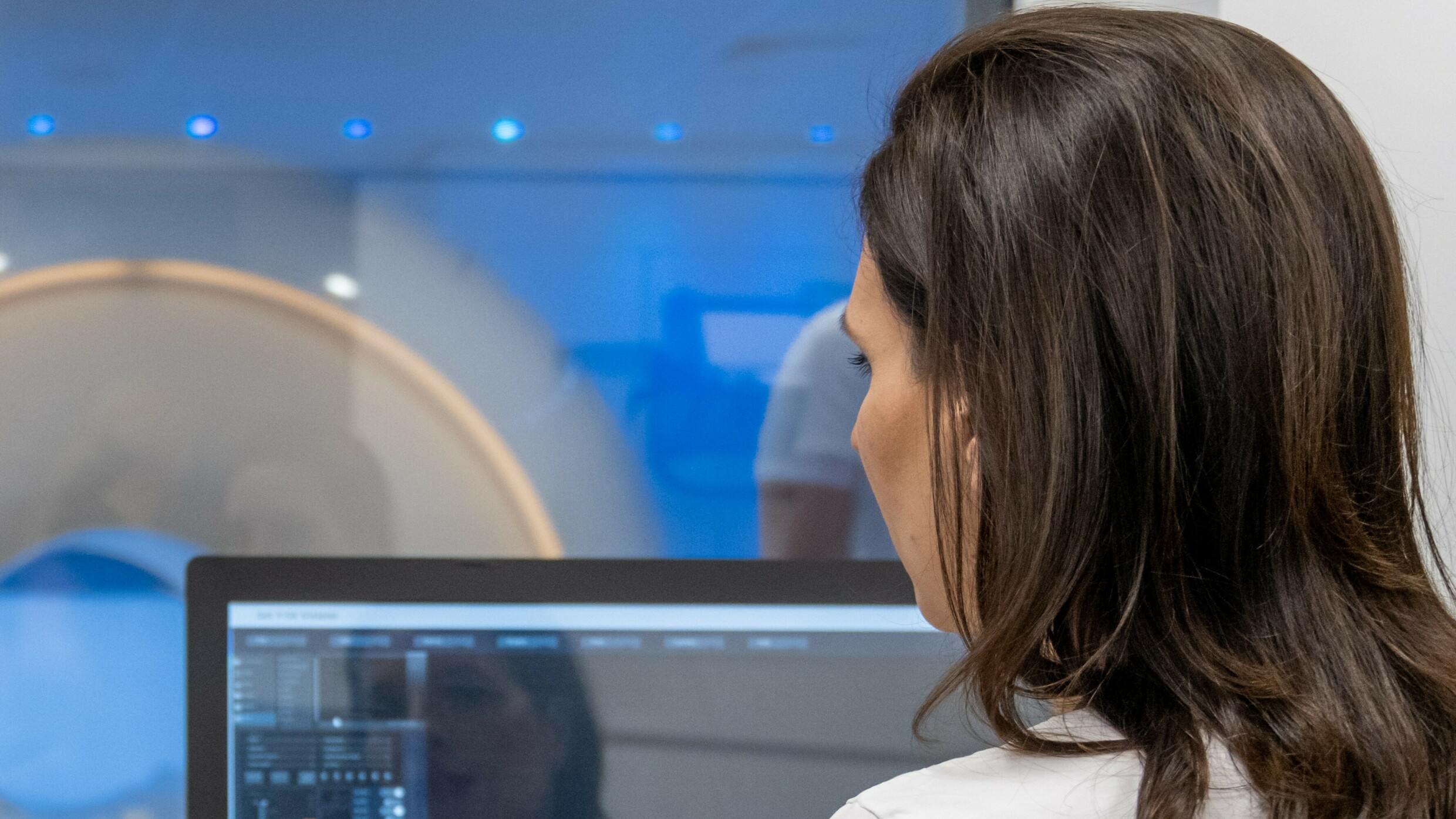
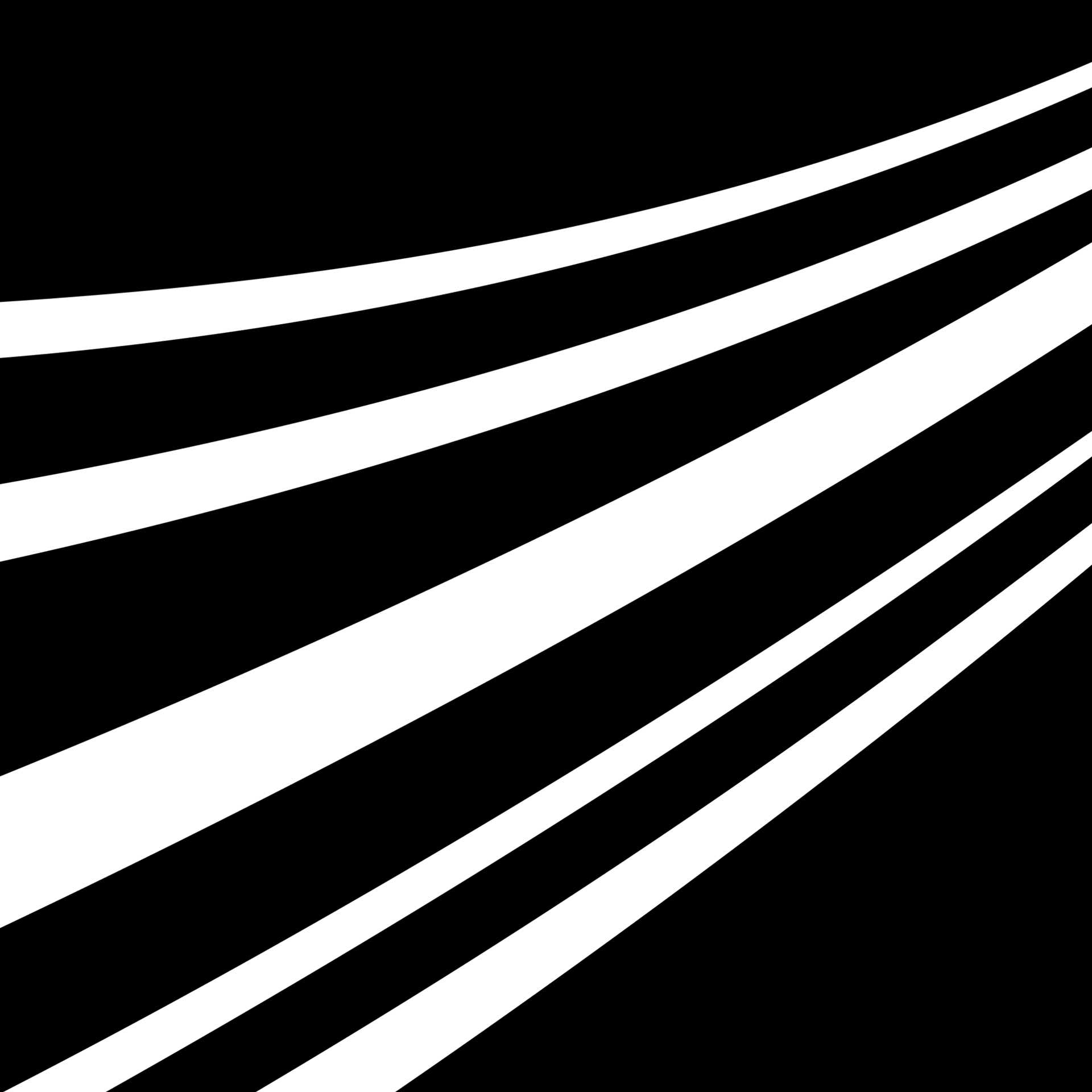
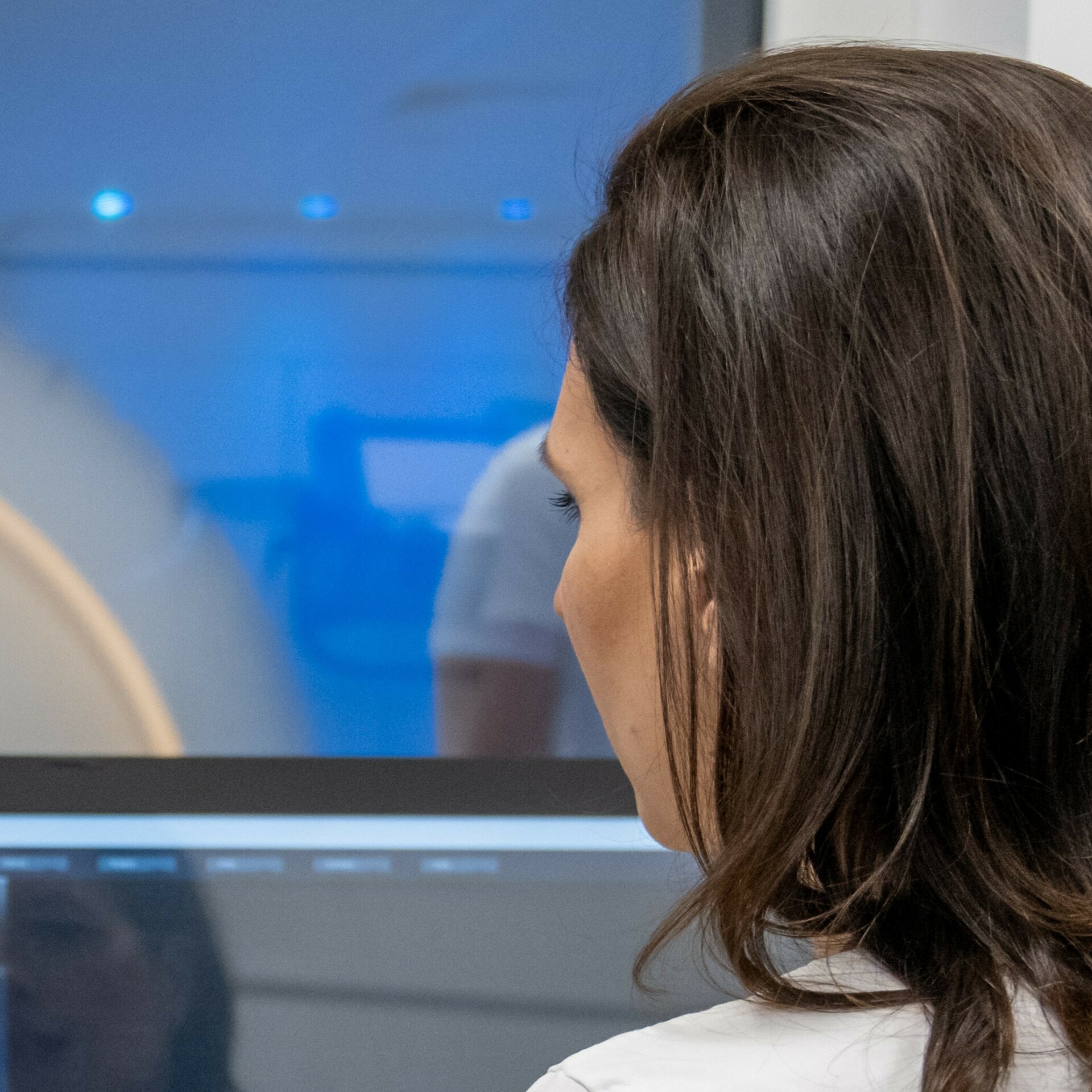
© iStock/vm
In recent years, throughout and after the Covid epidemic, it has become abundantly clear that our healthcare system is at the limit. This is not only a problem on a national level, but rather a global phenomenon. Studies have long identified the root causes, at the top: A shortage of qualified labor [1], [2]. Consequences include a higher likelihood of mistakes in medical decisions or procedures (diagnosis and treatment), less time for complex analyses, less patient interactions, high stress levels of medical staff, less access to high-quality care, etc. In Germany, the dragging digitalization further exacerbates the problem due to missing or disconnected documentation.
It is therefore no surprise that high hopes have been placed on the promises of automation through artificial intelligence (AI). While such efforts were initially focused on the most complex of medical tasks, such as diagnosis based on AI image classifications, it may be that more immediate impact lies in the automation of simpler knowledge management tasks. This is made possible by the impressive capabilities of newly emerging generative AI (genAI) models, and in particular large-language models (LLMs), to process and understand natural language.
Study: High potential for genAI in healthcare
A recent McKinsey study estimates that the unrealized potential of improvement in the global healthcare system is about one trillion dollars [3]. A significant portion of it can be addressed with genAI. How? Imagine that it was possible to fully automate only some of the following tasks [4]:
- Transcribe a verbal (live) conversation between a patient and a clinician into a structured form,
- Collect and summarize all relevant patient history from scattered documentation,
- Autogenerate a medical report based on the results of an examination,
- Autogenerate claims for a patient’s health insurance,
- Create complex schedules (e.g., in hospitals) adjusting to dynamic events,
- Collect all medical guidelines relevant for a decision, based on given symptoms,
- Interact with and advise about legal regulations relevant to a medical decision.
All the above examples and more can potentially be executed in seconds by a LLM, in combination with a customized logic and data connectors. Automating such time-consuming routine tasks saves resources for the staff to focus on complex tasks, or simply get back that “human touch” patients are missing. Domains such as marketing and customer service are already demonstrating how productivity can be gained by offloading very similar functionalities to chatbots [5], [6].
Healthcare businesses have started to recognize this potential. Mass General Brigham, a provider of diagnostic healthcare products in the US, has pivoted to generative AI to identify patients with similar profiles [6]. Diagnostic information, genetic data, and medical records are searched for correlations using LLMs, a task that previously could only be done semi-automated at a much slower pace. Also Siemens Healthineers sees genAI as the next leap forward in radiology [7]. An appealing possibility is that data, which is connected and structured by LLMs first, can be leveraged to improve radiological scans and help in the diagnosis. For example, an AI agent could find the optimal parameters for a CT scan based on a patient’s history, reducing overhead for technical assistants.
Medical practitioners can further hope to enjoy the automated generation of medical reports (including dispatch letters, prescriptions for pharmacies, insurances, etc.) through genAI-apps, a concept occasionally coined “Arztbriefgenerator” in German [8], [4]. Ultimately, the entire workflow in medical standard routines might benefit from LLM-support (see Figure 1). Such promises have also influenced the political agenda: The German academy of sciences and advisory board to the chancellor recommends to strategically support the national AI ecosystem [9], acknowledging the recent global impact of generative models.
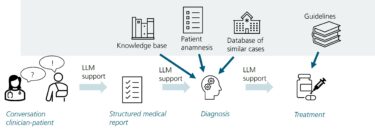
© Fraunhofer IKS
Figure 1: Potentially, LLMs can support the entire process workflow, from the initial patient conversation to treatment decisions.
Overcoming road blockers
A widespread adoption of genAI, however, needs to face two main challenges: security
and safety. Security issues not only include model manipulations or infrastructure attacks, but also situations where sensitive medical data is fed to AI services outside of the established data sphere. AI Cloud providers like OpenAI counter privacy concerns by offering enterprise accounts that promise GDPR compliance, and a full data ownership for the customer (e.g., data sent as prompts will not be used for training other models) [10]. Customers who are not fully convinced can alternatively resort to local, on-premise solutions with open-source models [11]. While those still require significant compute and memory resources for now, it seems only a matter of time until the first competitive LLM will be deployed on an off-the-shelf mobile computer.
A different type of challenge occurs in the safety context. As LLMs are statistical text generators, the correctness of the output cannot be guaranteed in all cases. Situations where the model generates plausible, but incorrect content, are well known as “hallucinations” or “dreaming”. Even for routine tasks like auto-filling a medical form, it is possible that an incorrect piece of information, for example a patient’s fictitious pre-existing condition, is generated accidentally, which may endanger later treatment options. To avoid such scenarios, one of the main research questions in the field of generative AI is therefore, how to improve the alignment of LLM results with user safety. This is where the work at the Fraunhofer Institute for Cognitive Systems IKS starts.
Popular pretrained LLMs have a built-in filter for hate speech, racism, or dangerous requests. Those are typically added after the self-supervised training process through supervised fine-tuning with specific examples, and reinforcement learning from human feedback (RLHF). To incorporate application-specific safety (for example, related to a set of treatment options in medicine), this approach is resource-intensive and requires sufficient high-quality data samples. Instead, the Fraunhofer IKS follows a different approach using safety-aware LLM agents [12].
A LLM agent is a wrapper program with access to a pretrained LLM, as well as other tools and domain-specific data. Following a customized logic, it can orchestrate such resources to solve a request in multiple steps. For example, after receiving a text prompt, the agent can first assess if additional information is required, and if so, extract it from available documents through retrieval-augmented generation (RAG). In a second step, the agent can, for instance, decide that this question should be answered by a simple lookup instead of using a LLM. Other subtasks, like text summarization, might be in contrast well-suited for a LLM call.
At Fraunhofer IKS, researchers develop agent workflows that either prevent or mitigate unsafe decisions that are possibly produced by a LLM. To that end, the agent is equipped with customized safety concepts, as well as medical standards and guidelines, such that a request can be answered via an optimal use of the most appropriate tool. For the near-term future a human review is obviously indispensable. . To facilitate it, generated results need to be readily verifiable, for example by listing the text sources that were relevant in the agent’s decision path.
Will the notoriously overstrained German healthcare system and its patients soon benefit from such productivity gains? Chances are, that companies and practitioners first have to navigate a legal labyrinth: With the recent EU AI Act and the European Medical Devices Regulation (MDR EU), medical AI products require a rigorous certification [13], [14]. To facilitate this process, Fraunhofer IKS develops guidelines and tools that help clients assess, what risks are associated with their product and which steps need to be taken towards certification – making use of LLM-powered agents as part of its solution.
References
[1] Ipsos, “Baustelle Gesundheitssystem: Fachkräftemangel mit Abstand größtes Problem,” 2021, [Online]. Available: https://www.ipsos.com/de-de/ba...
[2] Deutschlandfunk, “Die Überlastung hat System.” [Online]. Available: https://www.deutschlandfunk.de...
[3] McKinsey, “Tackling healthcare’s biggest burdens with generative AI.” [Online]. Available: https://www.mckinsey.com/indus...
[4] D. Antweiler, K. Beckh, N. Chakraborty, S. Giesselbach, K. Klug, and S. Rüping, “Natural Language Processing in der Medizin. Whitepaper,” Fraunhofer IAIS, 2023, doi: 10.24406/publica-1278.
[5] McKinsey & Company, “The economic potential of generative AI,” McKinsey Co. Reports, no. June, pp. 1–65, 2023, [Online]. Available: https://www.mckinsey.de/~/medi... and middle east/deutschland/news/presse/2023/2023-06-14 mgi genai report 23/the-economic-potential-of-generative-ai-the-next-productivity-frontier-vf.pdf
[6] WSJ, “How Did Companies Use Generative AI in 2023? Here’s a Look at Five Early Adopters,” 2023. [Online]. Available: https://www.wsj.com/articles/h...
[7] Elektroniknet.de, “Generative KI ist der nächste große Schritt in der Radiologie.” [Online]. Available: https://www.elektroniknet.de/m...
[8] Aerzteblatt, “KI-betriebener Arztbriefgenerator: In Sekunden zum Entlassbrief,” 2023, [Online]. Available: https://www.aerzteblatt.de/nac...
[9] acatech, “Vom Spitzentalent zum Schlüsselspieler: Strategie für die erfolgreiche Nutzung und Entwicklung generativer KI in Deutschland,” 2024, [Online]. Available: https://www.acatech.de/allgeme...
[10] “Enterprise privacy at OpenAI.” [Online]. Available: https://openai.com/enterprise-...
[11] Meta, “LLama 3.1.” [Online]. Available: https://ai.meta.com/blog/meta-...
[12] F. Geissler, K. Roscher, and M. Trapp, “Concept-Guided LLM Agents for Human-AI Safety Codesign,” Proc. AAAI Symp. Ser., vol. 3, no. 1, pp. 100–104, 2024, doi: 10.1609/aaaiss.v3i1.31188.
[13] L. Heidemann et al., “The European Artificial Intelligence Act: Overview and Recommendations for Compliance,” pp. 1–25, 2024.
[14] A. Zamanian, G. Lancho, and E. Pachl, “KI-Entwicklung - von der Vorschrift zum Computercode,” mt medizintechnik, vol. 2, 2024.