Read next
AI in medicine
Getting to grips with uncertainty
In the near future, medical engineering will be shaped by artificial intelligence and machine learning. However, the complex processes involved in machine learning create a problem for researchers — it is costly and challenging to eliminate errors before autonomous systems are put into use, and to reliably detect errors during operation.
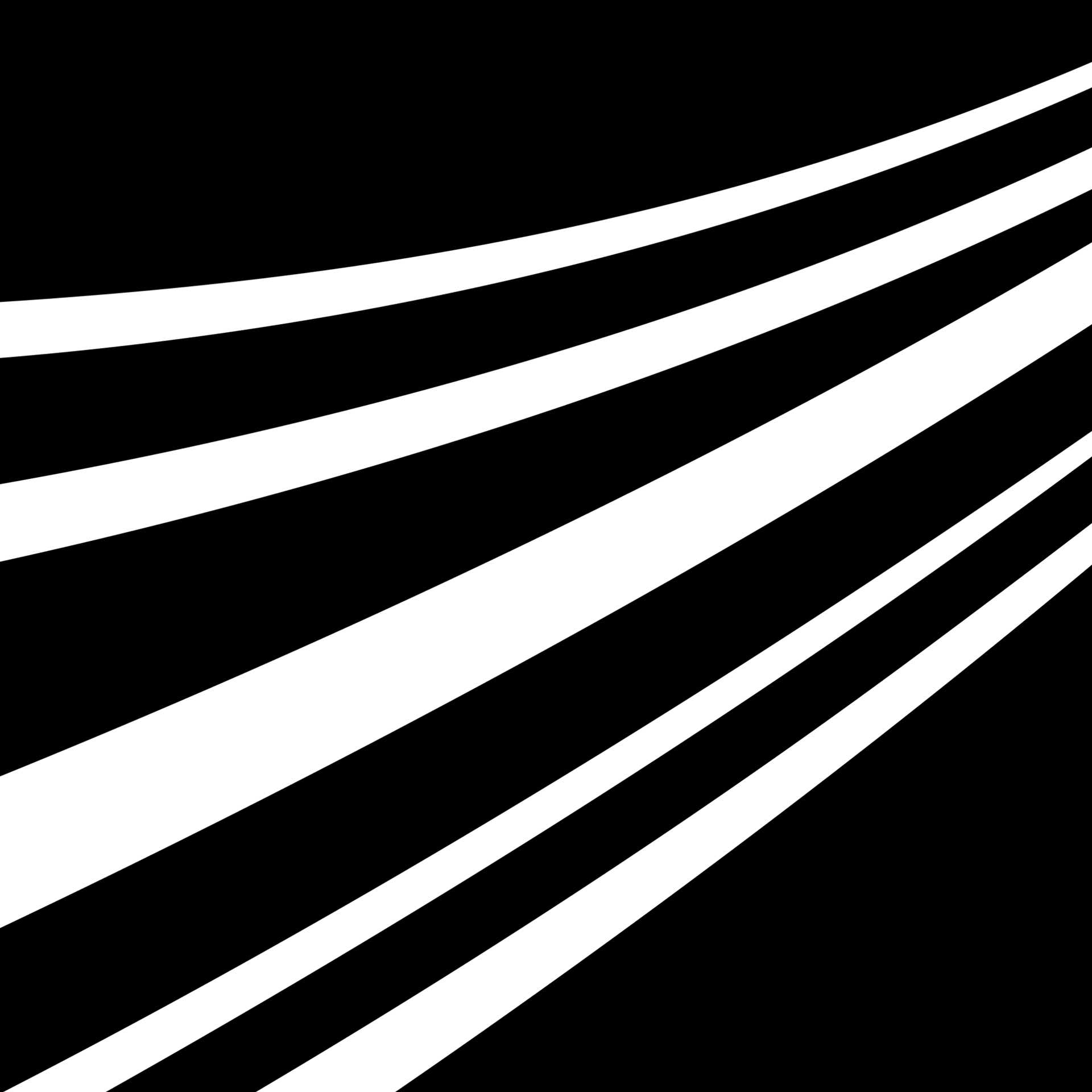
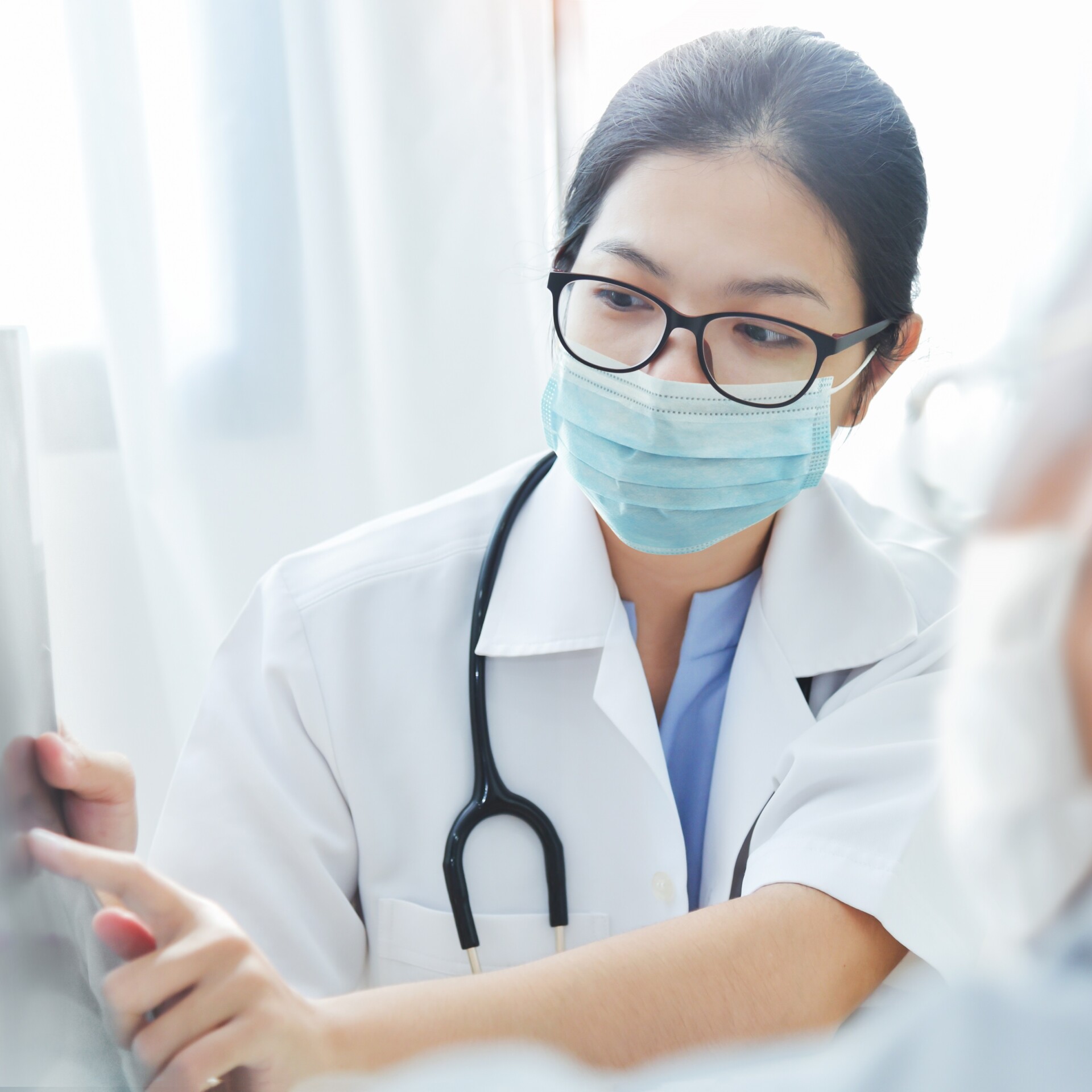
© iStock.com/eggeeggjiew
Artificial intelligence (AI) algorithms can be trained, evaluated and possibly even autonomously updated on a continuous basis without any human input. This is why they are often called black box technology. However, although AI is flexible and theoretically capable of outperforming humans when it comes to statistical methods, there are many elements of AI technology that are often not interpretable — especially the logic behind its decision-making processes. For example, if a dermatologist carries out a personalized assessment and comes to a conclusion, they can explain that conclusion based on the clinical evidence available. In contrast, decisions made by AI technology cannot currently be interpreted in this way.
This is a severe limitation, as it affects whether and under which conditions society and supervisory authorities will accept AI in daily medical practice. In other words, if artificial intelligence is going to be used in such safety-critical applications, it needs to be verifiable. That means we must be able to assess the degree of uncertainty in the results the technology produces. These uncertainty values provide information on how reliable the AI’s current predictions are. They also serve as influencing factors that other system components can use to evaluate the system’s current certainty level and corroborate the AI’s decisions.
Determining this uncertainty level is a significant challenge. Many machine learning (ML) models, especially neural networks, can be pictured as very complex nested functions with various applications; for example, in the case of classification, they can be used for assigning images to categories. When training this kind of model, to put it simply, its parameters are adjusted so that the confidence values — which measure the certainty of a statement — of the correct classes in each case are increased (as opposed to the incorrect ones).
Acceptance as a decisive criterion
What does this mean for medicine in general, and for dermatology in particular? In dermatology, the standard procedure for reaching a diagnosis is to take a proper medical history and then conduct an examination in a well-lit environment to assess textures and note specific symptoms of certain lesions; this is supplemented with additional examinations and/or image analysis or a biopsy.
In addition, it is widely known that some diagnoses are clinical, while others are based solely on histological findings or on a combination of both. This holistic approach cannot be fully replaced by computer programs — this is seen as one of the principal barriers to AI adoption. In addition, if an AI system were developed, many patients would still just want to see a doctor who would advocate for them so that they could tackle their treatment together, rather than settling for using an isolated computer-assisted program.
Fraunhofer IKS makes AI more self-critical
But let’s get back to the technology: The fact that many established machine learning methods use specific single values for parameters instead of their distributions can also be an issue. Using a single, fixed example that inadequately depicts real-world patterns and connections is less than ideal. It increases the risk of the models being misleading in awkward cases, and highlights a further cause of unreliable confidence values — models are trained using datasets that do not represent all relevant factors (such as skin color, differences in lighting, focus, etc.), which can lead to incorrect results.
To avoid these errors, the Fraunhofer Institute for Cognitive Systems IKS is developing new methods that allow higher uncertainty values to be assigned in circumstances that deviate significantly from the patterns in the training dataset. This allows the model to better indicate when its predictions are uncertain. One area of focus for the researchers is reliable predictive modeling for healthcare applications — a multi-disciplinary AI solution that combines machine learning, causal inference and optimization in the healthcare context. In these applied projects, Fraunhofer IKS is developing new ML and causal inference methods and transferring them from theory to practice, so that the future occurrence of adverse incidents, complications and results can be predicted using prior information on disease progression in patients.
Find out more!
If you would like to find out more about the research Fraunhofer IKS is undertaking in the field of AI in medicine, please contact our Business Development team to arrange a one-to-one meeting.
These new methods will help answer questions such as
- whether a patient will develop a certain illness due to their previous medical history.
They will also help identify categories of patients that are subject to increased risks; for example, they can determine the complications risks that certain treatments pose for patients. The direct and indirect effects of treatment decisions on patient outcomes also need to be assessed, i.e. by means of a mediation analysis. This will ultimately also involve developing techniques to evaluate the effects of alternative treatment decisions on patient outcomes, i.e. counterfactual analysis.
These assessments can answer questions such as
- whether a patient would have experienced a different outcome if physicians had given them a different treatment in the past.
In all of these areas, researchers at Fraunhofer IKS are particularly
interested in quantifying the degree of uncertainty involved in of
observations and decisions made by both humans and AI, designing robust models for cases of missing-not-at-random data, and developing reliable validation metrics that are aligned with clinical needs.
This article was first published in November 2021 in the journal Kompass Dermatologie.
Source: https://www.karger.com/Article...